Few-shot learning in realistic settings for text CAPTCHA recognition
NEURAL COMPUTING & APPLICATIONS(2023)
摘要
Text-based captcha is commonly used by many commercial websites. Most existing captcha recognition methods rely on deep learning and large-scale labeled data. Recently, few-shot learning has shown its effectiveness in various visual classification tasks in the case of insufficient data. However, the performance of current few-shot learning methods will deteriorate in realistic settings with class-imbalance and cross-domain. In this paper, we have proposed a novel captcha solver based on prototypical networks and model-agnostic meta-learning. Two major improvements, including multi-source domain data augmentation and intra-class variance distance weighting method, are proposed to alleviate the performance degradation problems caused by cross-domain and class imbalance. Our approaches achieve an average character accuracy of more than 90% in 5-shot and 10-shot tasks and an astonishing attack rate of 88% in one-shot tasks. The efficacy of this work may promote the application of few-shot learning in realistic settings.
更多查看译文
关键词
Text CAPTCHAs,Few-shot Learning,Cross-domain,Data-imbalance
AI 理解论文
溯源树
样例
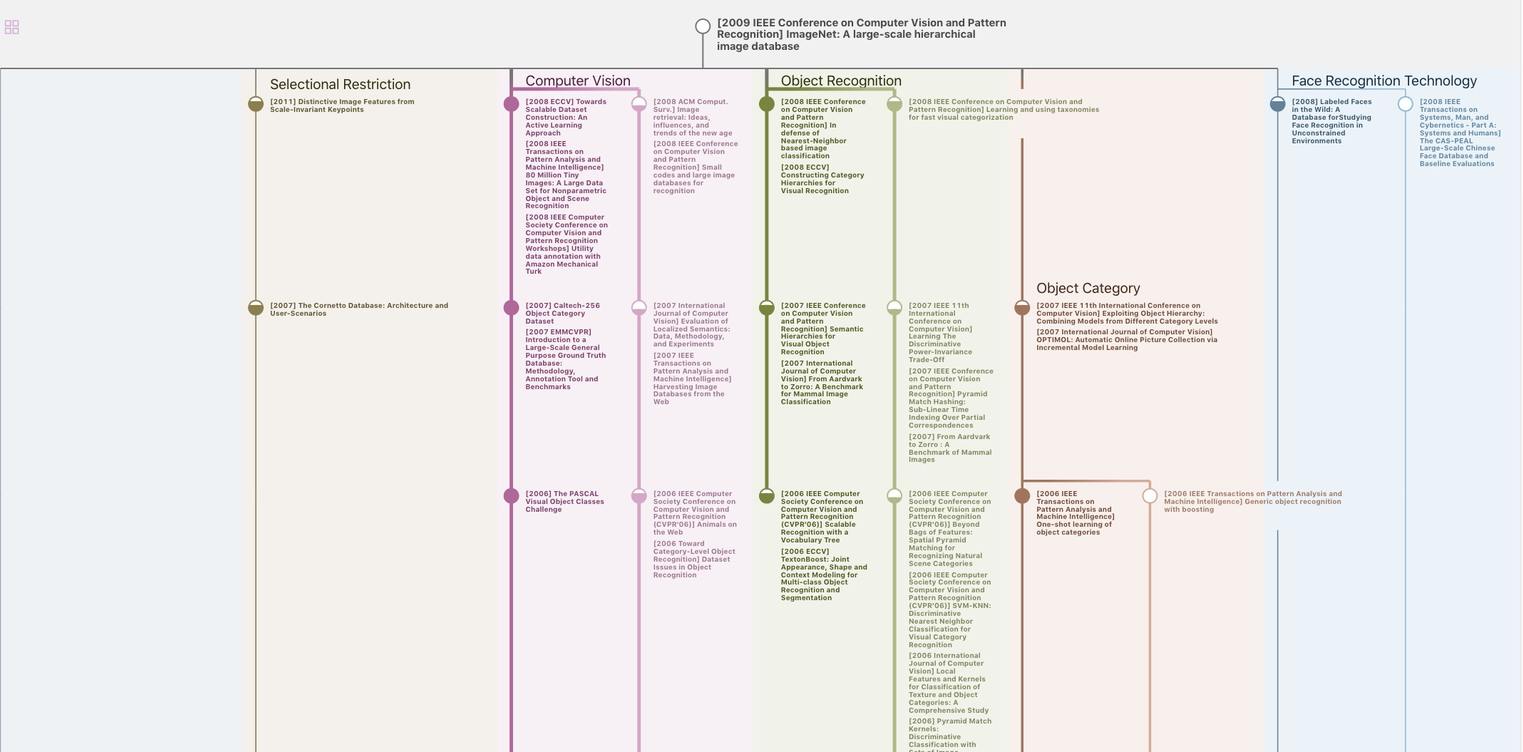
生成溯源树,研究论文发展脉络
Chat Paper
正在生成论文摘要