Degradation of benzene in anaerobic groundwater in the typical cold industrial region: Identification, interactions, and optimization of nitrate-/sulfate-reducing assemblages
BIOCHEMICAL ENGINEERING JOURNAL(2023)
摘要
Nitrate (NO3-) and sulfate (SO42-) are the electron acceptors (EAs) for benzene biodegradation in anaerobic groundwater. The efficiency of benzene biodegradation depends on the strength of the interaction between benzene degradation and EA reduction combinations. However, the interactions are less studied. Hence, the present study focuses on the effect of groundwater EA coexistence on the anaerobic degradation of benzene under cold climate conditions. Herein, response surface methodology (RSM) and artificial neural network (ANN) are used to predict and optimize the EAs (NO3- and SO42-) for the anaerobic degradation of benzene. The root mean square error and correlation coefficient of RSM and ANN fitting models fulfill the accuracy requirements. Experimental results show that NO3- stimulation degrades benzene more significantly than SO42- stimulation. Thus, the coexistence of NO3- and SO42- has a favorable effect on benzene degradation. Besides, the degradation efficiency of benzene is not affected by a single EA, and there is a higher degradation efficiency under the coexistence of two EAs. Furthermore, the optimization model predicts that for 29.93 mg/L benzene concentra-tion in groundwater, the NO3- and SO42- concentrations with high microbial degradation efficiencies are 0.87 mmol/L and 1.12 mmol/L, respectively. In addition, the best degradation efficiencies predicted by the RSM and ANN models are 75.42% and 73.65%, respectively. The RSM model had greater predictive ability than ANN model. Hence, this study provides an idea for the sustainable bioremediation of an aromatic hydrocarbon-contaminated environment where multiple EAs coexist.
更多查看译文
关键词
Groundwater,Anaerobic biodegradation,Electron acceptor optimization,Response surface methodology-artificial neural,network model
AI 理解论文
溯源树
样例
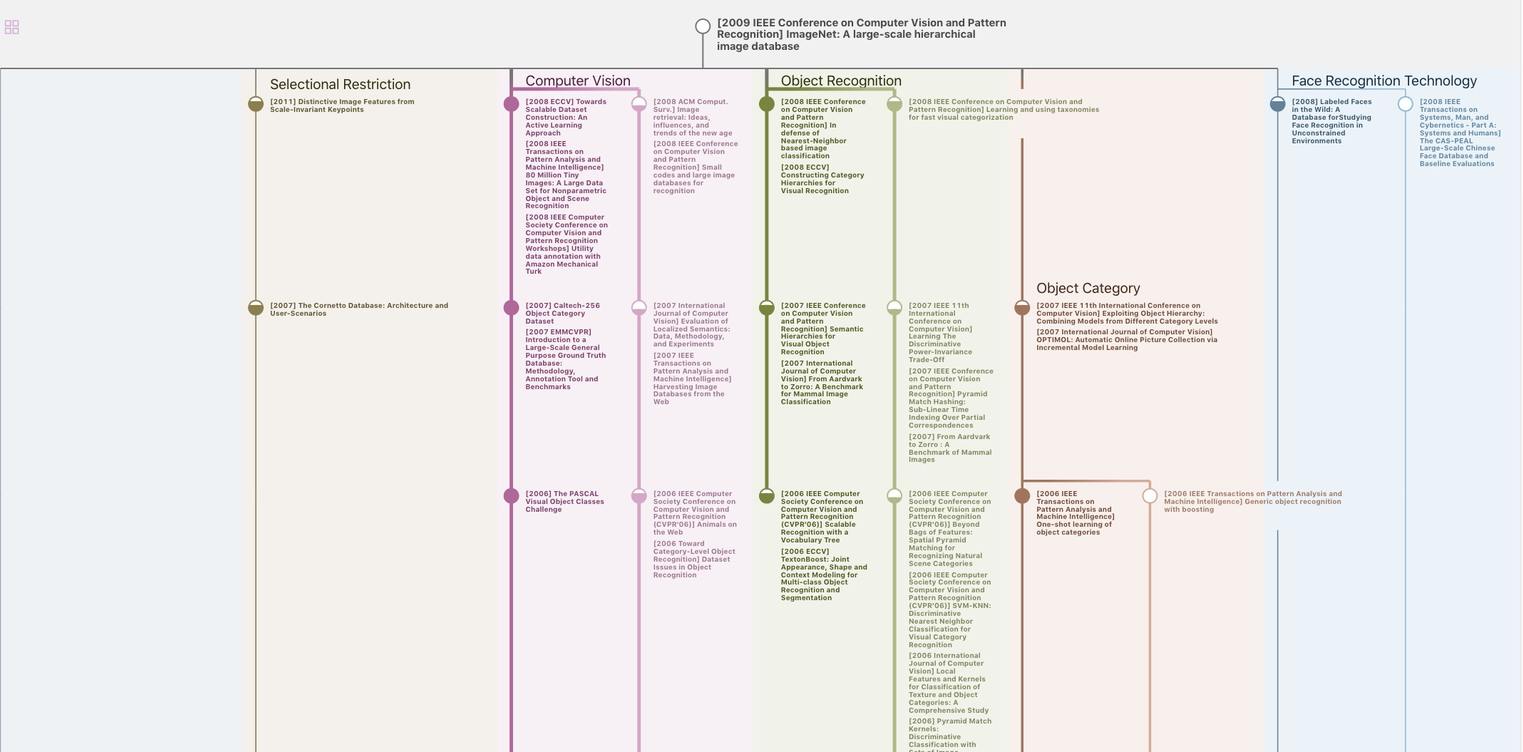
生成溯源树,研究论文发展脉络
Chat Paper
正在生成论文摘要