Sentiment analysis on cross-domain textual data using classical and deep learning approaches
MULTIMEDIA TOOLS AND APPLICATIONS(2023)
摘要
Sentiment Analysis (SA) using machine learning approaches is a fascinating field of research to unravel the opinion expressed by online users. It deals with the classification problem of opinions expressed on a product or service in review texts as positive or negative or neutral. Classification beyond binary classes poses even greater challenges using machine learning. In this paper two sets of approaches, first set of approach on traditional machine learning and second one on deep learning technique was and evaluated and analyzed for multiclass polarity classification at fine-grain level on cross-domain review dataset. The analysis of performance of traditional machine learning approaches that comprise ensemble models and incorporate semantic and diverse statistical features, shows that ensemble models are better in their category. With the proposed sentiment-document model coupled with the robustness of the proposed ensemble models, we were able to investigate and establish that the document polarity could serve as the latent mutual information that could leverage the model performance of predicting sentiments at sentence and document levels. Furthermore, the evaluation of deep learning models infers that they are more viable option for multi-class polarity classification of cross-domain dataset. They outperformed the traditional approaches based on feature extraction and feature engineering and achieved better accuracy and F 1 scores.
更多查看译文
关键词
Sentiment analysis,Contextual polarity,Cross-domain,Machine learning,Classifiers,Hybrid models,Latent mutual information,Feature engineering
AI 理解论文
溯源树
样例
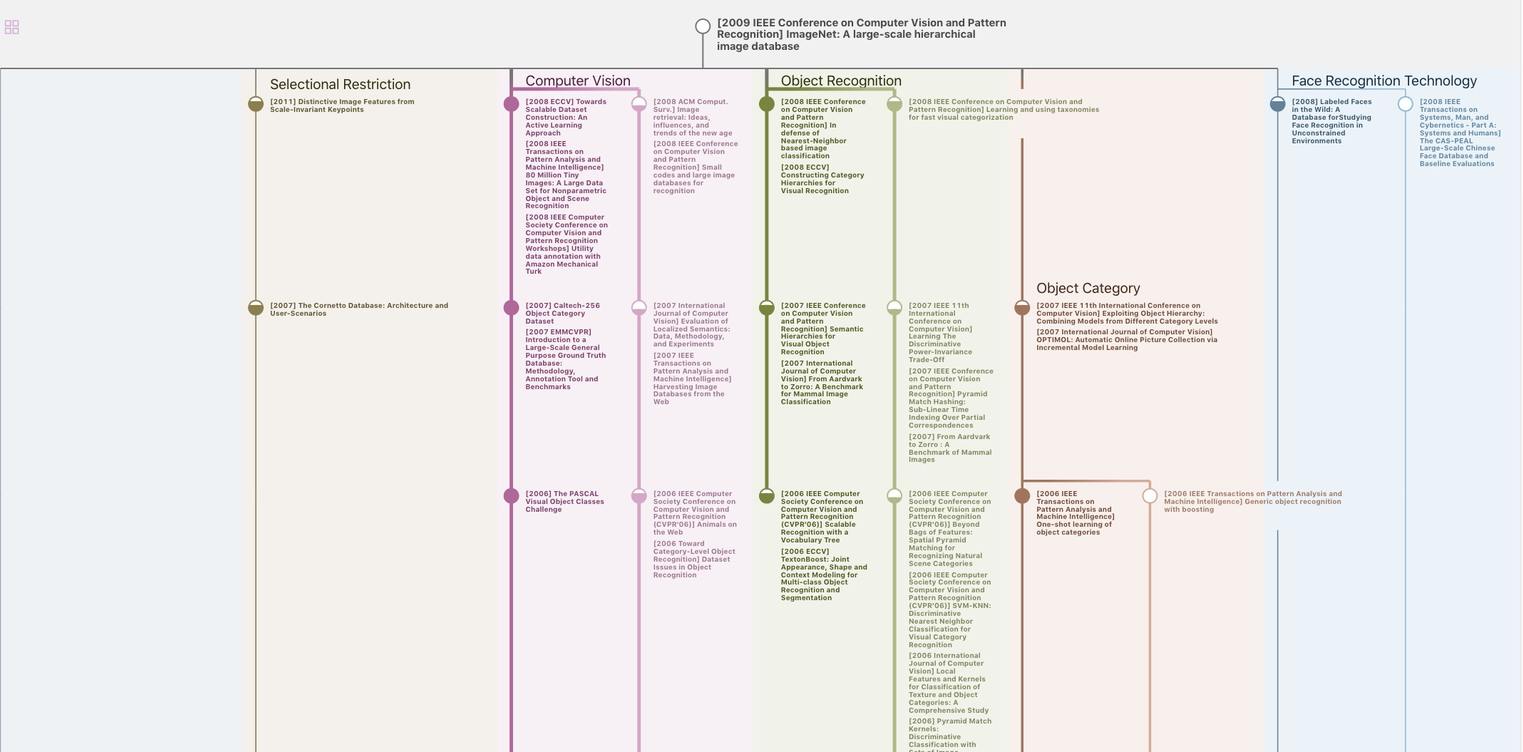
生成溯源树,研究论文发展脉络
Chat Paper
正在生成论文摘要