Both Efficiency and Effectiveness! A Large Scale Pre-ranking Framework in Search System
CoRR(2023)
摘要
In the realm of search systems, multi-stage cascade architecture is a prevalent method, typically consisting of sequential modules such as matching, pre-ranking, and ranking. It is generally acknowledged that the model used in the pre-ranking stage must strike a balance between efficacy and efficiency. Thus, the most commonly employed architecture is the representation-focused vector product based model. However, this architecture lacks effective interaction between the query and document, resulting in a reduction in the effectiveness of the search system. To address this issue, we present a novel pre-ranking framework called RankDFM. Our framework leverages DeepFM as the backbone and employs a pairwise training paradigm to learn the ranking of videos under a query. The capability of RankDFM to cross features provides significant improvement in offline and online A/B testing performance. Furthermore, we introduce a learnable feature selection scheme to optimize the model and reduce the time required for online inference, equivalent to a tree model. Currently, RankDFM has been deployed in the search system of a shortvideo App, providing daily services to hundreds of millions users.
更多查看译文
关键词
search,large scale,efficiency,effectiveness!,pre-ranking
AI 理解论文
溯源树
样例
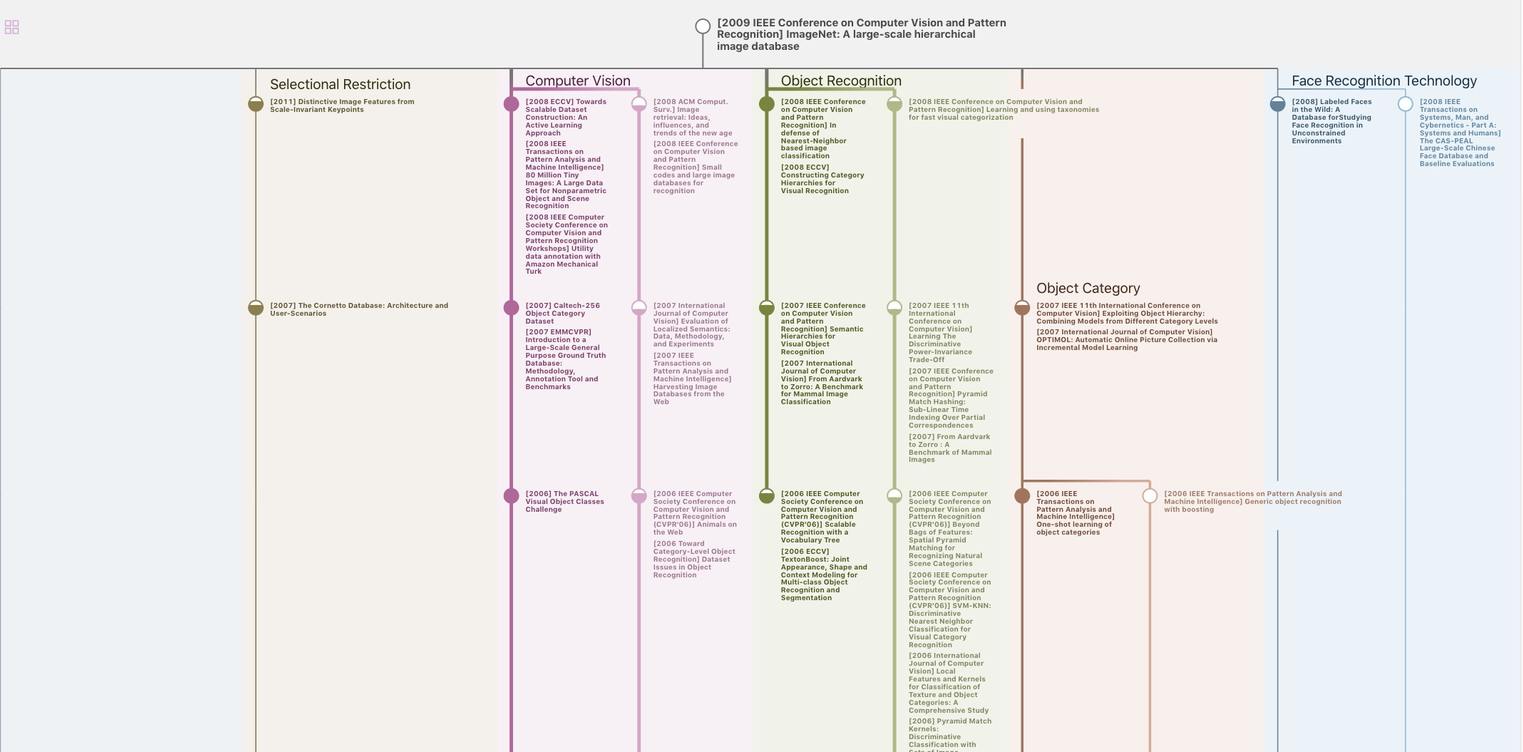
生成溯源树,研究论文发展脉络
Chat Paper
正在生成论文摘要