Inferring nonlinear fractional diffusion processes from single trajectories
New Journal of Physics(2023)
摘要
We present a method to infer the arbitrary space-dependent drift and diffusion of a nonlinear stochastic model driven by multiplicative fractional Gaussian noise from a single trajectory. Our method, fractional Onsager-Machlup optimisation (fOMo), introduces a maximum likelihood estimator by minimising a field-theoretic action which we construct from the observed time series. We successfully test fOMo for a wide range of Hurst exponents using artificial data with strong nonlinearities, and apply it to a data set of daily mean temperatures. We further highlight the significant systematic estimation errors when ignoring non-Markovianity, underlining the need for nonlinear fractional inference methods when studying real-world long-range (anti-)correlated systems.
更多查看译文
关键词
statistical inference,fractional Brownian motion,nonequilibrium statistical mechanics,maximum likelihood estimation,single-trajectory measurements,time series analysis,anomalous diffusion
AI 理解论文
溯源树
样例
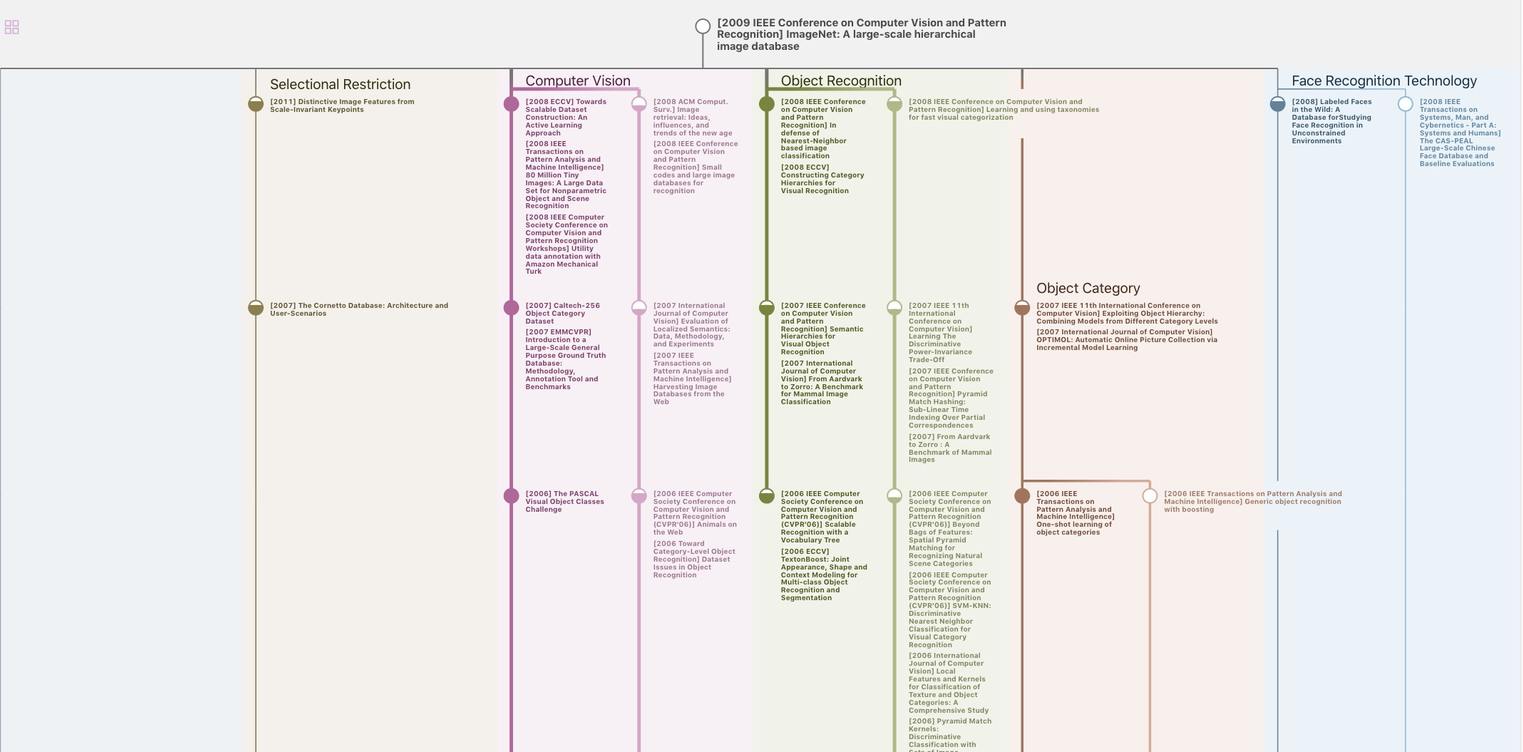
生成溯源树,研究论文发展脉络
Chat Paper
正在生成论文摘要