Min-Max Dynamic Programming Control for Systems with Uncertain Mathematical Models via Differential Neural Network Bellman's Function Approximation
MATHEMATICS(2023)
摘要
This research focuses on designing a min-max robust control based on a neural dynamic programming approach using a class of continuous differential neural networks (DNNs). The proposed controller solves the robust optimization of a proposed cost function that depends on the trajectories of a system with an uncertain mathematical model satisfying a class of non-linear perturbed systems. The dynamic programming min-max formulation enables robust control concerning bounded modelling uncertainties and disturbances. The Hamilton-Jacobi-Bellman (HJB) equation's value function, approximated by a DNN, permits to estimate the closed-loop formulation of the controller. The controller design is based on an estimated state trajectory with the worst possible uncertainties/perturbations that provide the degree of robustness using the proposed controller. The class of learning laws for the time-varying weights in the DNN is produced by studying the HJB partial differential equation. The controller uses the solution of the obtained learning laws and a time-varying Riccati equation. A recurrent algorithm based on the Kiefer-Wolfowitz method leads to adjusting the initial conditions for the weights to satisfy the final condition of the given cost function. The robust control suggested in this work is evaluated using a numerical example confirming the optimizing solution based on the DNN approximate for Bellman's value function.
更多查看译文
关键词
robust optimal control,artificial neural networks,approximate models,Kiefer-Wolfowitz method
AI 理解论文
溯源树
样例
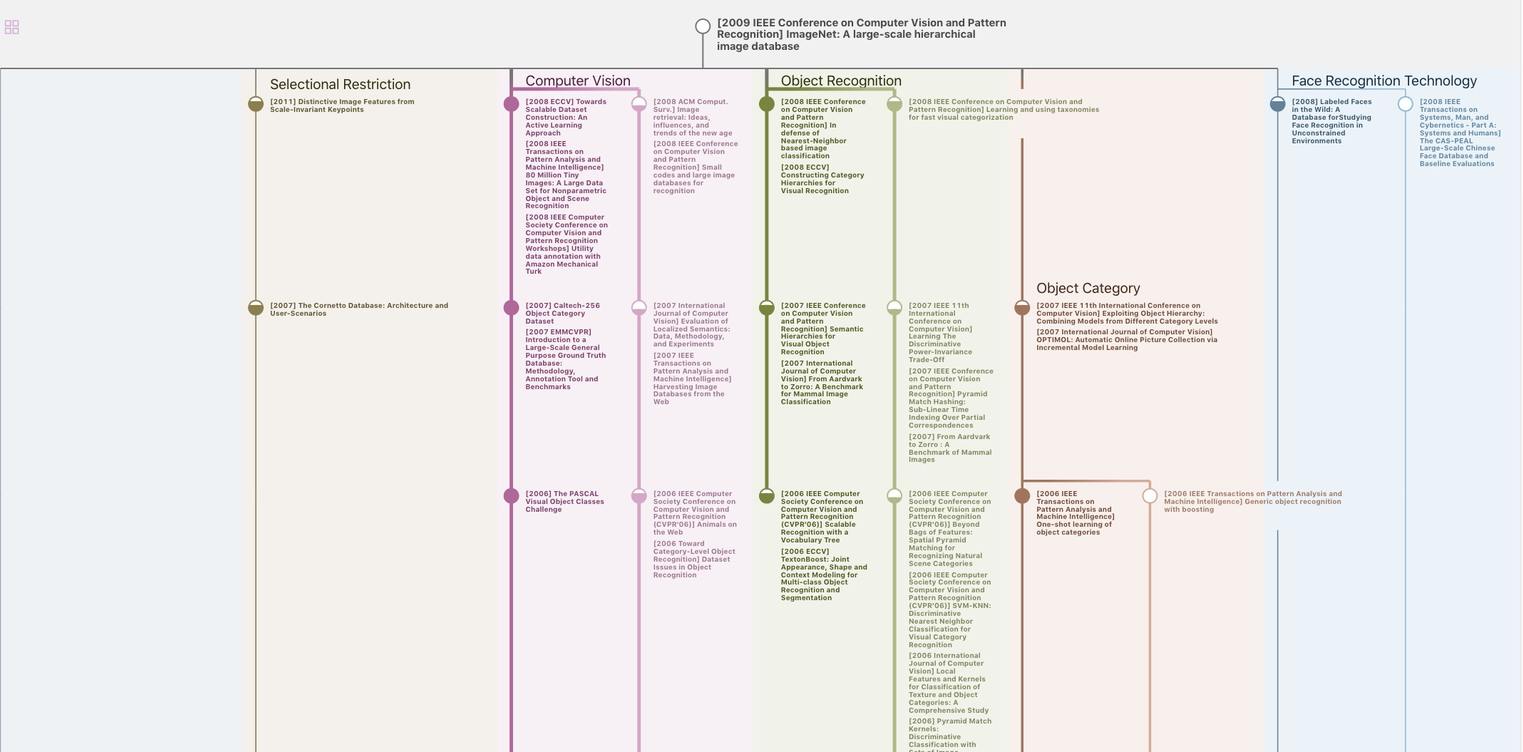
生成溯源树,研究论文发展脉络
Chat Paper
正在生成论文摘要