Improved sparse low-rank model via periodic overlapping group shrinkage and truncated nuclear norm for rolling bearing fault diagnosis
MEASUREMENT SCIENCE AND TECHNOLOGY(2023)
摘要
The early faults of rolling bearings are the common causes of rotating machinery failures. Rolling bearings with local faults usually generate periodic shocks during operation, but the pulse information is easily masked by a large number of random shocks and noise. To effectively diagnose the early fault information of rolling bearings, a dual-dimensional sparse low-rank (DDSLR) model is proposed in this paper, which can simultaneously extract the sparsity within and across groups and periodic self-similarity of fault signal. In the DDSLR model, a newly developed dimension transformation operator is used to transform the fault signal between one-dimensional vector and low-rank matrix, and the periodic overlapping group shrinkage and truncated nuclear norm are used to improve the traditional sparse low-rank model. In addition, the setting rules of periodic prior and parameters in the DDSLR model are discussed, so that the DDSLR model has certain adaptive ability. Finally, the DDSLR model is proved to be a multi-convex optimization problem, and its solution algorithm is derived by using soft threshold operator and majorization-minimization algorithm under the framework of block coordinate descent method. The results of simulation analysis and experiments show that the proposed DDSLR model has higher fault signal estimation accuracy and better fault feature extraction performance than some classical sparse noise reduction models.
更多查看译文
关键词
rolling bearing,fault diagnosis,improved sparse low-rank model,sparsity within and across groups,periodic self-similarity
AI 理解论文
溯源树
样例
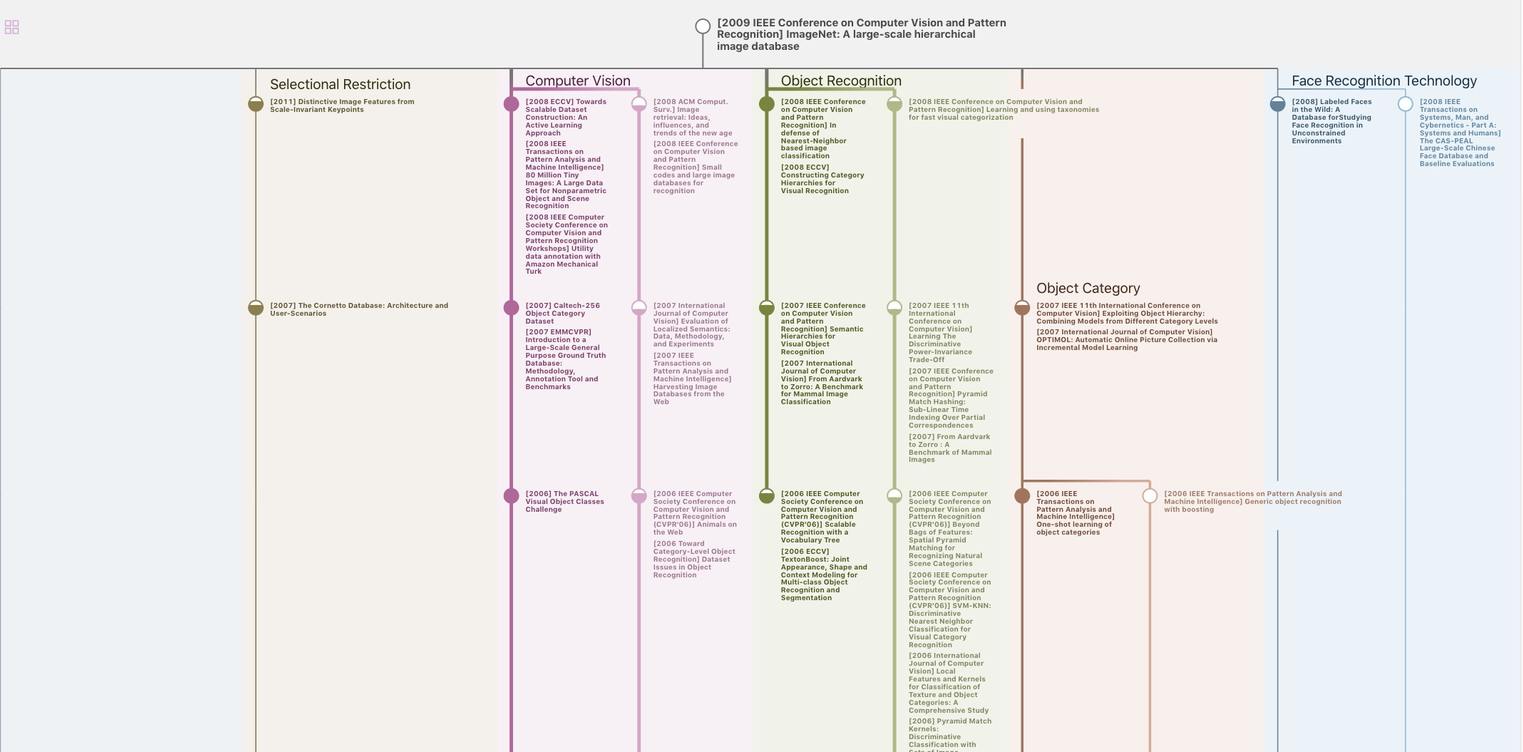
生成溯源树,研究论文发展脉络
Chat Paper
正在生成论文摘要