Towards Novel Statistical Methods for Anomaly Detection in Industrial Processes.
ICPE '23 Companion: Companion of the 2023 ACM/SPEC International Conference on Performance Engineering(2023)
摘要
This paper presents a novel methodology based on first principles of statistics and statistical learning for anomaly detection in industrial processes and IoT environments. We present a 5-level analytical pipeline that cleans, smooths, and eliminates redundancies from the data, and identifies outliers as well as the features that contribute most to these anomalies. We show how smoothing can make our methodology less sensitive to short-lived anomalies that might be, e.g., due to sensor noise. We validate the methodology on a dataset freely available in the literature. Our results show that we can identify all anomalies in the considered dataset, with the ability of controlling the amount of false positives. This work is the result of a research project co-funded by the Tuscany Region and a company leader in the paper and nonwovens sector. Although the methodology was developed for this domain, we consider here a dataset from a different industrial sector. This shows that our methodology can be generalized to other contexts with similar constraints on limited resources, interpretability, time, and budget.
更多查看译文
AI 理解论文
溯源树
样例
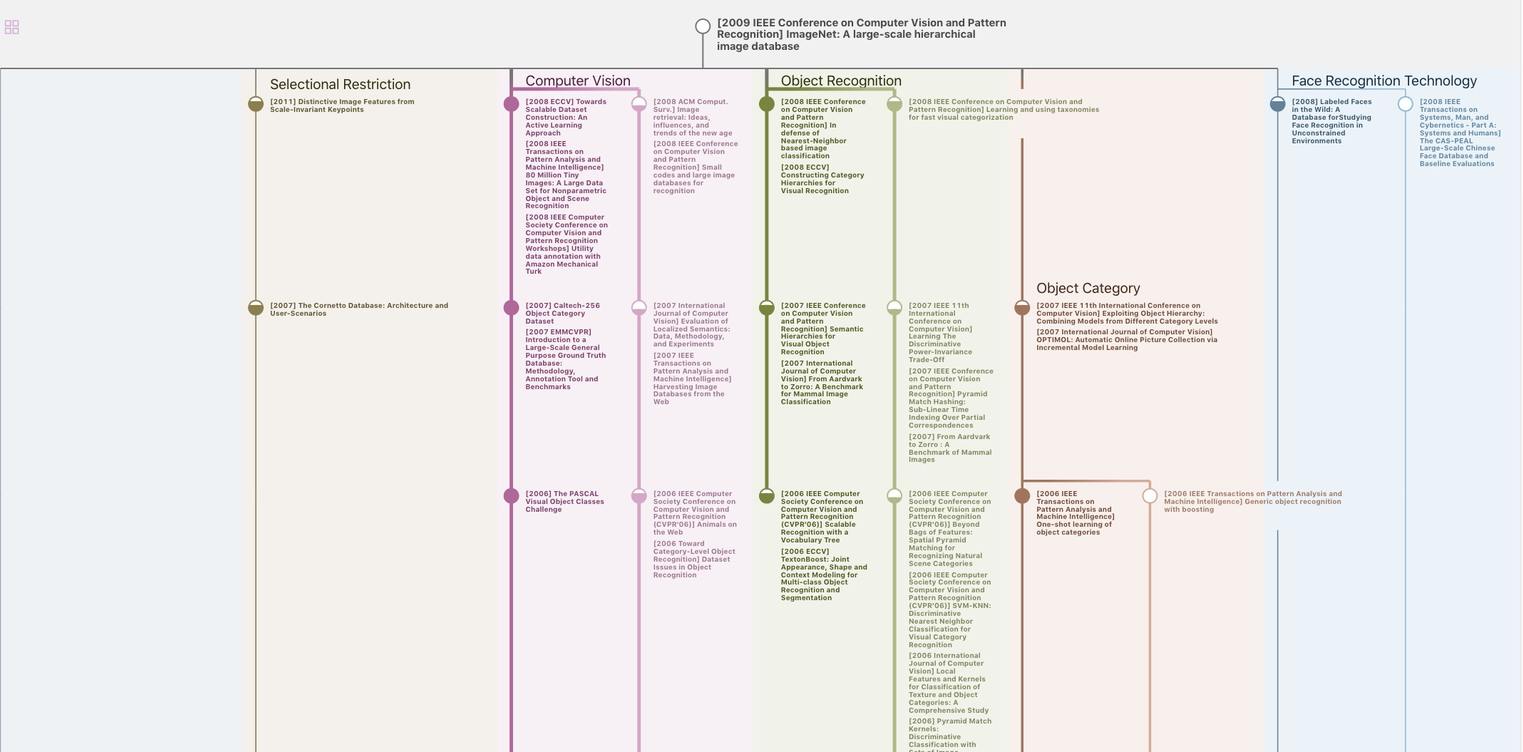
生成溯源树,研究论文发展脉络
Chat Paper
正在生成论文摘要