Advancing vapor-deposited perovskite solar cells via machine learning
JOURNAL OF MATERIALS CHEMISTRY A(2023)
摘要
The last decade witnessed a rapid development in perovskite solar cells in both power conversion efficiency and device lifetimes, mainly relying on lab-scale solution-processing technologies. Vapor-deposited perovskite solar cells compatible with the existing large-scale electronic industry, however, have significantly lagged behind. In this study, we extracted 220 reliable data from peer-reviewed publications on thermally evaporated perovskite solar cells and utilized 20 features consisting of material components and fabrication parameters for machine learning (ML) training. By combining the random forest model with the genetic algorithm, we constructed a performance prediction model with a root mean square error (RMSE) of 3.16% and a Pearson coefficient of 0.67. The SHapley Additive exPlanations (SHAP) analysis shows that the ratio of cations to anions in the perovskite layer and the annealing temperature contribute the most to power conversion efficiency. After detailed screening of the multi-dimensional variable spaces, we suggested an optimum device architecture as well as its critical fabrication conditions, forecasting the highest PCE of 26.1%, which exceeds the state-of-the-art efficiency record reported so far. Our work helps to accelerate the development of vapor-deposited perovskite solar cells and push forward their industrial production.
更多查看译文
关键词
perovskite solar cells,solar cells,machine learning,vapor-deposited
AI 理解论文
溯源树
样例
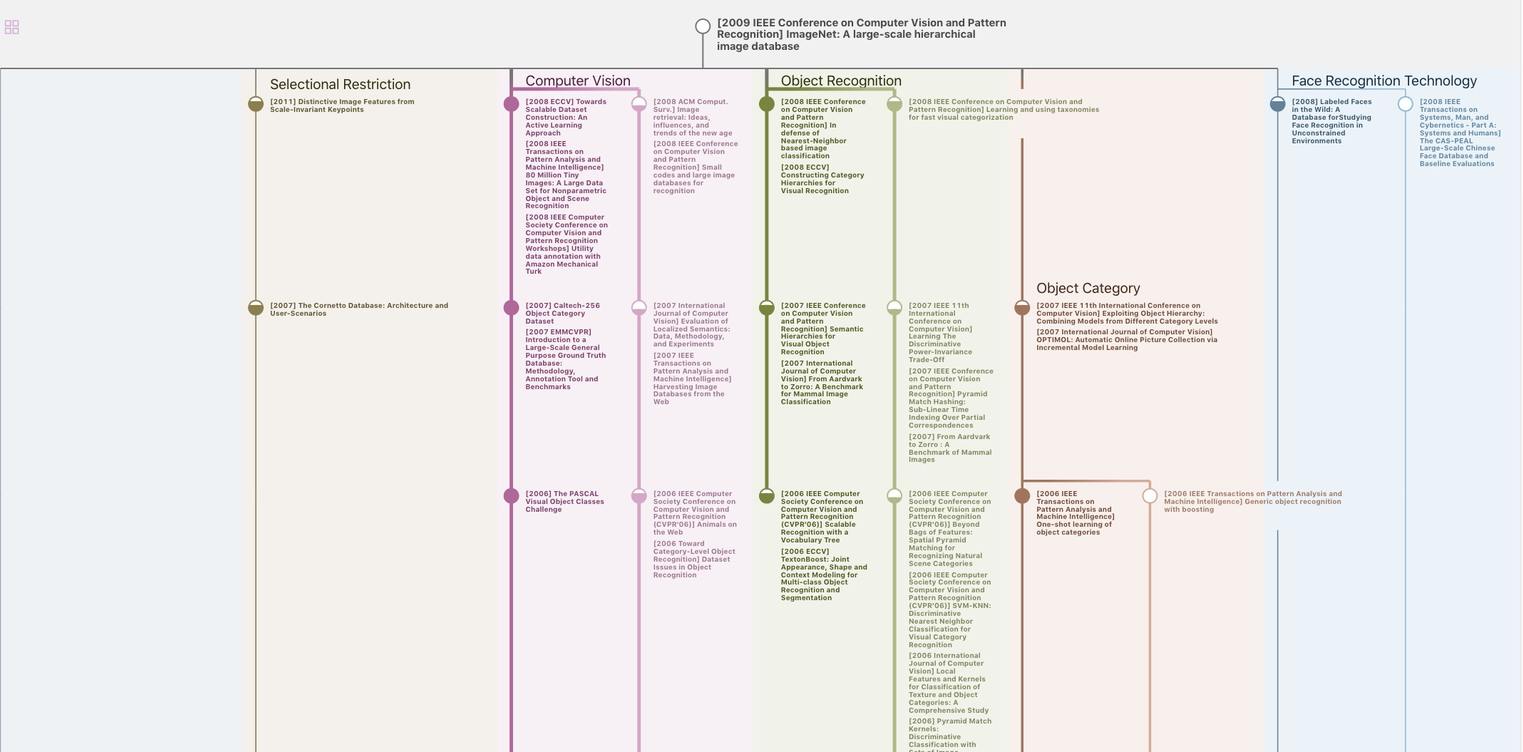
生成溯源树,研究论文发展脉络
Chat Paper
正在生成论文摘要