Towards Self-Explainability of Deep Neural Networks with Heatmap Captioning and Large-Language Models
CoRR(2023)
摘要
Heatmaps are widely used to interpret deep neural networks, particularly for computer vision tasks, and the heatmap-based explainable AI (XAI) techniques are a well-researched topic. However, most studies concentrate on enhancing the quality of the generated heatmap or discovering alternate heatmap generation techniques, and little effort has been devoted to making heatmap-based XAI automatic, interactive, scalable, and accessible. To address this gap, we propose a framework that includes two modules: (1) context modelling and (2) reasoning. We proposed a template-based image captioning approach for context modelling to create text-based contextual information from the heatmap and input data. The reasoning module leverages a large language model to provide explanations in combination with specialised knowledge. Our qualitative experiments demonstrate the effectiveness of our framework and heatmap captioning approach. The code for the proposed template-based heatmap captioning approach will be publicly available.
更多查看译文
关键词
captioning,deep neural networks,neural networks,self-explainability,large-language
AI 理解论文
溯源树
样例
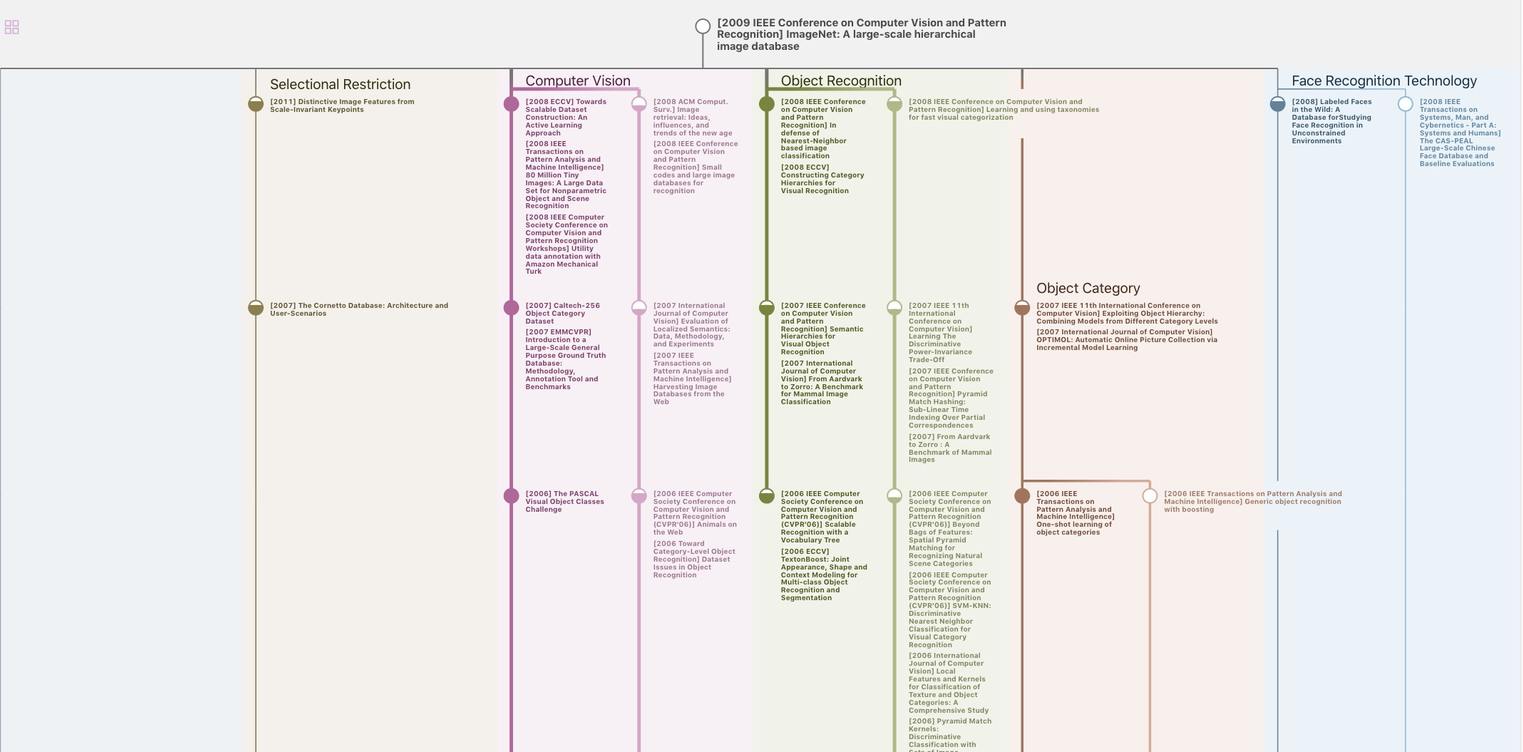
生成溯源树,研究论文发展脉络
Chat Paper
正在生成论文摘要