Analyzing Geographic Questions Using Embedding-based Topic Modeling
ISPRS INTERNATIONAL JOURNAL OF GEO-INFORMATION(2023)
摘要
Recently, open-domain question-answering systems have achieved tremendous progress because of developments in large language models (LLMs), and have successfully been applied to question-answering (QA) systems, or Chatbots. However, there has been little progress in open-domain question answering in the geographic domain. Existing open-domain question-answering research in the geographic domain relies heavily on rule-based semantic parsing approaches using few data. To develop intelligent GeoQA agents, it is crucial to build QA systems upon datasets that reflect the real users' needs regarding the geographic domain. Existing studies have analyzed geographic questions using the geographic question corpora Microsoft MAchine Reading Comprehension (MS MARCO), comprising real-world user queries from Bing in terms of structural similarity, which does not discover the users' interests. Therefore, we aimed to analyze location-related questions in MS MARCO based on semantic similarity, group similar questions into a cluster, and utilize the results to discover the users' interests in the geographic domain. Using a sentence-embedding-based topic modeling approach to cluster semantically similar questions, we successfully obtained topic models that could gather semantically similar documents into a single cluster. Furthermore, we successfully discovered latent topics within a large collection of questions to guide practical GeoQA systems on relevant questions.
更多查看译文
关键词
GeoQA,GeoQA dataset,KBQA,semantic parsing,topic modeling
AI 理解论文
溯源树
样例
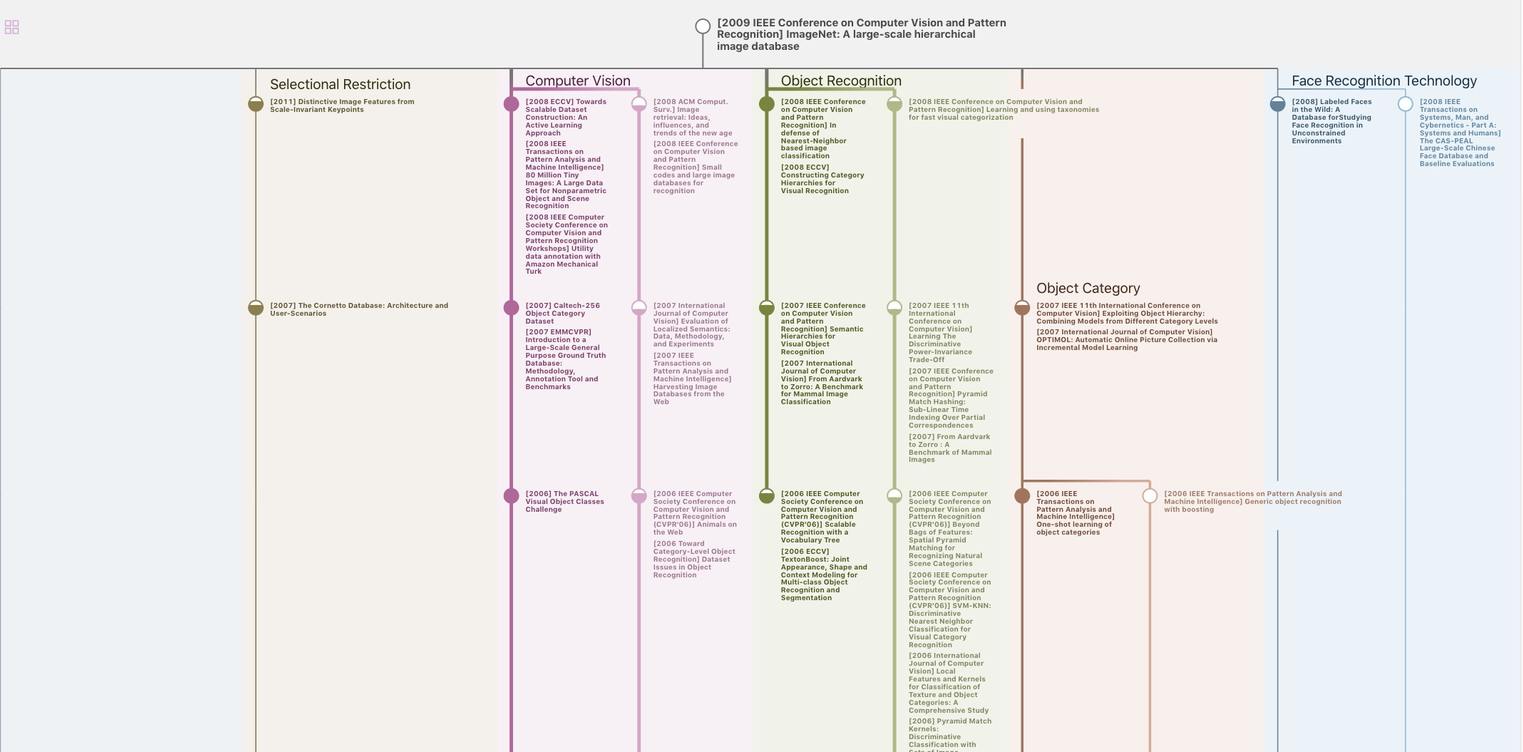
生成溯源树,研究论文发展脉络
Chat Paper
正在生成论文摘要