Energy Consumption Forecasts by Gradient Boosting Regression Trees
MATHEMATICS(2023)
摘要
Recent years have seen an increasing interest in developing robust, accurate and possibly fast forecasting methods for both energy production and consumption. Traditional approaches based on linear architectures are not able to fully model the relationships between variables, particularly when dealing with many features. We propose a Gradient-Boosting-Machine-based framework to forecast the demand of mixed customers of an energy dispatching company, aggregated according to their location within the seven Italian electricity market zones. The main challenge is to provide precise one-day-ahead predictions, despite the most recent data being two months old. This requires exogenous regressors, e.g., as historical features of part of the customers and air temperature, to be incorporated in the scheme and tailored to the specific case. Numerical simulations are conducted, resulting in a MAPE of 5-15% according to the market zone. The Gradient Boosting performs significantly better when compared to classical statistical models for time series, such as ARMA, unable to capture holidays.
更多查看译文
关键词
energy forecasting,machine learning,neural networks,Italian energy market,gradient boosting decision tree
AI 理解论文
溯源树
样例
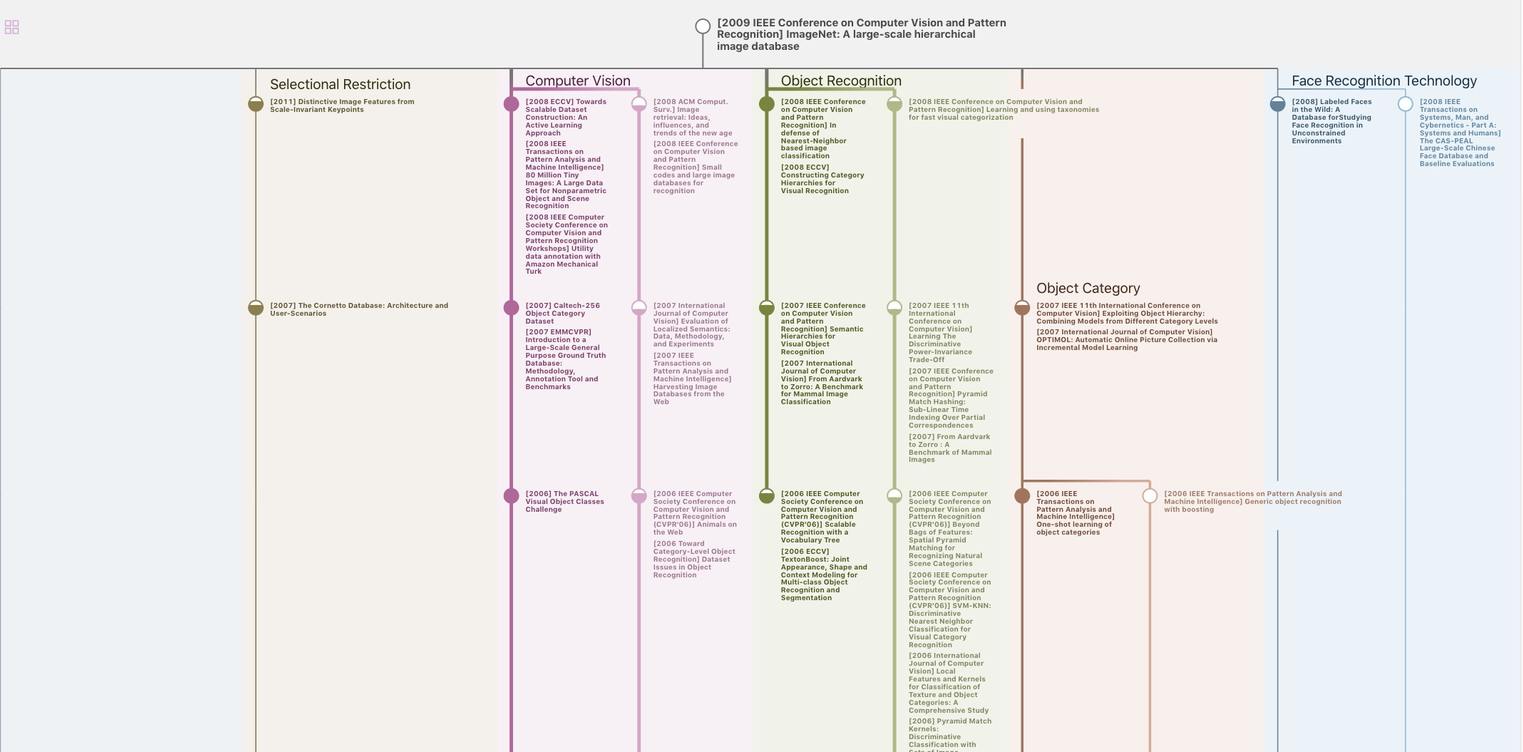
生成溯源树,研究论文发展脉络
Chat Paper
正在生成论文摘要