An Improved Adaptive Kalman Filter based on Auxiliary Model for State of Charge Estimation with Random Missing Outputs
JOURNAL OF THE ELECTROCHEMICAL SOCIETY(2023)
摘要
In this study, an improved adaptive Kalman filter based on auxiliary model (IAKF-AM) is proposed for estimating the state of charge (SOC) with random missing outputs. Since the traditional auxiliary model (AM) method is inefficient for systems with scarce measurements, this paper provides an IAKF-AM method. Compared with the AM method, the proposed method uses the measurable data to adjust missing outputs in each interval, thus has higher estimation accuracy. In addition, a recursive least squares (RLS) algorithm is introduced, which can combine the IAKF-AM method to iteratively estimate the SOC and outputs. In the simulation part, the mean absolute errors (MAE) and the root mean squared error (RMSE) is used to evaluate the model performance under different cases. Simulation example verify the effectiveness of the proposed IAKF-AM algorithm.
更多查看译文
关键词
Battery management system,State of charge,Recursive least squares algorithm,Second-order RC model,Kalman filter,Auxiliary model
AI 理解论文
溯源树
样例
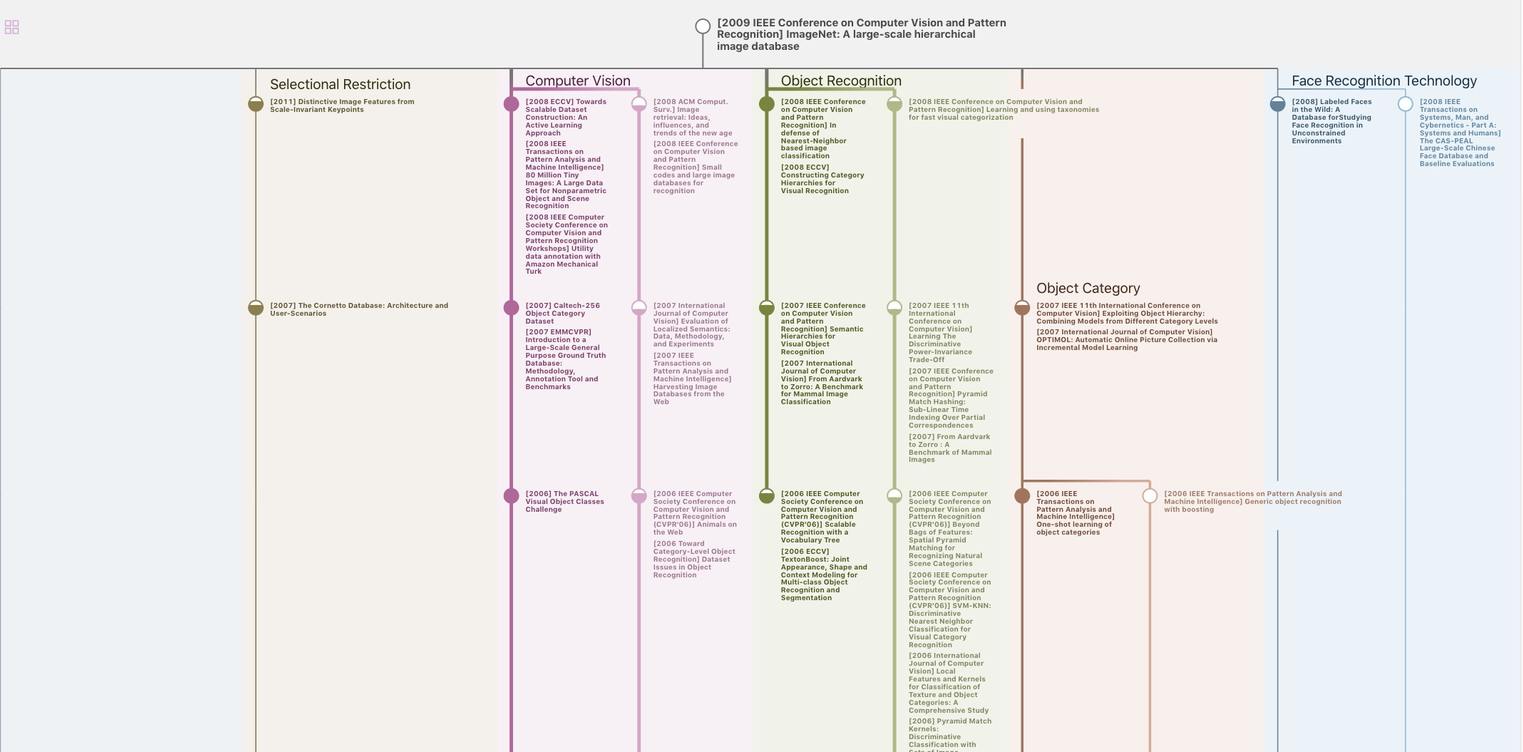
生成溯源树,研究论文发展脉络
Chat Paper
正在生成论文摘要