Learning earthquake sources using symmetric autoencoders
arxiv(2023)
摘要
We introduce Symmetric Autoencoder (SymAE), a neural-network architecture
designed to automatically isolate earthquake information from far-field seismic
waves. SymAE represents the measured displacement field using a code that is
partitioned into two interpretable components: source and path-scattering
information. We achieve this source-path representation using the scale
separation principle and stochastic regularization, which traditional
autoencoding methods lack. According to the scale separation principle, the
variations in far-field band-limited seismic measurements resulting from finite
faulting occur across two spatial scales: a slower scale associated with the
source processes and a faster scale corresponding to path effects. The primary
challenge in leveraging SymAE to enhance our understanding of earthquake
sources lies in the lack of direct interpretability of the latent code it
generates. However, despite this hurdle, we present two strategies. Firstly, we
employ clustering analysis of the source codes to reveal connections between
the characteristics of various earthquakes. This method is less susceptible to
variations in path effects, allowing us to assess the correlation in source
properties between observed earthquakes and their corresponding finite-fault
models. Secondly, we introduce the generation of virtual seismograms with
desired source and path information. Through this approach, we engineer
seismograms to incorporate the source characteristics of a specific earthquake
from different azimuths while maintaining consistent subsurface scattering
effects. We propose that these virtual seismograms may facilitate a
comprehensive and precise examination of directivity effects for the earthquake
in question. The paper conducts an analysis of nearly forty complex earthquake
events along with synthetic earthquakes.
更多查看译文
AI 理解论文
溯源树
样例
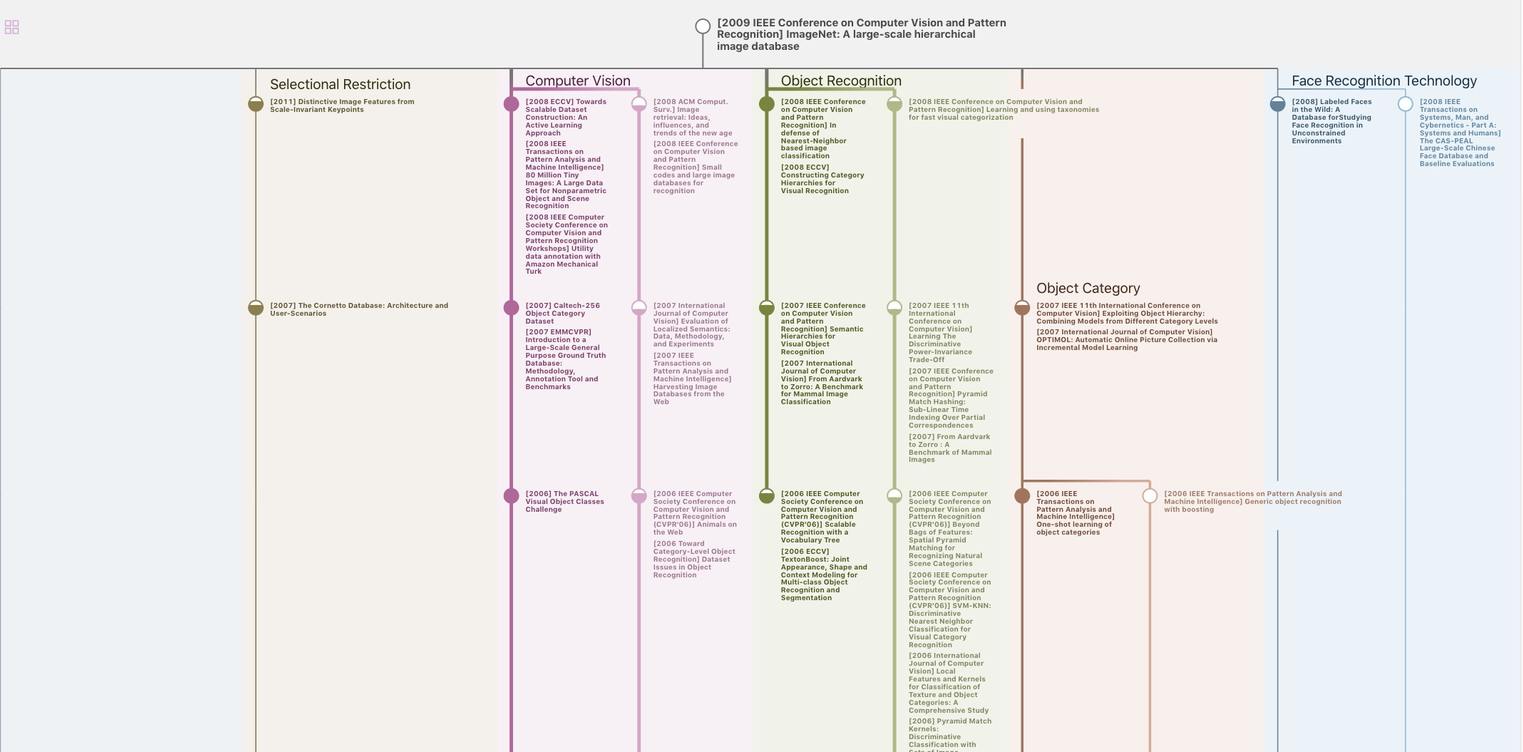
生成溯源树,研究论文发展脉络
Chat Paper
正在生成论文摘要