An entropy and copula-based framework for streamflow prediction and spatio-temporal identification of drought
STOCHASTIC ENVIRONMENTAL RESEARCH AND RISK ASSESSMENT(2023)
摘要
Reliable and easy-to-implement predictions of hydrometeorological variables are important for policymaking and public security. In this study, we developed a probabilistic framework for the description of hydrometeorological high-dimensional dependence and prediction by first-time coupling the principle of maximum entropy (POME) with C-vine copulas (PC). Two case studies with different emphases were investigated to evaluate the application of the PC framework. In the first case, we tested the PC framework based on a one-month-ahead streamflow forecast at the outlet station of the Jinsha River Basin. Results indicated that: (1) the marginal probability distributions or margins derived from optimal-moment-based POME best represented the current state of knowledge compared with those from traditional parametric distributions, and (2) the PC framework produced more skillful forecasts than did the traditional parametric C-vine (TC) and three data-driven models. The second case verified the performance of the PC framework in nationwide summer drought identification. Results of visual comparison of two typical historical summer drought events indicated that the PC framework captured the spatio-temporal characteristics of droughts. The PC framework combines the respective advantages of POME and C-vine copulas, ensuring its potential in higher-dimensional hydrometeorological modeling and flexibility in extending to other fields.
更多查看译文
关键词
Principle of maximum entropy,Vine copula,Hydrometeorological variable modeling,Drought identification,Moment constraints,High-dimensional hydrometeorological dependence,Probabilistic framework,Entropy-copula coupling
AI 理解论文
溯源树
样例
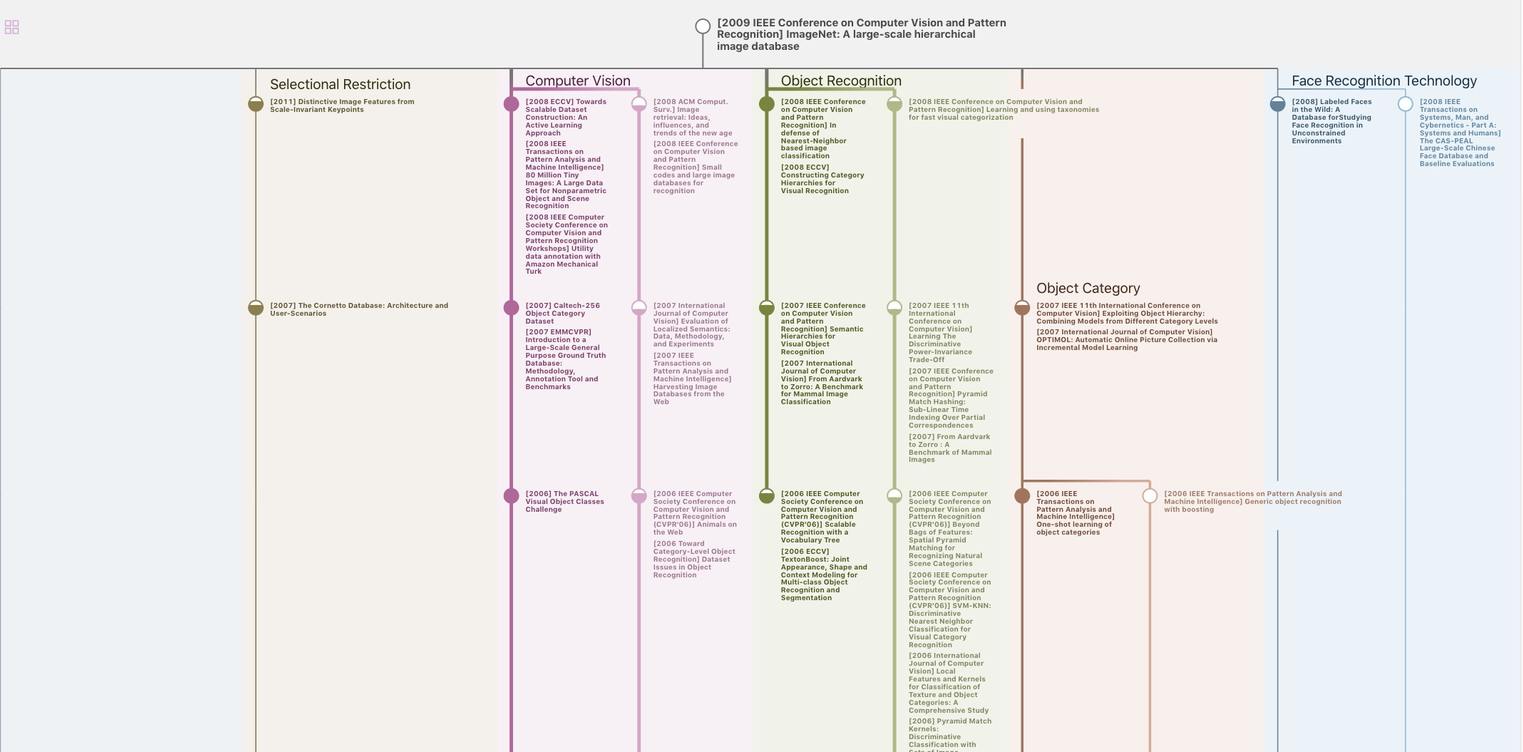
生成溯源树,研究论文发展脉络
Chat Paper
正在生成论文摘要