Temporal Extraction of Complex Medicine by Combining Probabilistic Soft Logic and Textual Feature Feedback
APPLIED SCIENCES-BASEL(2023)
摘要
In medical texts, temporal information describes events and changes in status, such as medical visits and discharges. According to the semantic features, it is classified into simple time and complex time. The current research on time recognition usually focuses on coarse-grained simple time recognition while ignoring fine-grained complex time. To address this problem, based on the semantic concept of complex time in Clinical Time Ontology, we define seven basic features and eleven extraction rules and propose a complex medical time-extraction method. It combines probabilistic soft logic and textual feature feedback. The framework consists of two parts: (a) text feature recognition based on probabilistic soft logic, which is based on probabilistic soft logic for negative feedback adjustment; (b) complex medical time entity recognition based on text feature feedback, which is based on the text feature recognition model in (a) for positive feedback adjustment. Finally, the effectiveness of our approach is verified in text feature recognition and complex temporal entity recognition experimentally. In the text feature recognition task, our method shows the best F1 improvement of 18.09% on the Irregular Instant Collection type corresponding to utterance l17. In the complex medical temporal entity recognition task, the F1 metric improves the most significantly, by 10.42%, on the Irregular Instant Collection type.
更多查看译文
关键词
probabilistic soft logic,temporal entity extraction,logic rules,text feature automation,text feature feedback
AI 理解论文
溯源树
样例
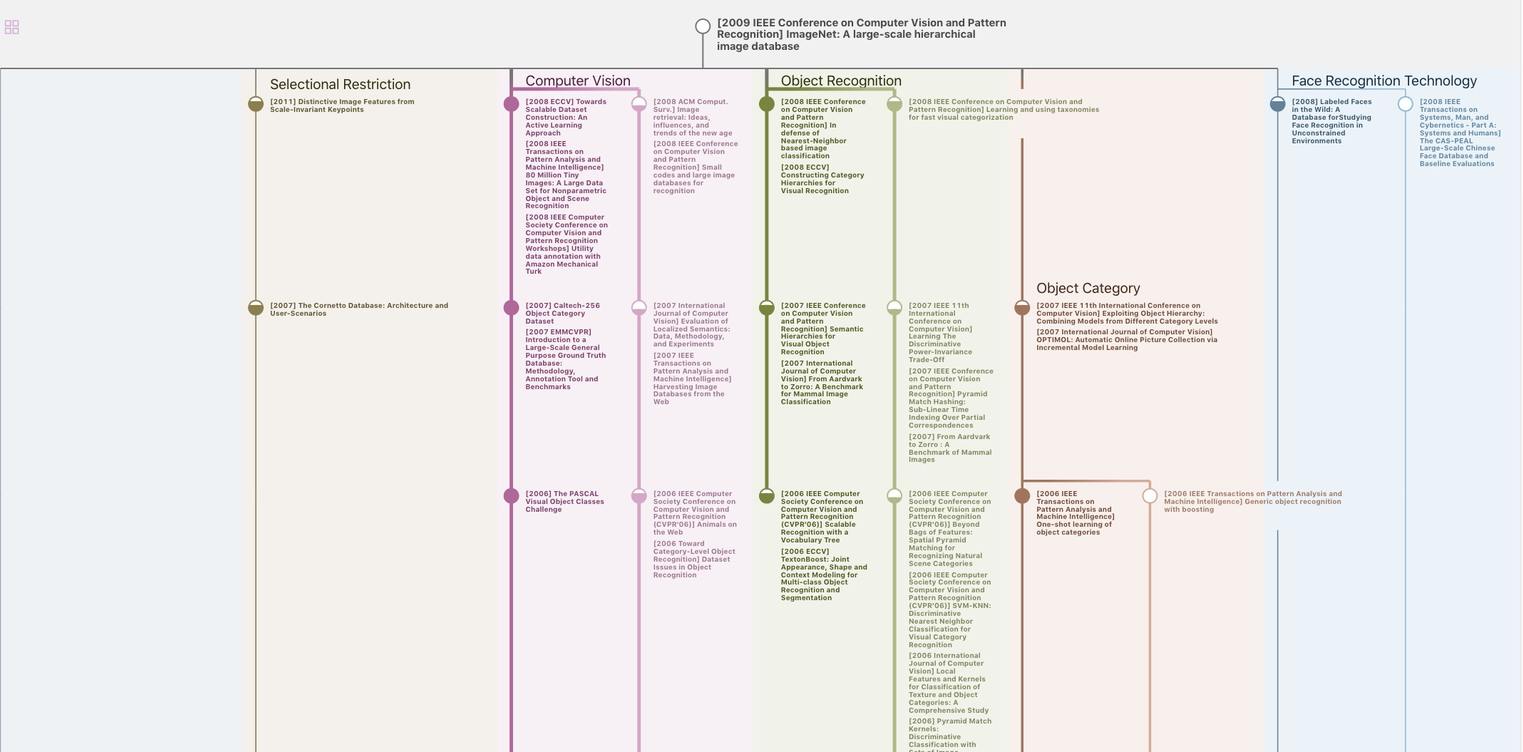
生成溯源树,研究论文发展脉络
Chat Paper
正在生成论文摘要