A hybrid data-driven framework for satellite telemetry data anomaly detection
ACTA ASTRONAUTICA(2023)
摘要
Anomaly detection based on telemetry data is a prominent health condition monitoring task that plays an increasingly crucial role in identifying unexpected events and improving overall satellite reliability. In this paper, a new hybrid data-driven framework for satellite telemetry data anomaly detection is constructed by an inte-gration of ensemble empirical mode decomposition with complexity deviation index and grey wolf optimizer -optimized support vector machine. Initially, the ensemble empirical mode decomposition algorithm is employed to decompose the raw telemetry data into a collection of intrinsic mode functions that represent different inherent oscillatory modes or characteristics. A critical intrinsic mode function selection method, i.e., the complexity deviation index method, is proposed based on the sample entropy theory to select the most appropriate intrinsic mode functions with the goal of improving the sufficiency of the ensemble empirical mode decomposition algorithm and enhancing the anomalous characteristics of the telemetry data. Subsequently, a grey wolf optimizer-optimized support vector machine model is developed to forecast the variation tendency of the synthesized series of the selected intrinsic mode functions. The prediction residual between the ground truth and model estimator is calculated and the optimal threshold for anomaly detection is set according to the principle of maximum Youden index. Finally, the proposed method is applied to analyze and detect anomalies in telemetry data collected from a real-world satellite. The experimental results verify the capability of the proposed method in anomaly detection for satellite telemetry data.
更多查看译文
关键词
Ensemble empirical mode decomposition,Complexity deviation index,Grey wolf optimizer,Support vector machine,Anomaly detection
AI 理解论文
溯源树
样例
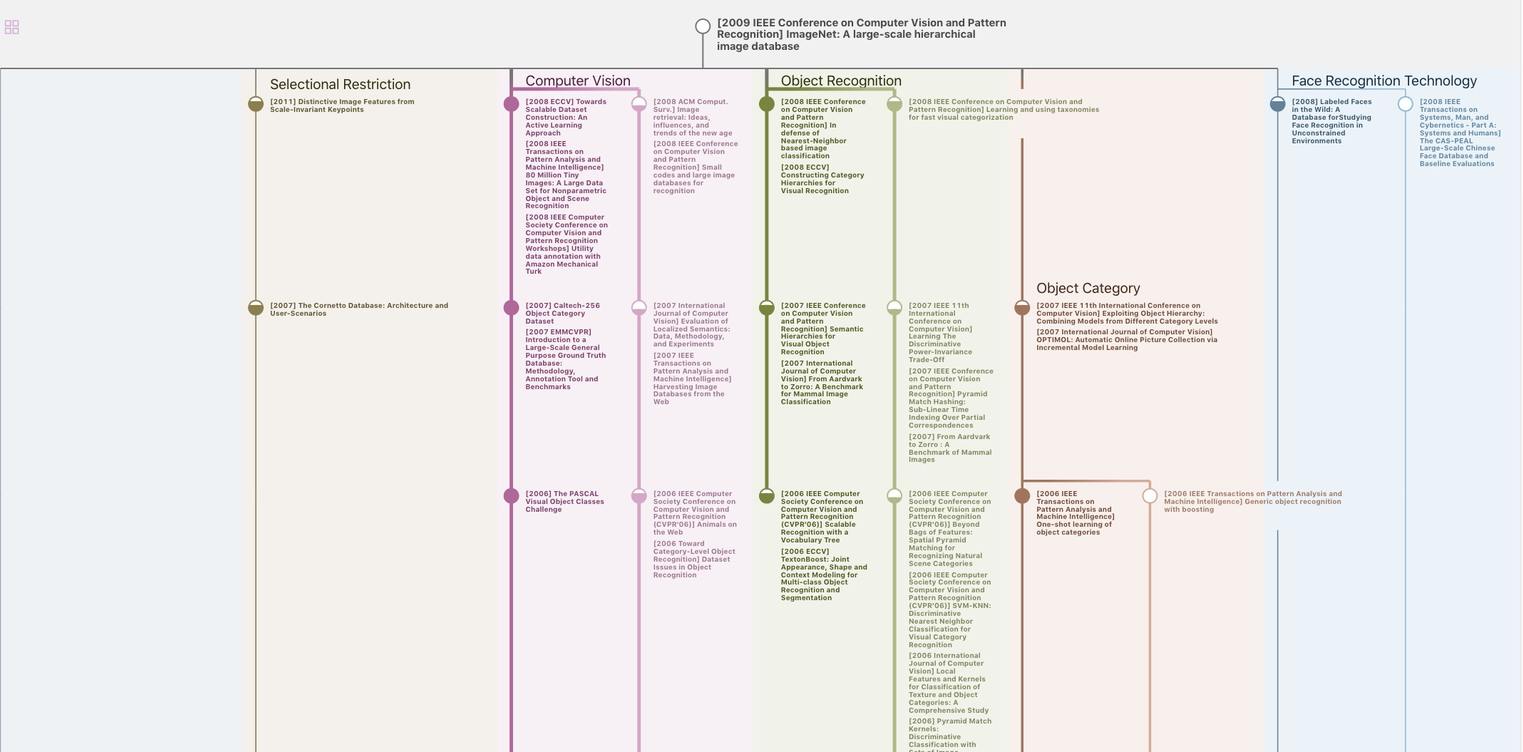
生成溯源树,研究论文发展脉络
Chat Paper
正在生成论文摘要