A light-weight stereo matching network based on multi-scale features fusion and robust disparity refinement
IET IMAGE PROCESSING(2023)
摘要
In recent years, convolutional-neural-network based stereo matching methods have achieved significant gains compared to conventional methods in terms of both speed and accuracy. Current state-of-the-art disparity estimation algorithms require many parameters and large amounts of computational resources and are not suited for applications on edge devices. In this paper, an end-to-end light-weight network (LWNet) for fast stereo matching is proposed, which consists of an efficient backbone with multi-scale feature fusion for feature extraction, a 3D U-Net aggregation architecture for disparity computation, and color guidance in a 2D convolutional neural network (CNN) for disparity refinement. MobileNetV2 is adopted as an efficient backbone in feature extraction. The channel attention module is applied to improve the representational capacity of features and multi-resolution information is adaptively incorporated into the cost volume via cross-scale connections. Further, a left-right consistency check and color guidance refinement are introduced and a robust disparity refinement network is designed with skip connections and dilated convolutions to capture global context information and improve disparity estimation accuracy with little computational cost and memory space. Extensive experiments on Scene Flow, KITTI 2015, and KITTI 2012 demonstrate that the proposed LWNet achieves competitive accuracy and speed when compared with state-of-the-art stereo matching methods.
更多查看译文
关键词
computer vision,image processing,stereo image processing
AI 理解论文
溯源树
样例
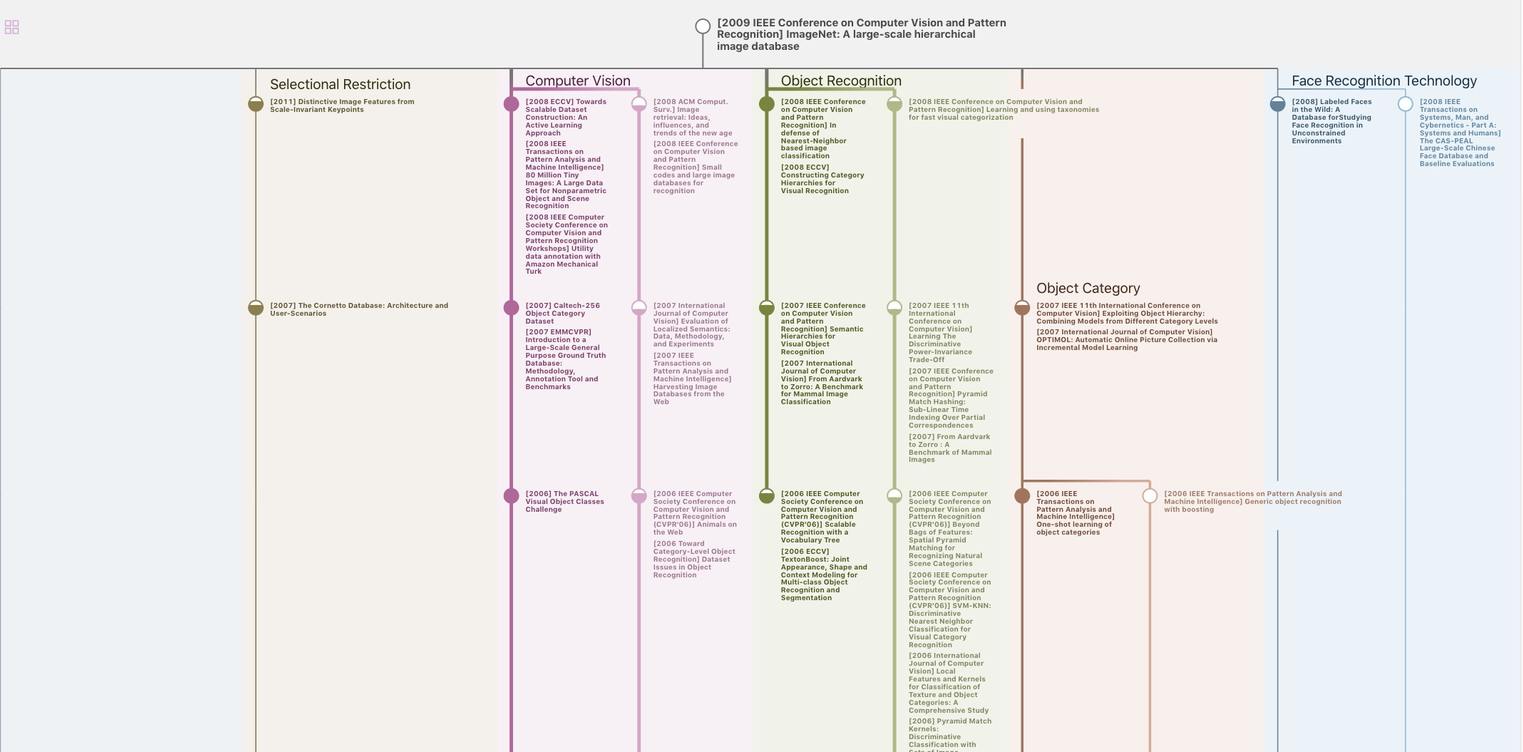
生成溯源树,研究论文发展脉络
Chat Paper
正在生成论文摘要