Multi-radar data fusion for maritime moving target detection based on three-dimensional sliding window
INTERNATIONAL JOURNAL OF REMOTE SENSING(2023)
摘要
With the continuous improvement of radar resolution, distributed characteristics are presented with regard to maritime moving targets, which occupy multi-resolution cells in Synthetic Aperture Radar (SAR) image domain. However, the current constant false alarm rate (CFAR) detection algorithms rarely consider the impact of distributed characteristics on target detection performance, and thus the corresponding performance evaluation methods could not be applicable to this issue. In this paper, a multi-station fusion detection method for maritime moving target (MMT) is presented based on three-dimensional (3D) sliding window. Firstly, the multi-station echoes in Cartesian-Doppler frequency rate (DFR) domain are obtained under the simple-transmitting and multiple-receiving operation configuration, and the 3D sliding window is designed to achieve the optimal matching for a specified target with any moving direction in terms of its prior information, i.e. Radar Cross Section (RCS), radar resolution and target size. Then, the target cells within the sliding window are directly processed by means of the M/N criterion, and thus to avoid target detection performance loss caused by the artificial construction of extended detection statistics. Finally, a novel quantitative evaluation method in regards to a high-resolution radar is designed by mining the relationship between the target detection performance and the occupied cell number, which could greatly improve detection probability of distributed targets on the premise of a constant false alarm rate. The proposed algorithm does not need to design complex extended detection statistics, which provides a feasible way for robust detection and performance evaluation for a high-resolution radar. Simulation results verify the effectiveness of this research.
更多查看译文
关键词
Distributed target,multi-station fusion,DFR,3D sliding window,detection probability
AI 理解论文
溯源树
样例
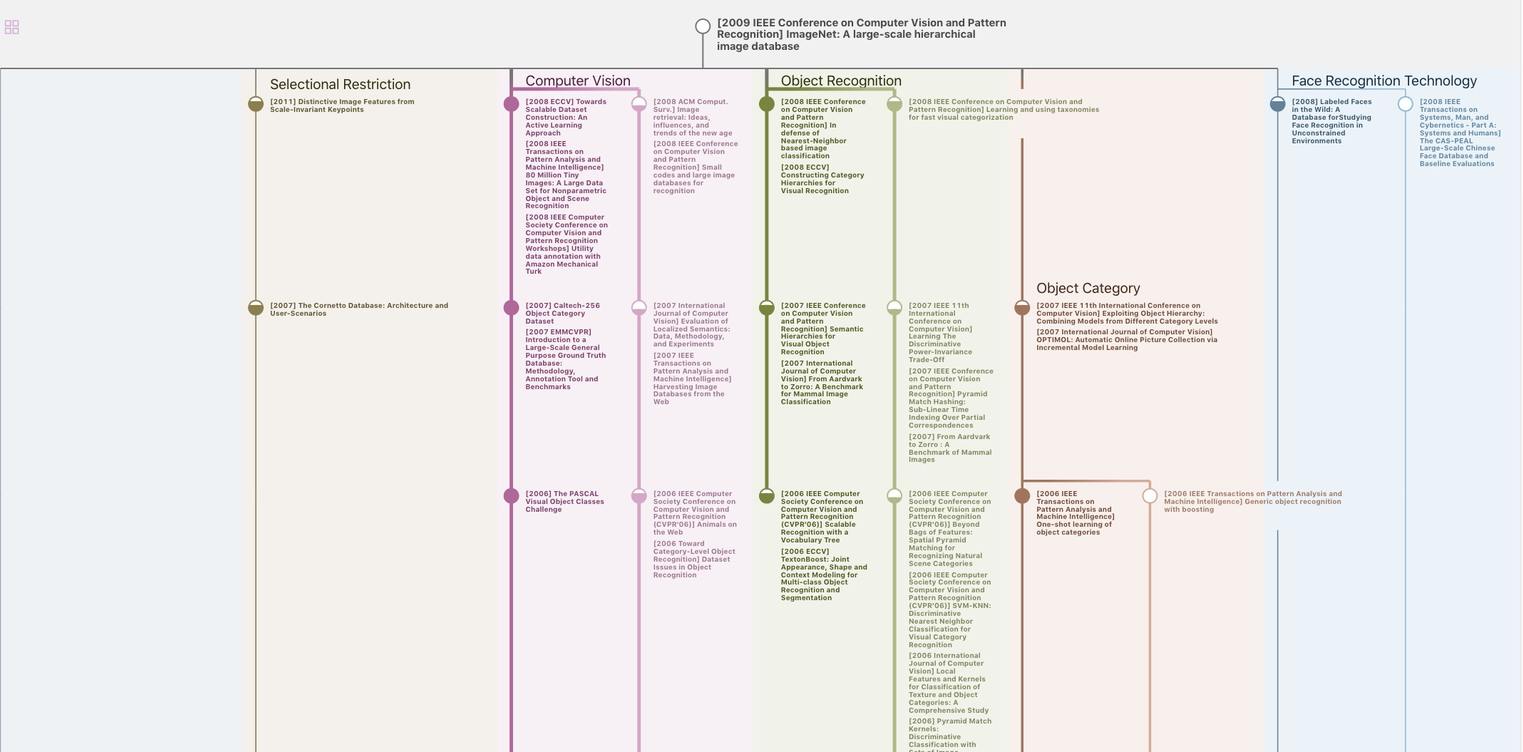
生成溯源树,研究论文发展脉络
Chat Paper
正在生成论文摘要