A Multi-Granularity Word Fusion Method for Chinese NER
APPLIED SCIENCES-BASEL(2023)
摘要
Named entity recognition (NER) plays a crucial role in many downstream natural language processing (NLP) tasks. It is challenging for Chinese NER because of certain features of Chinese. Recently, large-scaled pre-training language models have been used in Chinese NER. However, since some of the pre-training language models do not use word information or just employ word information of single granularity, the semantic information in sentences could not be fully captured, which affects these models' performance. To fully take advantage of word information and obtain richer semantic information, we propose a multi-granularity word fusion method for Chinese NER. We introduce multi-granularity word information into our model. To make full use of the information, we classify the information into three kinds: strong information, moderate information, and weak information. These kinds of information are encoded by encoders and then integrated with each other through the strong-weak feedback attention mechanism. Specifically, we apply two separate attention networks to word embeddings and N-grams embeddings. Then, the outputs are fused into another attention. In these three attentions, character embeddings are used to be the query of attentions. We call the results the multi-granularity word information. To combine character information and multi-granularity word information, we introduce two fusion strategies for better performance. The process makes our model obtain rich semantic information and reduces word segmentation errors and noise in an explicit way. We design experiments to get our model's best performance by comparing some components. Ablation study is used to verify the effectiveness of each module. The final experiments are conducted on four Chinese NER benchmark datasets and the F1 scores are 81.51% for Ontonotes4.0, 95.47% for MSRA, 95.87% for Resume, and 69.41% for Weibo. The best improvement achieved by the proposed method is 1.37%. Experimental results show that our method outperforms most baselines and achieves the state-of-the-art method in performance.
更多查看译文
关键词
Chinese NER,character word fusion,N-grams,BERT-based model,attention mechanism
AI 理解论文
溯源树
样例
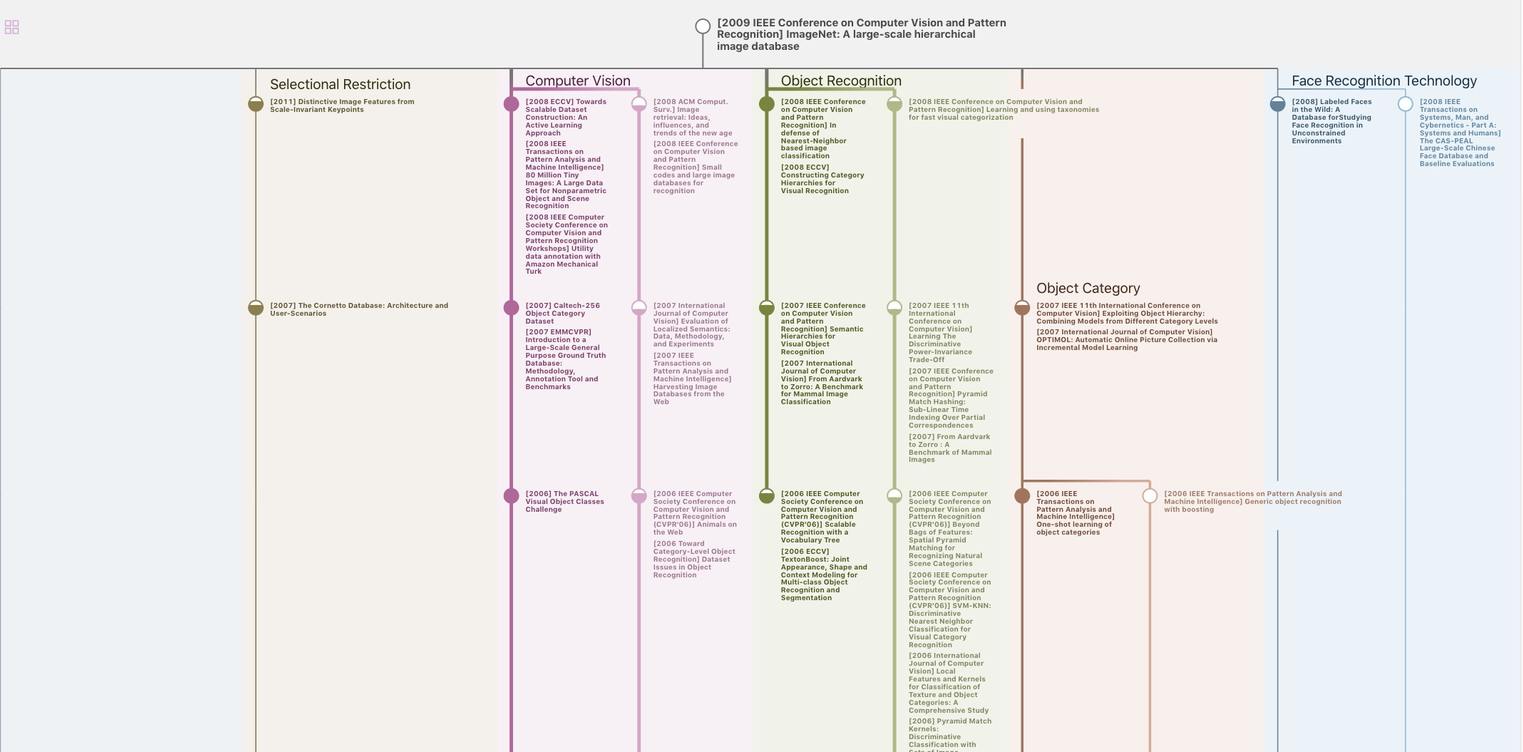
生成溯源树,研究论文发展脉络
Chat Paper
正在生成论文摘要