Machine learning exploration of the mobility and environmental assessment of toxic elements in mining-associated solid wastes
JOURNAL OF CLEANER PRODUCTION(2023)
摘要
Continuous development of the mining industry has led to the production of large volumes of solid wastes. Toxic elements (TEs) have been identified in mining-associated solid wastes, whose leaching potential poses a sig-nificant environmental risk. In this study, we introduce a synthesis framework titled Fast mobIlity Evaluation and environmentaL inDex (FIELD). The physicochemical properties of solid waste, element properties, and total TEs concentrations were used as input variables to construct the machine learning model and predict the TEs frac-tions. Novel environmental indexes were then proposed based on both the total TEs concentrations and corre-sponding chemical speciation. Applying the framework to a case study of coal fly ash (CFA) demonstrated robust performance of the deep neural network model with good generalization capabilities (R2 = 0.83 on the testing set), indicating it can rapidly and accurately predict TEs fractions. The TEs fractions were found to be primarily affected by the element properties. The results also demonstrate that the proposed environmental indexes are superior to current indexes for identifying the most environmentally hazardous TEs within a specific CFA sample, as well as for identifying the most dangerous CFA sample based on TEs fractions. Compared with traditional laboratory analysis and environmental risk assessment methods, the FIELD framework proposed in this study is more efficient and achieves robust results, with important reference significance for environmental pollution control.
更多查看译文
关键词
Mobility evaluation,Environmental risks,Mining-associated solid wastes,Sequential extraction,Machine learning,Coal fly ash
AI 理解论文
溯源树
样例
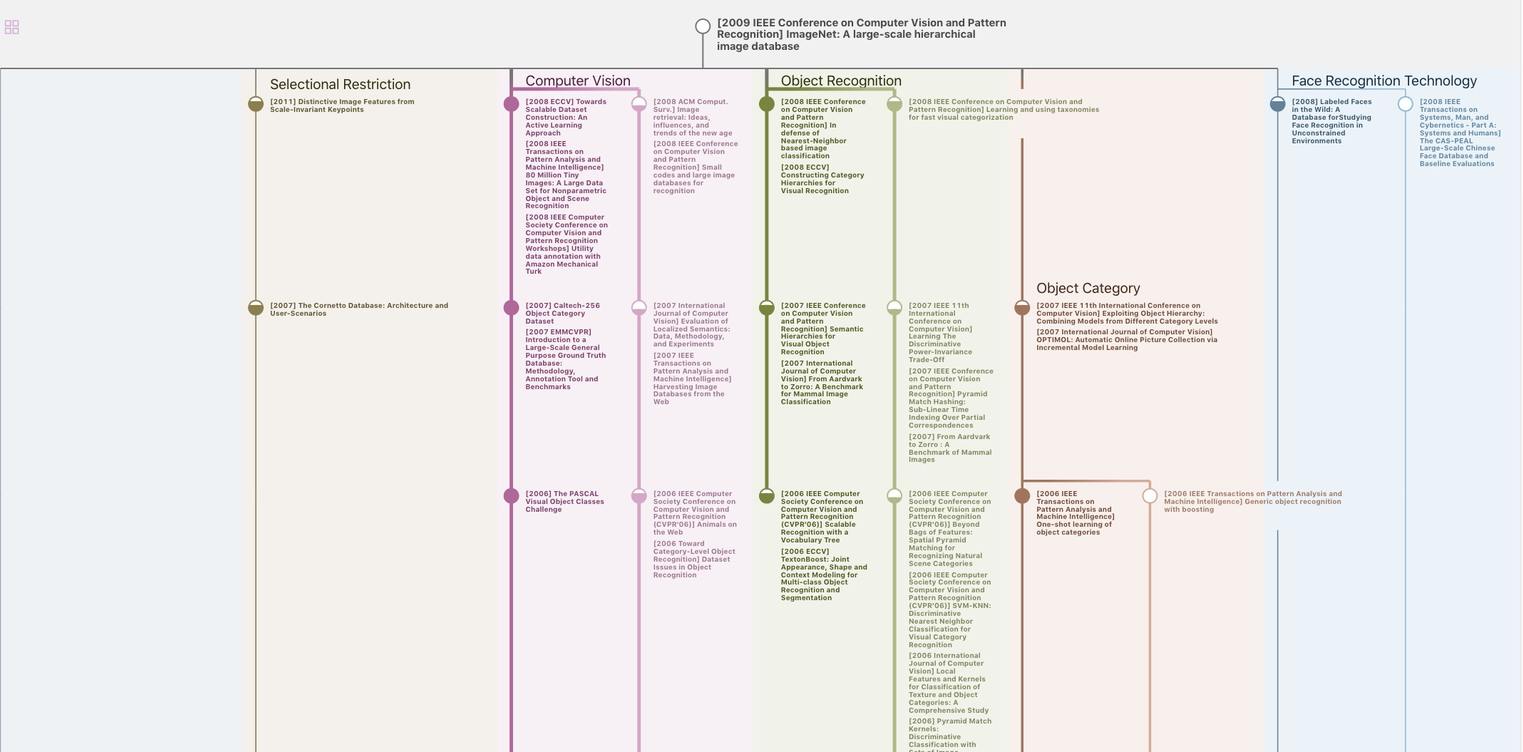
生成溯源树,研究论文发展脉络
Chat Paper
正在生成论文摘要