DRRU-Net: DCT-Coefficient-Learning RRU-Net for Detecting an Image-Splicing Forgery
APPLIED SCIENCES-BASEL(2023)
摘要
In this paper, we propose a lightweight deep learning network (DRRU-Net) for image-splicing forgery detection. DRRU-Net is an architecture that combines RRU-Net for learning the visual content of images and image acquisition artifacts, and a JPEG artifact learning module for learning compression artifacts in the discrete cosine transform (DCT) domain. The backbone model of a network based on pre-training, such as CAT-Net, a representative network for image forgery detection, has a relatively large number of parameters, resulting in overfitting in a small dataset, which hinders generalization performance. Therefore, in this paper, the learning module is designed to learn the characteristics according to the DCT domain in real time without pre-training. In the experiments, the proposed network architecture and training method of DRRU-Net show that the network parameters are smaller than CAT-Net, the forgery detection performance is better than that of RRU-Net, and the generalization performance for various datasets can be improved.
更多查看译文
关键词
image-splicing forgery,discrete cosine transform,DCT,RRU-Net,DRRU-Net
AI 理解论文
溯源树
样例
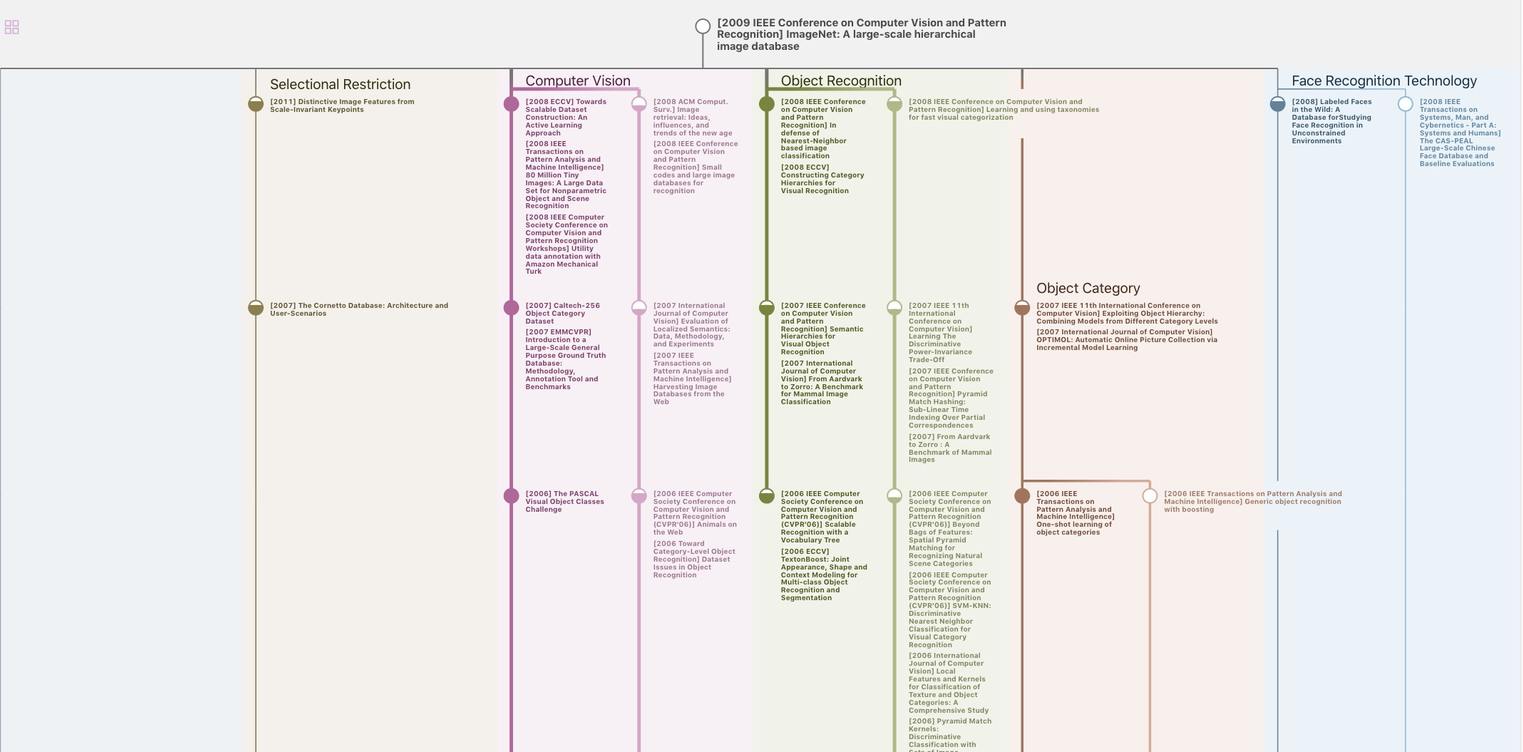
生成溯源树,研究论文发展脉络
Chat Paper
正在生成论文摘要