A novel long-short term memory network approach for stress model updating for excavations in high stress environments
GEORISK-ASSESSMENT AND MANAGEMENT OF RISK FOR ENGINEERED SYSTEMS AND GEOHAZARDS(2023)
摘要
Digitalisation has increased access to large amounts of data for rock engineers. Machine learning presents an opportunity to aid data interpretation. The operators of Garson Mine use a microseismic database to calibrate a mine-scale finite difference model, used to assess seismic risk to inform mine operations. A Long-Short Term Memory (LSTM) network is proposed for stress model updating. The model is trained using microseismic data, geology, and geomechanical parameters from the FLAC3D model. Two LSTM networks are developed for Garson Mine: (1) predicting far field principal stresses in the FLAC3D model, and (2) predicting the far field six-component stress tensors in the model. Various LSTM network hyperparameters were analyzed to determine the architecture for the targets: input encoding and pre-processing, training solver, network layer architecture, and cost function. Architectures were chosen based on the corrected Akaike Information Criterion (AIC(c)), coefficient of determination (R-2), and percent capture (%C). When predicting principal stresses, AIC(c) = -59.62, R-2 = 0.996, and %C = 97%, and when predicting the six-component stress tensor AIC(c) = -45.50, R-2 = 0.997, and %C = 80%. This research represents progress towards continuous, automated updating of numerical models such that rapid, more accurate forecasts of changes in stress conditions will allow earlier reaction to challenging stress environments, increasing safety of excavations.
更多查看译文
关键词
Machine learning,stress modelling updating,Long-Short Term Memory network
AI 理解论文
溯源树
样例
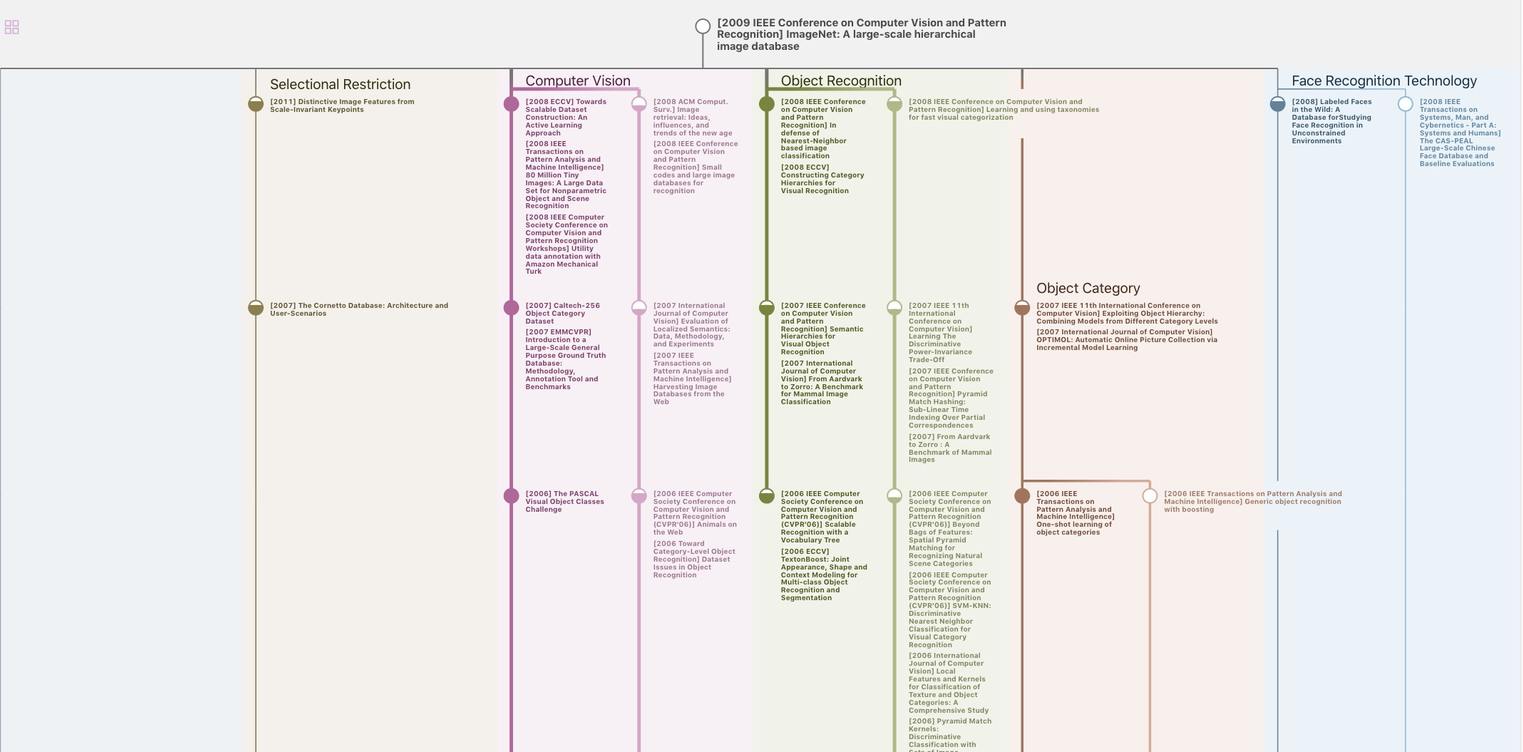
生成溯源树,研究论文发展脉络
Chat Paper
正在生成论文摘要