Deep-learning-based super-resolution and classification framework for skin disease detection applications
OPTICAL AND QUANTUM ELECTRONICS(2023)
摘要
One of the most dangerous malignancies in global diseases is skin cancer. The best way to survive skin cancer is to detect it in its early stages. Its diagnosis is made by medical imaging with dermoscopy, which provides High-Resolution (HR) lesion images to simplify the detection process. Dermoscopy is not available to everyone, and other available imaging technologies, such as mobile devices, deteriorate the image quality required for diagnosis. This research presents a proposed novel Enhanced Deep Super-Resolution Generative Adversarial Network (EDSR-GAN) model to generate HR skin disease images from Low-Resolution (LR) ones. Particularly, a new design of the loss function is adopted for more details and for creating HR images. Experimental results reveal that the proposed model provides a higher performance on the HAM10000 dataset compared with other models applied on the same dataset. Additionally, for evaluating the proposed model, a variety of metrics are employed, including the Peak Signal-to-Noise Ratio (PSNR), Mean Square Error (MSE), Structural Similarity Index (SSIM), Multi-scale Structural Similarity Index (MS-SSIM), and histogram characteristics, to conduct thorough and impartial comparisons and identify the best models according to performance training time, and storage space. By obtaining an accuracy of 98.9958%, the results show that the proposed model outperforms traditional and previous models for color and texture reconstruction and recognition.
更多查看译文
关键词
GAN,Deep learning,Skin images,SSIM,MS-SSIM,PSNR,Single-Image Super-Resolution (SISR)
AI 理解论文
溯源树
样例
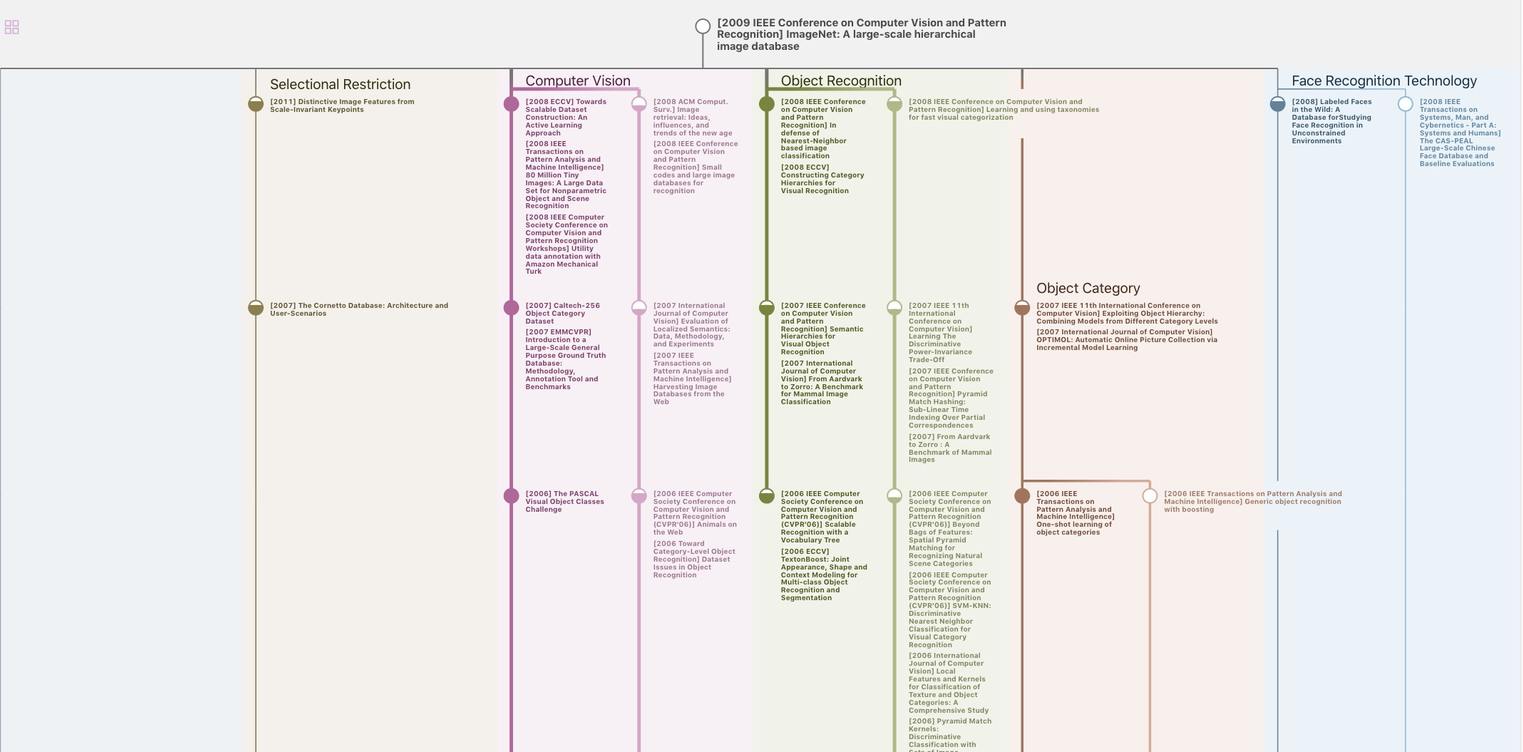
生成溯源树,研究论文发展脉络
Chat Paper
正在生成论文摘要