A New Random Forest and Support Vector Machine-based Intrusion Detection Model in Networks
NATIONAL ACADEMY SCIENCE LETTERS-INDIA(2023)
摘要
There exist many intrusion detection systems (IDSs) to provide privacy and security to user data in networks. However, these models are prone to generate high false alarms due to large amounts of noisy data and large feature dimensions. This work aims to achieve a robust IDS by using a hybrid classification model consisting of random forest (RF) and support vector machine (SVM), called RF-SVM. Here, a novel feature optimization technique based on RF has been proposed to optimize the original feature space. Later, SVM is used over the optimized feature space for classification. To test the performance of the proposed model, both scenarios: (i) Anomaly detection and (ii) Signature detection, have been considered. For anomaly detection, binary SVM is used, where the data contain two classes: (i) Normal and (ii) Attack types, whereas, for attack signature detection, multi-class SVM is used to detect each attack type. Simulation results on four standard data sets: (i) NSL-KDD, (ii) ISCX-URL2016, (iii) CICDarknet2020 and (iv) CICDoHBrw2020 demonstrate that the proposed model shows better accuracy and false alarm rate (FAR) compared to other state-of-the-art models.
更多查看译文
关键词
Cyber analytic,Feature selection,Intrusion detection system (IDS),Random forest (RF),Support vector machine (SVM)
AI 理解论文
溯源树
样例
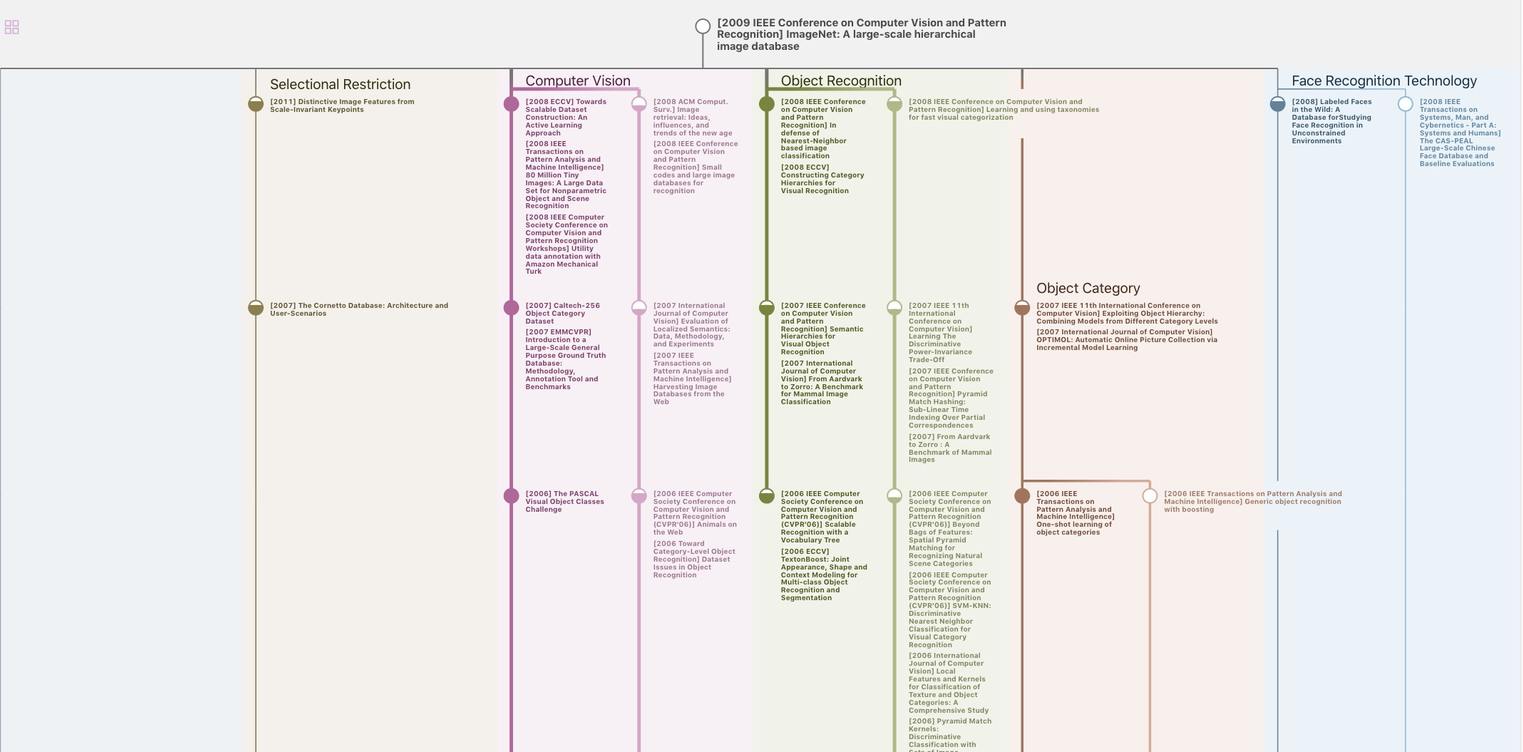
生成溯源树,研究论文发展脉络
Chat Paper
正在生成论文摘要