Query lower bounds for log-concave sampling
2023 IEEE 64TH ANNUAL SYMPOSIUM ON FOUNDATIONS OF COMPUTER SCIENCE, FOCS(2023)
摘要
Log-concave sampling has witnessed remarkable algorithmic advances in recent years, but the corresponding problem of proving lower bounds for this task has remained elusive, with lower bounds previously known only in dimension one. In this work, we establish the following query lower bounds: (1) sampling from strongly log-concave and log-smooth distributions in dimension d >= 2 requires Omega(log kappa) queries, which is sharp in any constant dimension, and (2) sampling from Gaussians in dimension d (hence also from general logconcave and log-smooth distributions in dimension d) requires (Omega) over tilde (min(root kappa log d, d)) queries, which is nearly sharp for the class of Gaussians. Here kappa denotes the condition number of the target distribution. Our proofs rely upon (1) a multiscale construction inspired by work on the Kakeya conjecture in geometric measure theory, and (2) a novel reduction that demonstrates that block Krylov algorithms are optimal for this problem, as well as connections to lower bound techniques based onWishart matrices developed in the matrix-vector query literature.
更多查看译文
关键词
Sampling,log-concave distributions,matrix-vector model,sublinear algorithms
AI 理解论文
溯源树
样例
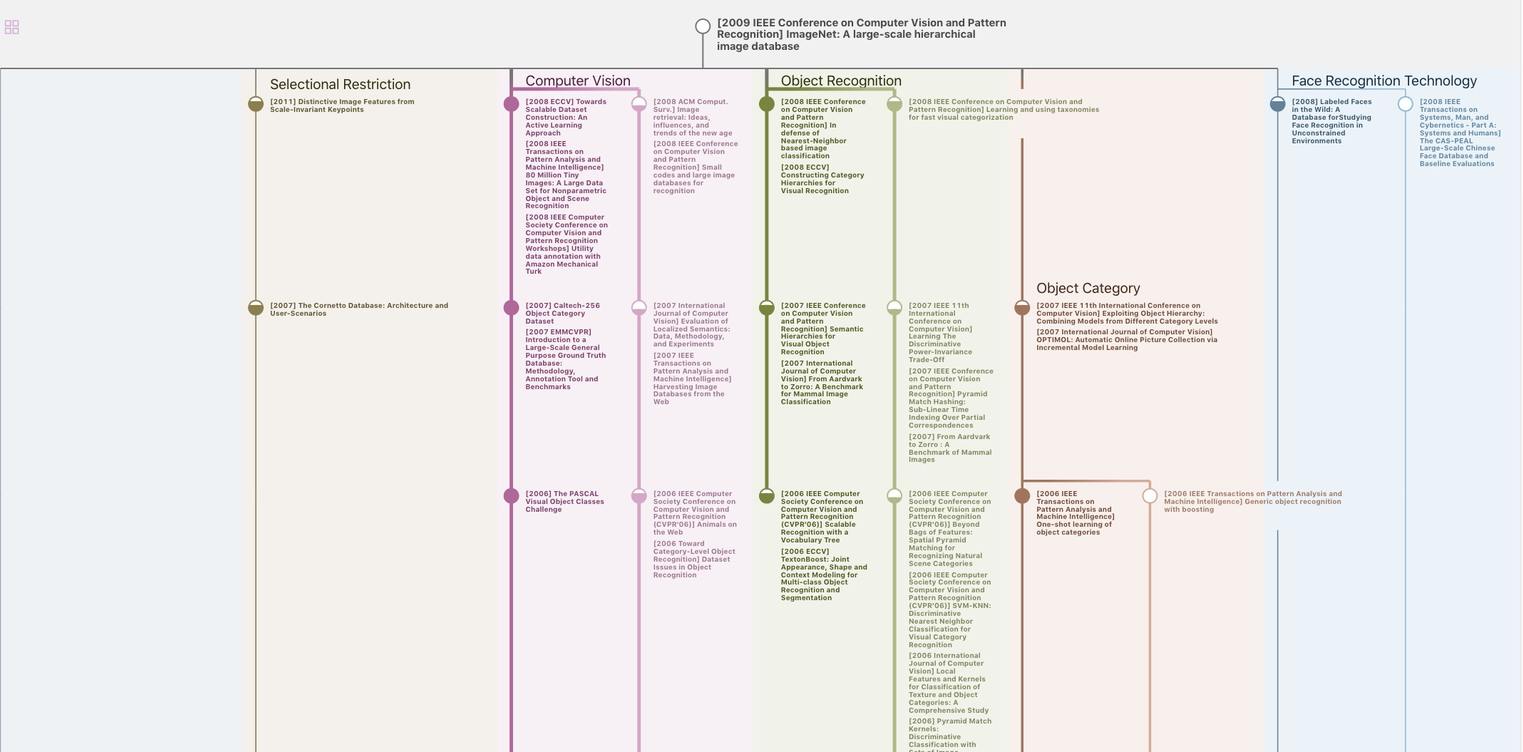
生成溯源树,研究论文发展脉络
Chat Paper
正在生成论文摘要