Bearing Fault Diagnosis Based on Wide Deep Convolutional Neural Network and Long Short Term Memory
TEHNICKI VJESNIK-TECHNICAL GAZETTE(2023)
摘要
Mechanical fault can cause economic loss of different degrees, even casualties. Timely fault diagnosis is an essential condition for ensuring safe production in modern industry. With the growth of intelligent manufacturing, more and more attention is paid to fault diagnosis methods that are based on deep learning. However, the diagnostic accuracy of existing diagnostic methods has still to be improved. Therefore, a fault diagnosis method called WDCNN-LSTM is proposed by combining Wide Firstlayer Deep Convolutional Neural Network with Long and Short Term Memory. Feature information is extracted adaptively from one-dimensional original vibration signals by Convolutional Neural Network. The extracted features are further extracted by Long and Short Term Memory, so that the fault feature information can be fully obtained. Experiments are performed on CWRU datasets to verify our proposed method. By analyzing the experimental results, we find that the average accuracy of the proposed
更多查看译文
关键词
bearing fault diagnosis,deep learning,mechanical equipment,vibration signal
AI 理解论文
溯源树
样例
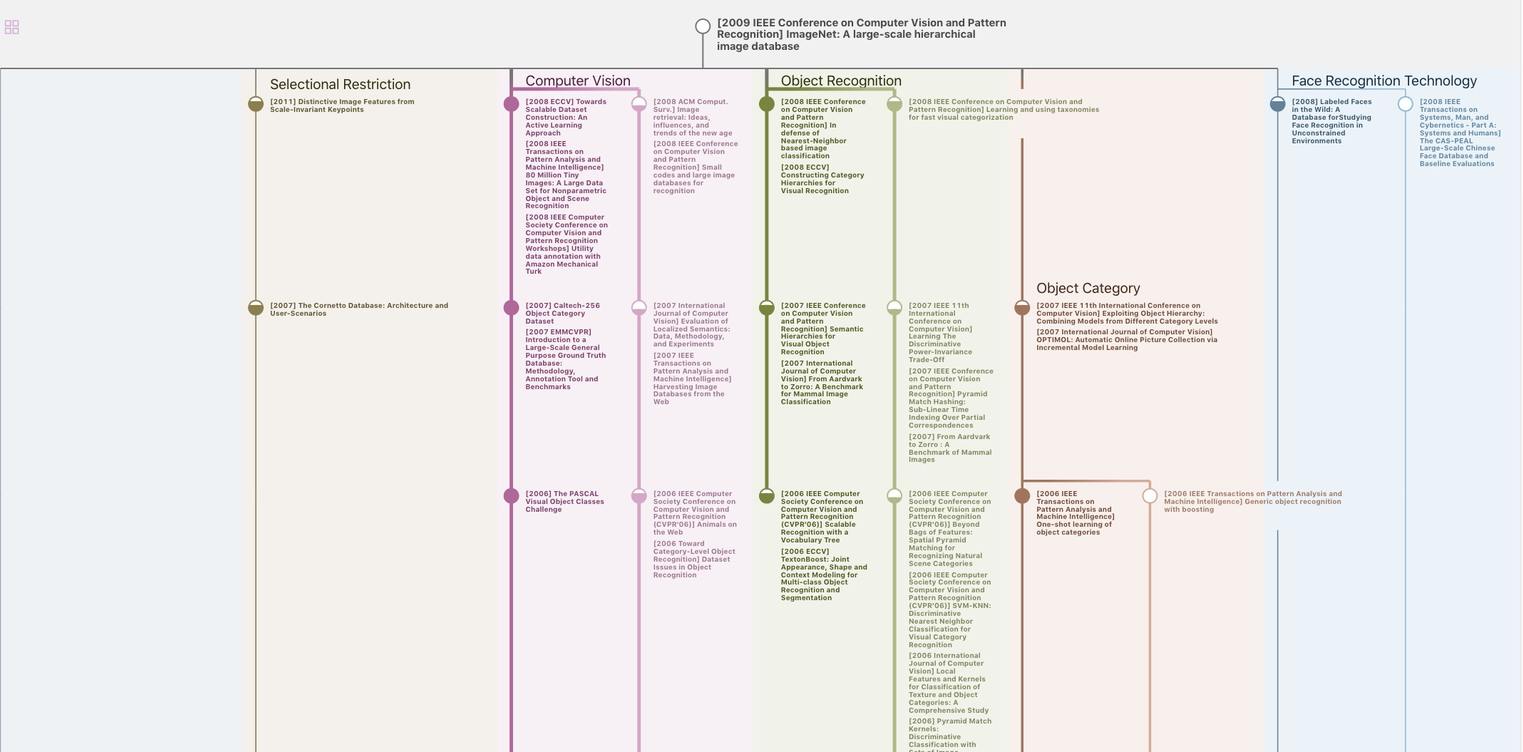
生成溯源树,研究论文发展脉络
Chat Paper
正在生成论文摘要