Federated learning-based colorectal cancer classification by convolutional neural networks and general visual representation learning
INTERNATIONAL JOURNAL OF IMAGING SYSTEMS AND TECHNOLOGY(2023)
摘要
Colorectal cancer is the fourth fatal disease in the world, and the massive burden on the pathologists related to the classification of precancerous and cancerous colorectal lesions can be decreased by deep learning (DL) methods. However, the data privacy of the patients is a big challenge for being able to train deep learning models using big medical data. Federated Learning is a rising star in this era by providing the ability to train deep learning models on different sites without sacrificing data privacy. In this study, the Big Transfer model, which is a new General Visual Representation Learning method and six other classical DL methods are converted to the federated version. The effect of the federated learning is measured on all these models on four different data settings extracted from the MHIST and Chaoyang datasets. The proposed models are tested for single learning, centralized learning, and federated learning. The best AUC values of federated learning on Chaoyang are obtained by the Big Transfer and VGG models at 90.77% and 90.76%, respectively, whereas the best AUC value on MHIST is obtained by the Big Transfer model at 89.72%. The overall obtained results of models on all data settings show that the contribution of Federated Learning with respect to single learning is 4.71% and 11.68% for the "uniform" and "label-biased" data settings of Chaoyang, respectively, and 6.89% for the "difficulty level-biased" data setting of MHIST. Thus, it is experimentally shown that federated learning can be applied to the field of computational pathology for new institutional collaborations.
更多查看译文
关键词
big transfer,colorectal cancer,computational pathology,convolutional neural network,federated learning,general visual representation learning
AI 理解论文
溯源树
样例
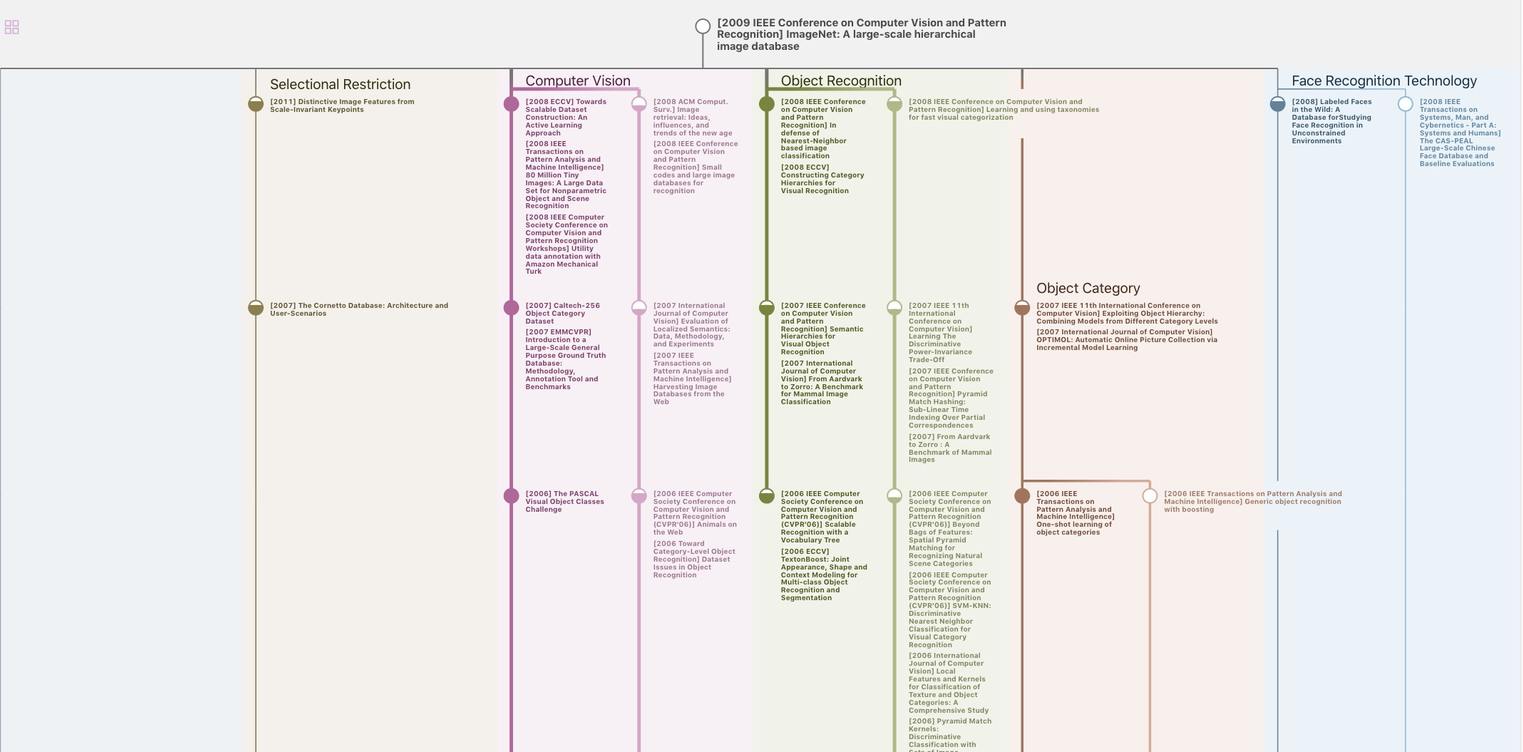
生成溯源树,研究论文发展脉络
Chat Paper
正在生成论文摘要