Enhancing unsupervised domain adaptation by exploiting the conceptual consistency of multiple self-supervised tasks
SCIENCE CHINA-INFORMATION SCIENCES(2023)
摘要
Unsupervised domain adaptation (UDA) aims to transfer the knowledge from a label-rich source domain to an unlabeled target domain. Current approaches mainly focus on aligning the target domain’s data distribution with the source domain, but lacking attention to allowing target data to guide which features need to be captured. Source data dominates feature extraction. As a result, target embedding would lose some vital discriminative features and limit UDA in complex tasks. In this paper, we argue that utilizing auxiliary tasks to capture target-intrinsic patterns, which do not depend on source supervision, could further enhance UDA. Multiple auxiliary tasks understand the instance concept from different perspectives. Our findings show that exploiting the conceptual consistency of multiple auxiliary tasks to characterize the common part of these various understandings should reveal the target’s hidden ground truth. Furthermore, we propose a novel method named multiple self-supervision conceptual consistency domain adaptation (MSCC). Experiments and analysis on benchmark datasets show the effectiveness of our idea and method.
更多查看译文
关键词
deep learning,transfer learning,domain adaptation,domain shift,self-supervised
AI 理解论文
溯源树
样例
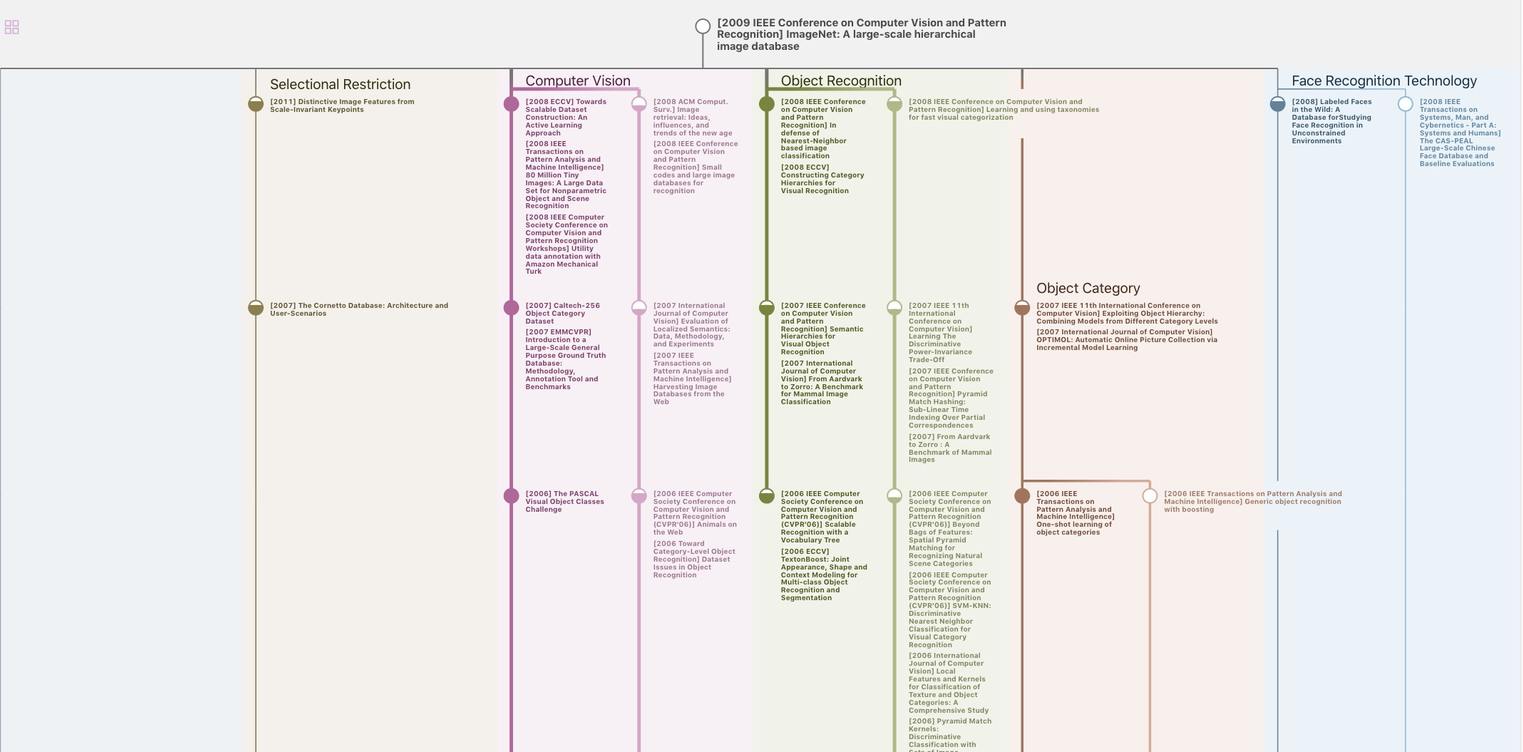
生成溯源树,研究论文发展脉络
Chat Paper
正在生成论文摘要