Bayesian solution to the monotone likelihood in the standard mixture cure model
STATISTICA NEERLANDICA(2023)
摘要
An advantage of the standard mixture cure model over an usual survival model is how it accounts for the population heterogeneity. It allows a joint estimation for the distribution related to the susceptible and non-susceptible subjects. The estimation algorithm may provide +/-infinity$$ \pm \infty $$ coefficients when the likelihood cannot be maximized. This phenomenon is known as Monotone Likelihood (ML), common in survival and logistic regressions. The ML tends to appear in situations with small sample size, many censored times, many binary or unbalanced covariates. Particularly, it occurs when all uncensored cases correspond to one level of a binary covariate. The existing frequentist solution is an adaptation of the Firth correction, originally proposed to reduce bias of maximum likelihood estimates. It prevents +/-infinity$$ \pm \infty $$ estimates by penalizing the likelihood, with the penalty interpreted as the Bayesian Jeffreys prior. In this paper, the penalized likelihood of the standard mixture cure model is considered with different penalties (Bayesian priors). A Monte Carlo simulation study indicates good inference results, especially for balanced data sets. Finally, a real application involving a melanoma data illustrates the approach.
更多查看译文
关键词
cure rate,logistic link,melanoma,penalized likelihood,survival analysis
AI 理解论文
溯源树
样例
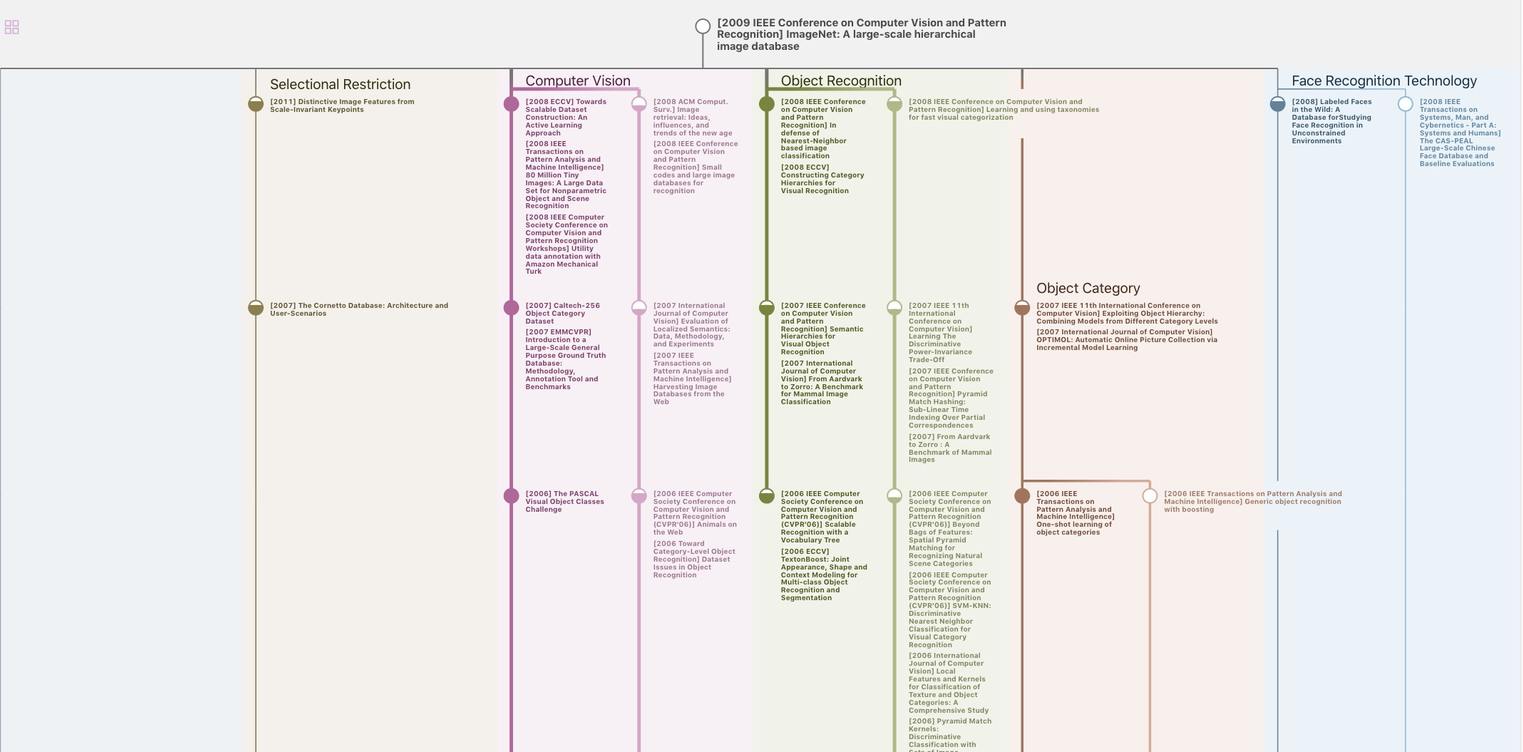
生成溯源树,研究论文发展脉络
Chat Paper
正在生成论文摘要