The optimal dynamic regret for smoothed online convex optimization with squared l 2 norm switching costs
JOURNAL OF THE FRANKLIN INSTITUTE-ENGINEERING AND APPLIED MATHEMATICS(2023)
摘要
Online convex optimization (OCO) with switching costs is a key enabler for cloud resource provision, online portfolio optimization, and many other applications. Surprisingly, very little theoretical under-standing is known. In this study, we investigate OCO with squared l 2 norm switching cost (OCOl2SC) for three kinds of loss functions: (a) generally convex, (b) convex and smooth, and (c) strongly convex and smooth. We design customized gradient descent algorithms for OCOl2SC in these three cases: specif-ically, SOGD (smoothed online gradient descent) for generally convex loss functions, OOMD (online op-timistic mirror descent) for convex and smooth functions, and OMGD (online multiple gradient descent) for strongly convex and smooth loss functions. We theoretically analyze the three algorithms' dynamic regrets and their upper bounds. By showing that the dynamic regrets match their lower bounds, we con-clude that the three algorithms achieve the order optimal or near-optimal dynamic regret bounds in the corresponding case. Numerical studies further verify the remarkable performance of the three algorithms.(c) 2023 The Franklin Institute. Published by Elsevier Inc. All rights reserved.
更多查看译文
AI 理解论文
溯源树
样例
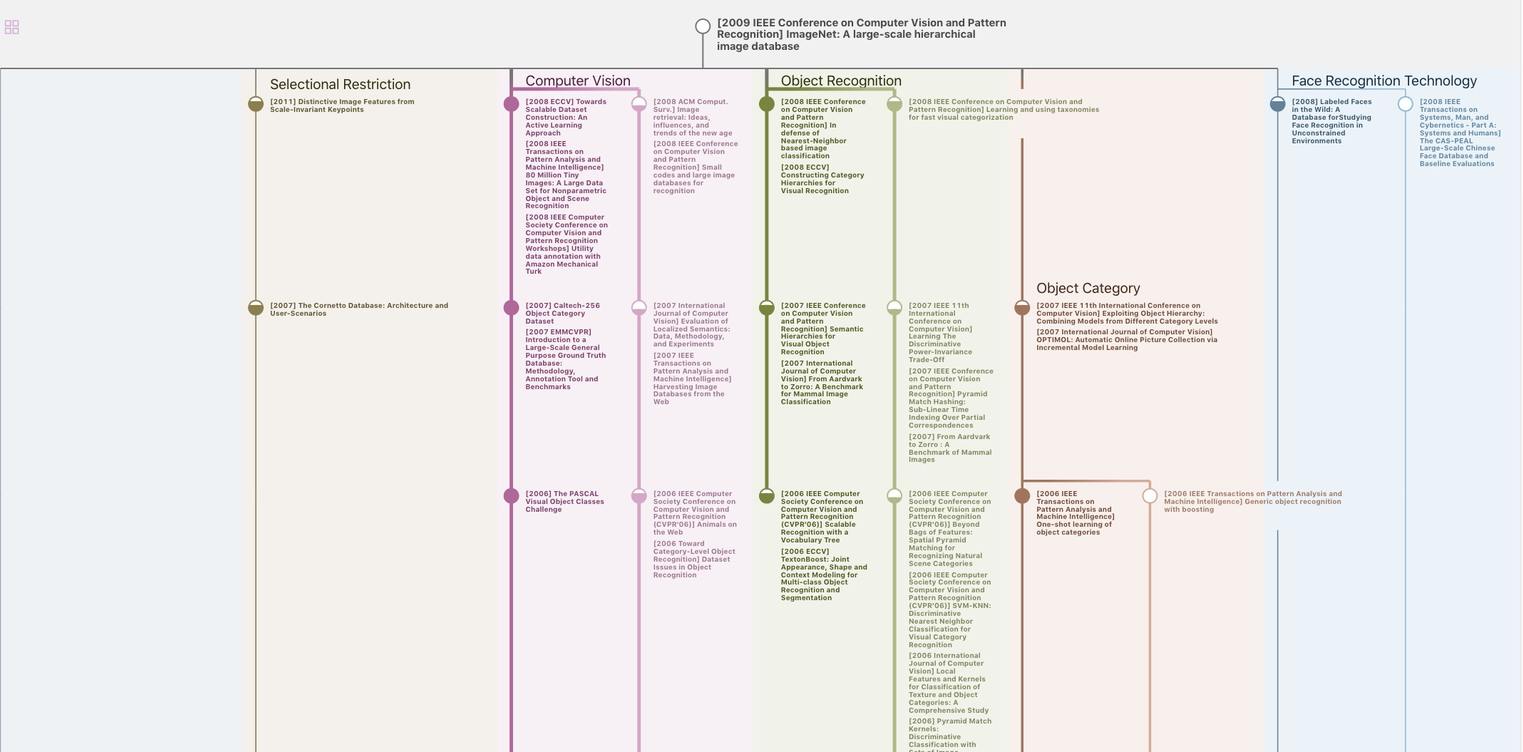
生成溯源树,研究论文发展脉络
Chat Paper
正在生成论文摘要