Reshaping the material research paradigm of electrochemical energy storage and conversion by machine learning
ECOMAT(2023)
摘要
For a "Carbon Neutrality " society, electrochemical energy storage and conversion (EESC) devices are urgently needed to facilitate the smooth utilization of renewable and sustainable energy where the electrode materials and catalysts play a decisive role. However, the efficiency of the current trial-and-error research paradigm largely lags behind the imminent demands of EESC requiring increasingly improved performance. The emerged machine learning (ML), a subfield of artificial intelligence, is capable of evaluating and analyzing big data for hidden rules. In this regard, the relationships between the structure and performance of the key materials can be more efficiently revealed, which fundamentally revolutionizes the material research manner of the current EESC devices. In this review, the typical ML algorithms utilized in EESC development are first introduced. Then, focused attention has been paid to multiple aspects of applying ML to reshape the materials research for EESC. In addition to highlighting the emerging prospect, the challenges which are still hindering the further development of this emerging field are also discussed.
更多查看译文
关键词
algorithm,electrochemical energy storage and conversion,machine learning,material research paradigm
AI 理解论文
溯源树
样例
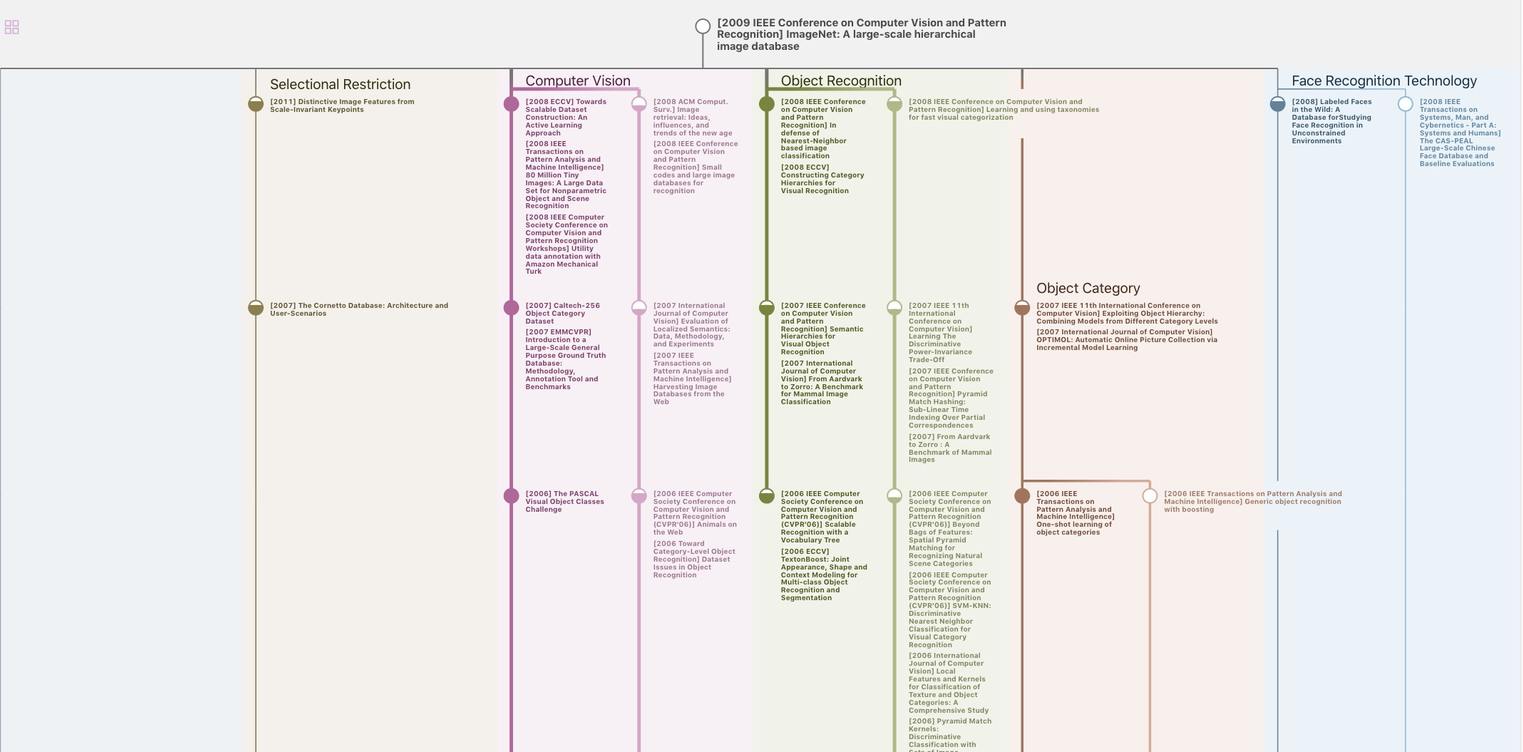
生成溯源树,研究论文发展脉络
Chat Paper
正在生成论文摘要