Predicting lattice thermal conductivity from fundamental material properties using machine learning techniques
JOURNAL OF MATERIALS CHEMISTRY A(2023)
摘要
High-throughput screening and material informatics have shown a great power in the discovery of novel materials, including batteries, high entropy alloys, and photocatalysts. However, the lattice thermal conductivity (kappa) oriented high-throughput screening of advanced thermal materials is still limited to the intensive use of first principles calculations, which is inapplicable to fast, robust, and large-scale material screening due to the unbearable computational cost demanding. In this study, 15 machine learning algorithms are utilized for fast and accurate kappa prediction from basic physical and chemical properties of materials. The well-trained models successfully capture the inherent correlation between these fundamental material properties and kappa for different types of materials. Moreover, deep learning combined with a semi-supervised technique shows the capability of accurately predicting diverse kappa values spanning 4 orders of magnitude, especially the power of extrapolative prediction on 3716 new materials. The developed models provide a powerful tool for large-scale advanced thermal functional materials screening with targeted thermal transport properties.
更多查看译文
关键词
thermal conductivity,fundamental material properties,machine learning,lattice
AI 理解论文
溯源树
样例
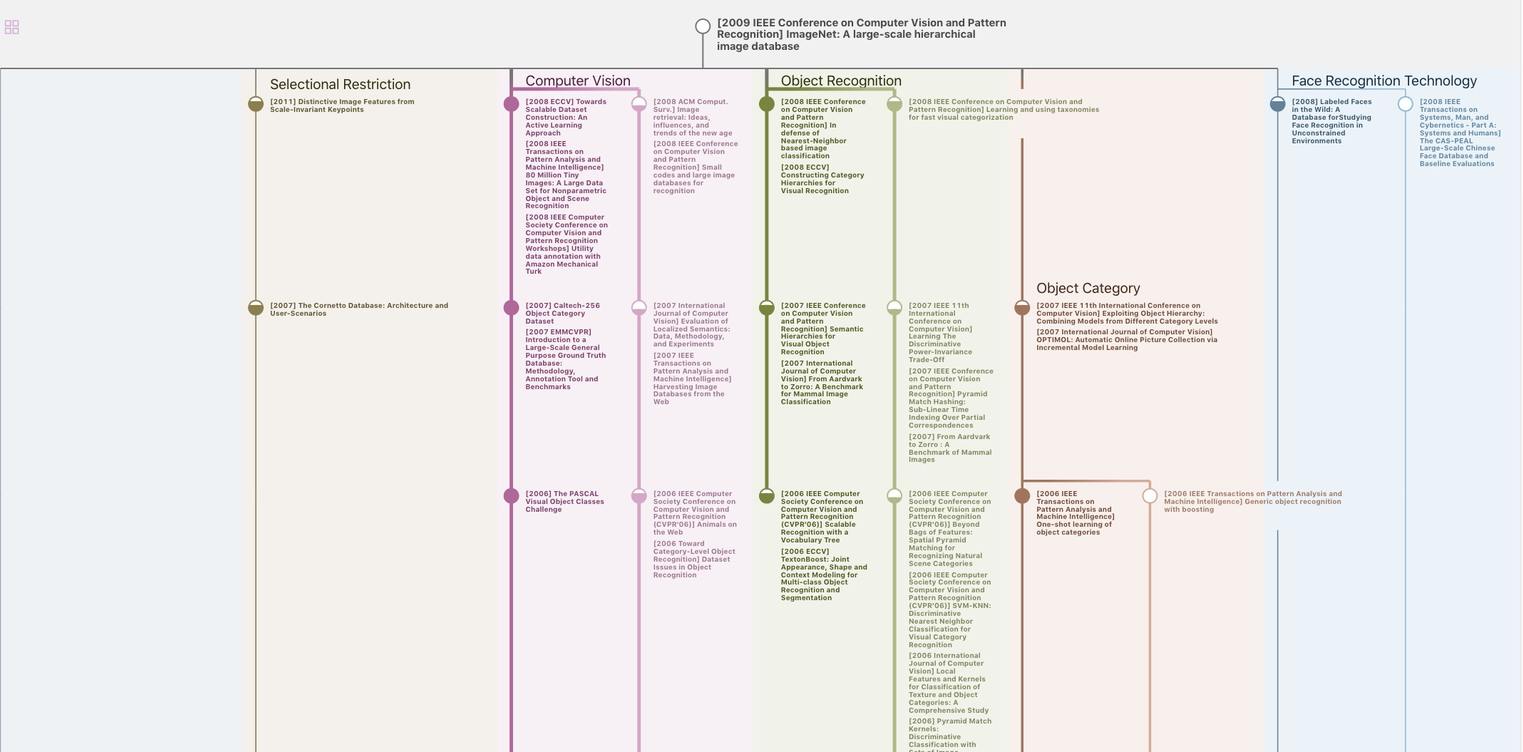
生成溯源树,研究论文发展脉络
Chat Paper
正在生成论文摘要