Toward self-organizing low-dimensional organic–inorganic hybrid perovskites: Machine learning-driven co-navigation of chemical and compositional spaces
MRS BULLETIN(2023)
摘要
Low-dimensional hybrid perovskites combine the richness of physical functionalities of inorganic materials and complexity and stimulus responsiveness of organic molecules in a single bulk dynamic material. The unique aspect of these materials is the thermodynamic (meta) stability, allowing for self-organized formation of complex large-period structures. Combined with the ease of fabrication, these materials not only have extensively demonstrated state-of-the-art high-performance optoelectronics, but also offer the pathway toward versatile applications, including sensors, electronic, and neuromorphic devices as well as their cost-effective mass production. However, discovery and optimization of this material require joint optimization of the composition of the inorganic components and selection of the molecular moieties, to harness the phase formation and self-assembly processes on the material level, and extend it to micro- and macroscale functional devices. Here, we discuss the potential of machine learning-driven automated experiments to accelerate the discovery of these materials, optimize the processing pathways, and transition from the lab-level to the product-level manufacturing. Graphical abstract
更多查看译文
关键词
Low-dimensional perovskite,Automation,High throughput,Synthesis,Machine learning
AI 理解论文
溯源树
样例
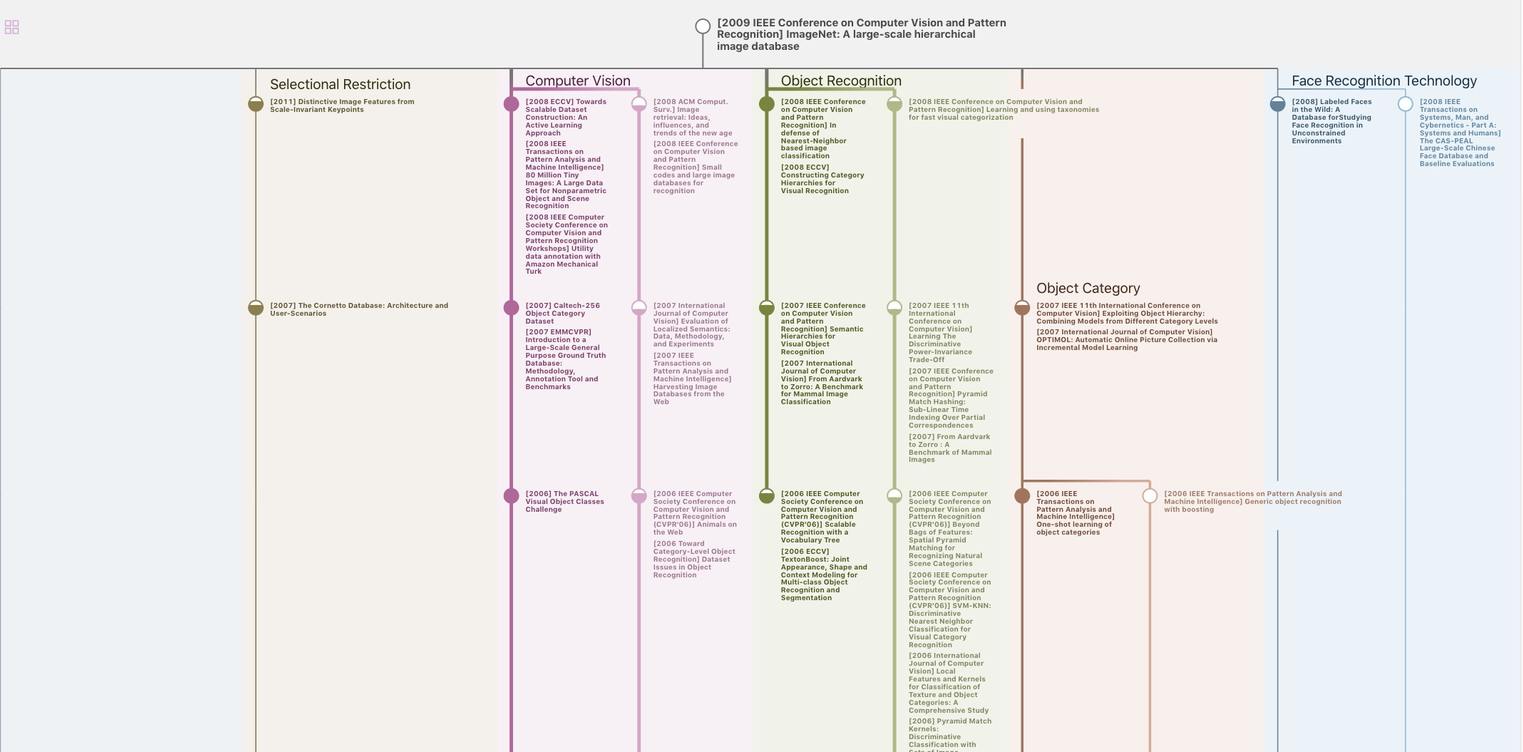
生成溯源树,研究论文发展脉络
Chat Paper
正在生成论文摘要