Ordinal few-shot learning with applications to fault diagnosis of offshore wind turbines
RENEWABLE ENERGY(2023)
摘要
It is an important but challenging work to develop novel fault diagnosis (FD) methods of offshore wind turbines (WTs) for their maintenance cost that accounts for 20%-35% of the total lifecycle cost. In FD of offshore WTs, there are two common problems: lack of high-quality label data and ignoring fault severity. In this study, we apply the prototypical networks in few-short learning to cope with a small high-quality label data, and adopt the ordinal regression method to consider fault severity. To sum up, we develop a novel ordinal classification prototypical networks (OCPN) model by introducing ordinal regression into prototypical networks, which is suitable for the FD of offshore WTs. The real case data gathered by an enterprise engaging in equipment condition monitoring and fault diagnosis in China is used to verify OCPN's effectiveness. The experimental results show that the OCPN model outperforms several competing models in terms of better multi-classification performance. In practical engineering applications, the OCPN model is flexible for diagnostic experts to consider the priority of fault levels by introducing decision preference into the loss function.
更多查看译文
关键词
Few-shot learning,Prototypical networks,Ordinal regression,Offshore wind turbines,Fault diagnosis,Decision preference
AI 理解论文
溯源树
样例
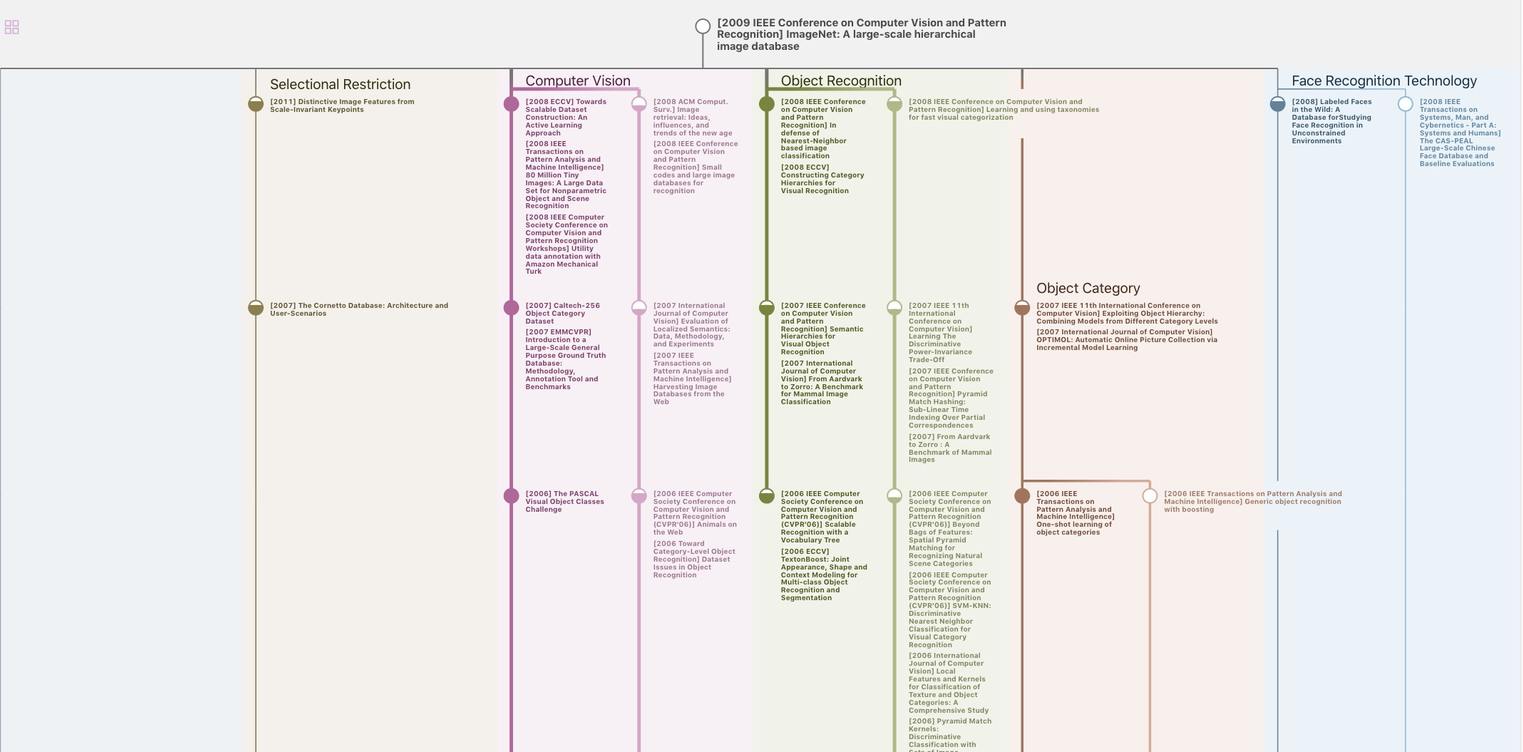
生成溯源树,研究论文发展脉络
Chat Paper
正在生成论文摘要