Identification of table grapes in the natural environment based on an improved Yolov5 and localization of picking points
PRECISION AGRICULTURE(2023)
摘要
Aiming at the difficulty in locating the picking points of table grapes in the natural environment, this paper proposed a Yolov5-CBAM-Fourth Detection Layer-Decoupled (Yolov5-CFD) network based on Yolov5 to realize grape and stem recognition. Meanwhile, the fast localization of the picking point was realized using a geometric method. First, to enhance the feature extraction ability of the Backbone module, it was improved by integrating the Convolutional Block Attention Module (CBAM) attention mechanism. Second, aiming at the problem that a small target was difficult to detect, the fourth layer of detection was added to the Neck module. In addition, the paper improved the Head module of Yolov5 by borrowing from Decoupled structure in Yolox, which optimized the classification and regression performance of the network. Further, a geometric method was used to quickly and accurately locate the picking points of table grapes. In order to verify the effectiveness of the proposed network model, about 10,000 grape images were used for training. The results showed that the detection precision, recall, mAP_0.5 and mAP_0.5:0.95 of the Yolov5s-CFD model were 0.857, 0.804, 0.855, 0.642, respectivaly. And the detection precision, recall, mAP_0.5 and mAP_0.5:0.95 of the Yolov5m-CFD model were 0.986, 0.987, 0.993 and 0.910, respectivaly. In addition, the success rate of picking point localization was compared with the corresponding network structure. The results showed that the success rate of picking point positioning of the Yolov5s-CFD model was increased by 11.53% compared with the initial Yolov5s, and the success rate of the Yolov5m-CFD model was increased by 5.84% compared with the initial Yolov5. Although the recognition time of the improved Yolov5 model is increased compared to the initial Yolov5 model, it is still acceptable. It can fully meet the requirements of mechanized picking of table grapes and provide a theoretical basis for mechanized picking of table grapes.
更多查看译文
关键词
Table grape,Picking point,Deep learning,Yolov5,Attention mechanism,Decoupled
AI 理解论文
溯源树
样例
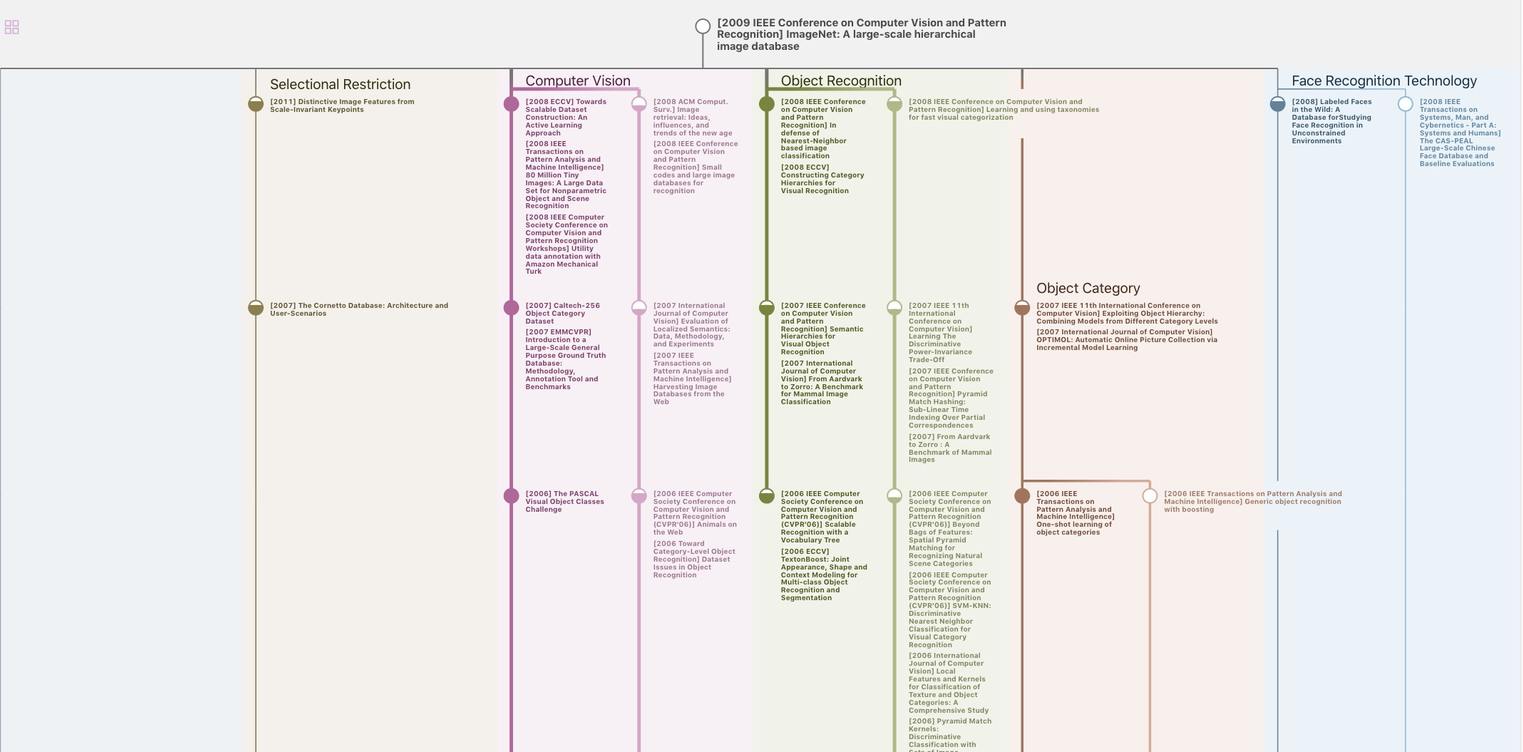
生成溯源树,研究论文发展脉络
Chat Paper
正在生成论文摘要