What's in a Name? Beyond Class Indices for Image Recognition
CoRR(2023)
摘要
Existing machine learning models demonstrate excellent performance in image object recognition after training on a large-scale dataset under full supervision. However, these models only learn to map an image to a predefined class index, without revealing the actual semantic meaning of the object in the image. In contrast, vision-language models like CLIP are able to assign semantic class names to unseen objects in a `zero-shot' manner, although they still rely on a predefined set of candidate names at test time. In this paper, we reconsider the recognition problem and task a vision-language model to assign class names to images given only a large and essentially unconstrained vocabulary of categories as prior information. We use non-parametric methods to establish relationships between images which allow the model to automatically narrow down the set of possible candidate names. Specifically, we propose iteratively clustering the data and voting on class names within them, showing that this enables a roughly 50\% improvement over the baseline on ImageNet. Furthermore, we tackle this problem both in unsupervised and partially supervised settings, as well as with a coarse-grained and fine-grained search space as the unconstrained dictionary.
更多查看译文
关键词
class indices,recognition,image
AI 理解论文
溯源树
样例
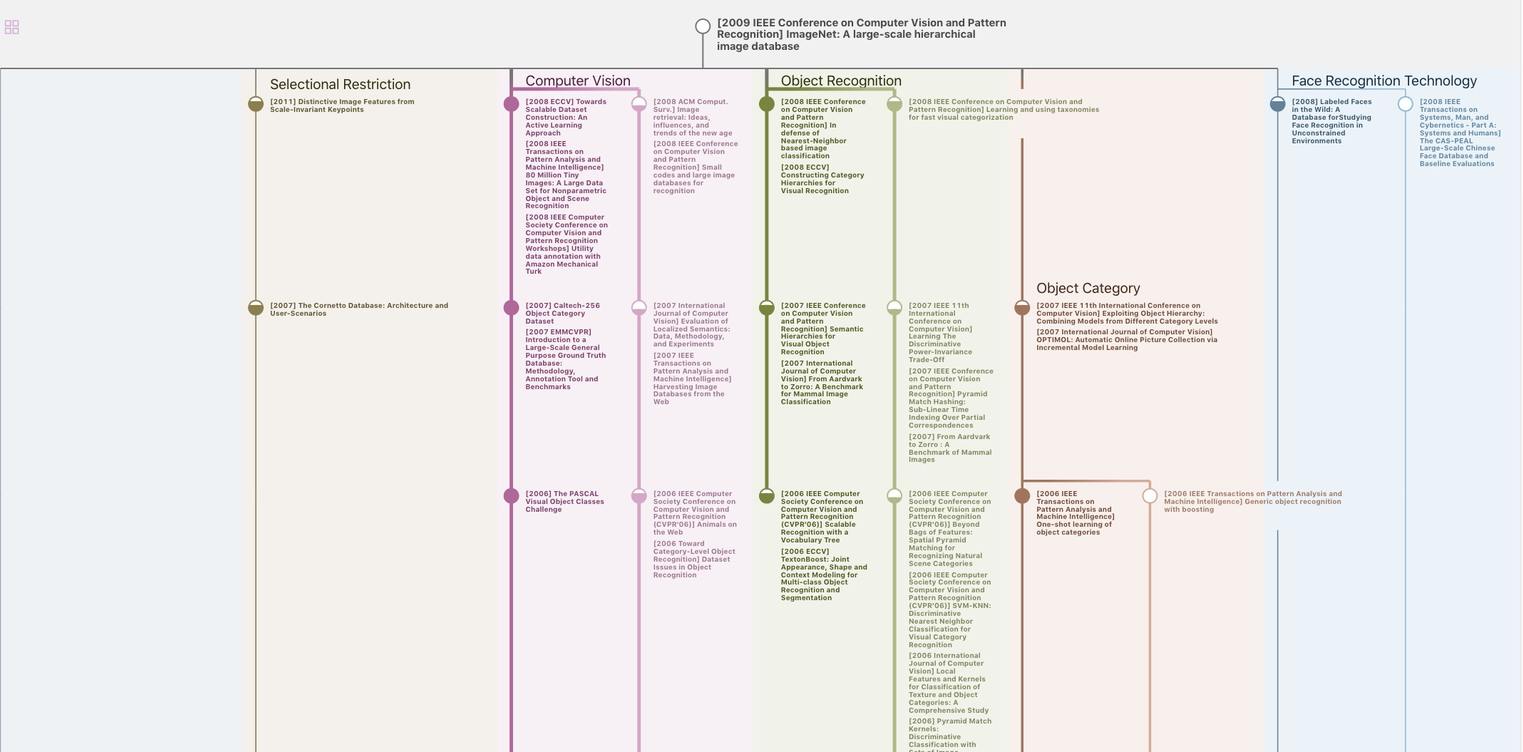
生成溯源树,研究论文发展脉络
Chat Paper
正在生成论文摘要