Pedestrian gender classification on imbalanced and small sample datasets using deep and traditional features
NEURAL COMPUTING & APPLICATIONS(2023)
摘要
In this manuscript, imbalanced and small sample space (IB-SSS) dataset problems for pedestrian gender classification using fusion of selected deep and traditional features (PGC-FSDTF) are considered. In this regard, data preparation is first done through data augmentation and preprocessing steps to handle imbalanced classification problem and environmental effects, respectively. The proposed approach follows different types of feature extraction schemes, for instance, pyramid histogram of oriented gradients, hue saturation value histogram, deep visual features of DenseNet201 and InceptionResNetV2-based convolutional neural network architectures. The parallel fusion method computes the maximum and average values-based features from the learned features of both deep networks. Features are selected through features selection methods such as entropy and principal component analysis (PCA). The subsets of features are serially fused and provided to multiple classifiers to perform gender classification on IB-SSS datasets. Resultantly, the proposed PGC-FSDTF method shows better results in terms of different accuracies (overall, mean, and balanced), and area under curve on selected datasets. Further, improved results are achieved on applied datasets using PCA-based selected features and medium Gaussian support vector machine (M-SVM) classifier. These results on different datasets confirm that the selected feature combination provides a way to handle IB-SSS issues for PGC effectively.
更多查看译文
关键词
IB-SSS datasets,Data augmentation,Deep and traditional features,Features selection and fusion,Gender classification
AI 理解论文
溯源树
样例
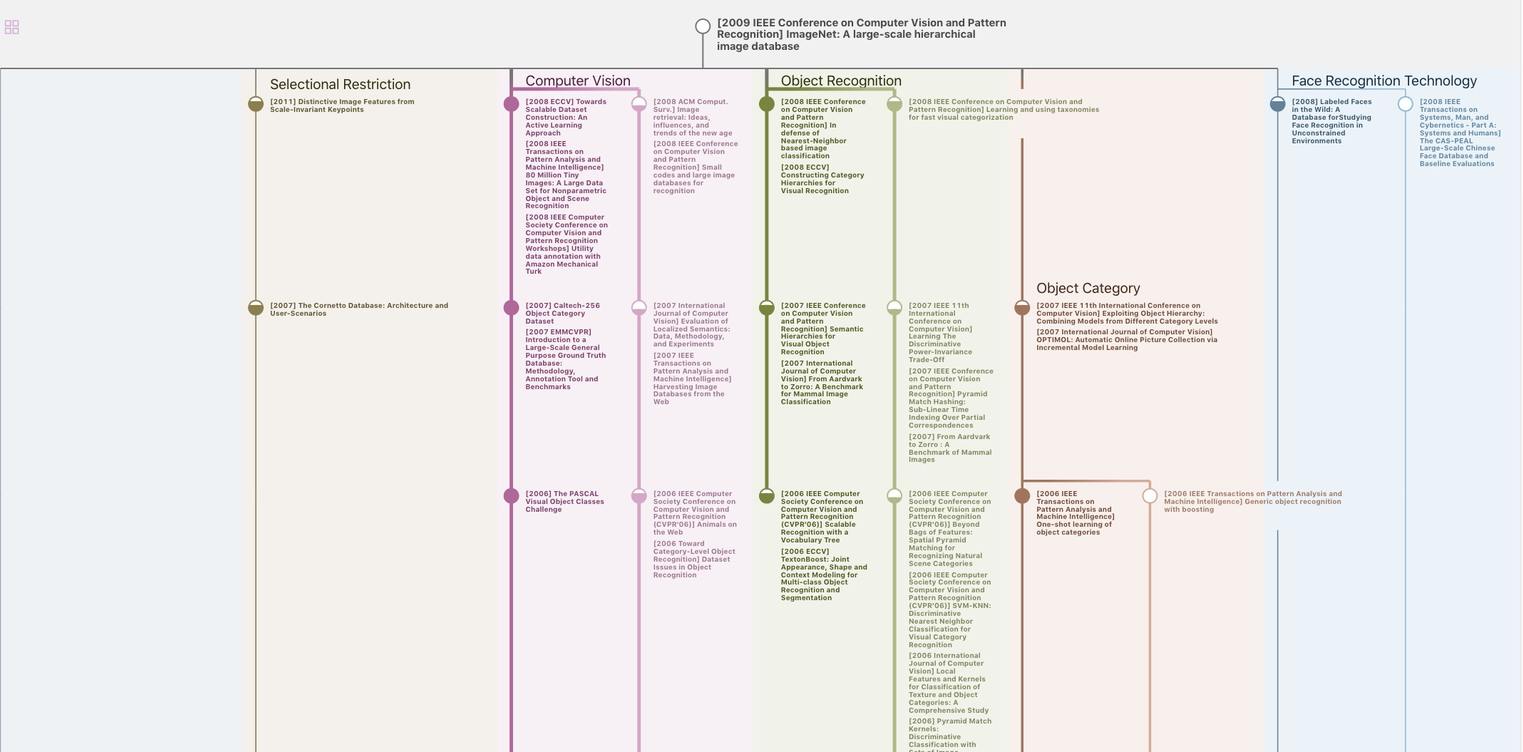
生成溯源树,研究论文发展脉络
Chat Paper
正在生成论文摘要