Spatial multi-scale attention U-improved network for blood vessel segmentation
SIGNAL IMAGE AND VIDEO PROCESSING(2023)
摘要
Vessel segmentation in digital subtraction angiography (DSA) is of great significance for the diagnosis, evaluation and detection of cerebral diseases. Manual segmentation is relatively time-consuming and subjective, so that automatic cerebrovascular segmentation technology has good application value in the treatment of cerebrovascular diseases. The traditional segmentation algorithm performs poorly because of the complexity of cerebrovascular structure, large-scale changes, and the impact of noise such as artifacts in DSA. In this work, using depth learning method and attention mechanism, we propose a spatial multi-scale attention U improved network (SMAU-Net) for vessel segmentation of DSA images. The network mainly consists of three parts: multi-scale spatial attention module, feature aggregate module, and detail supervision module. Using various attention mechanisms to pay attention to scale, space and channel information, the semantic, edge information and thin vessel features are enhanced. We applied the proposed method to the benchmark retinal vessel dataset CHASE and DSAC (cerebral DSA imaging dataset made in our laboratory). The experimental results show that the proposed SMAU-Net achieves the F1 score of 86.32% and the precision of 90.04%, which is superior to other models and is an improvement of 1.98 and 5.71% over the baseline U-Net. The experiment also proves that the method can be extended to various vascular segmentation tasks and has good visual diagnosis quality.
更多查看译文
关键词
Attention mechanism,Digital subtraction angiography,Multi-scale,Vessel segmentation
AI 理解论文
溯源树
样例
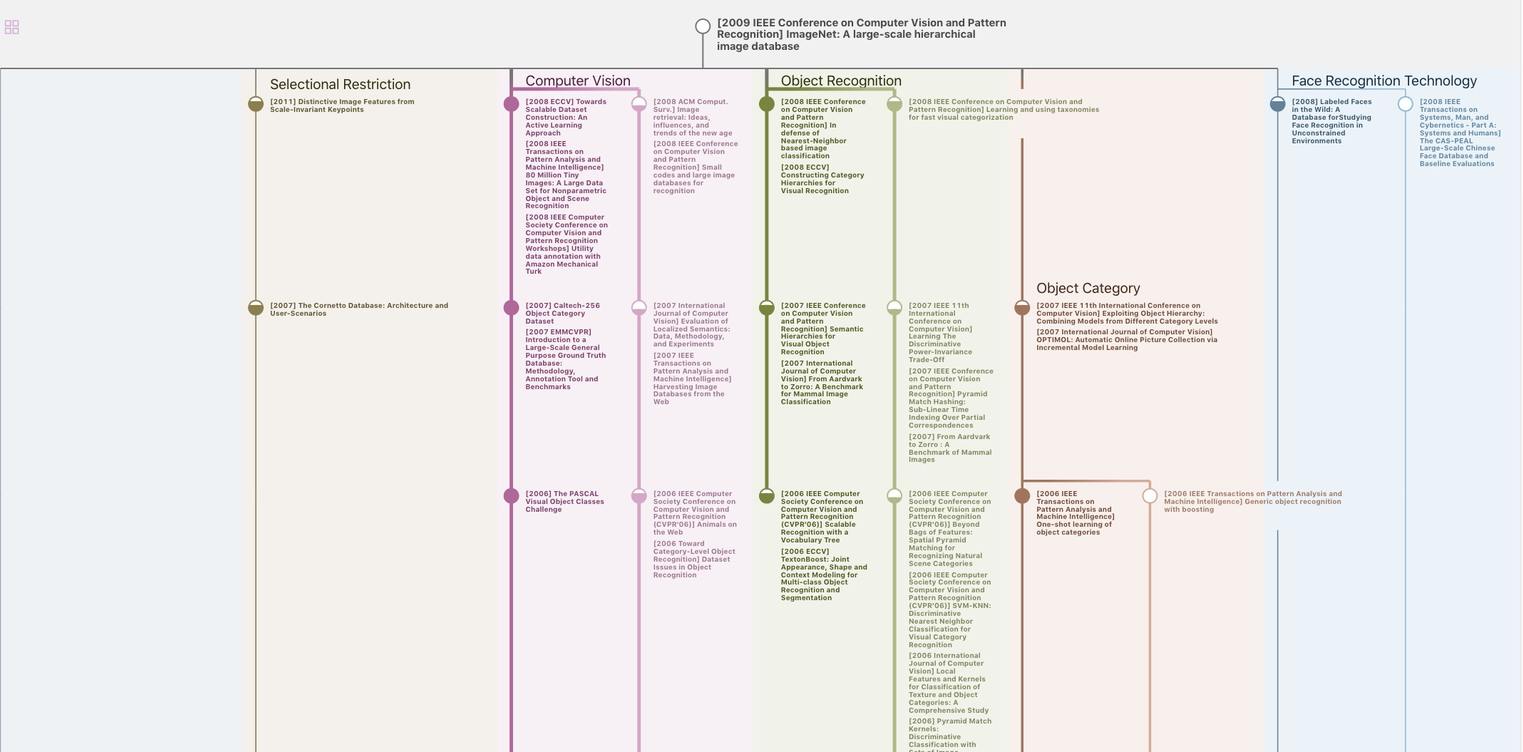
生成溯源树,研究论文发展脉络
Chat Paper
正在生成论文摘要