Paᗧ-HuBERT: Self-Supervised Music Source Separation Via Primitive Auditory Clustering And Hidden-Unit Bert
CoRR(2023)
摘要
In spite of the progress in music source separation research, the small amount of publicly-available clean source data remains a constant limiting factor for performance. Thus, recent advances in self-supervised learning present a largely-unexplored opportunity for improving separation models by leveraging unlabelled music data. In this paper, we propose a self-supervised learning framework for music source separation inspired by the HuBERT speech representation model. We first investigate the potential impact of the original HuBERT model by inserting an adapted version of it into the well-known Demucs V2 time-domain separation architecture. We then propose Paᗧ-HuBERT, a time-frequency-domain self-supervised model, that we later use in combination with a ResU-Net decoder for source separation. Paᗧ-HuBERT uses primitive auditory features of music as unsupervised clustering labels to initialize the self-supervised pretraining process using the Free Music Archive (FMA) dataset. The resulting framework achieves better source-to-distortion ratio (SDR) performance on the MusDB18 test set than the original Demucs V2 and Res-U-Net models. We further demonstrate that it can boost performance with small amounts of supervised data. Ultimately, our proposed framework is an effective solution to the challenge of limited clean source data for music source separation.
更多查看译文
关键词
Music source separation,primitive auditory principles,self-supervised Learning,BERT
AI 理解论文
溯源树
样例
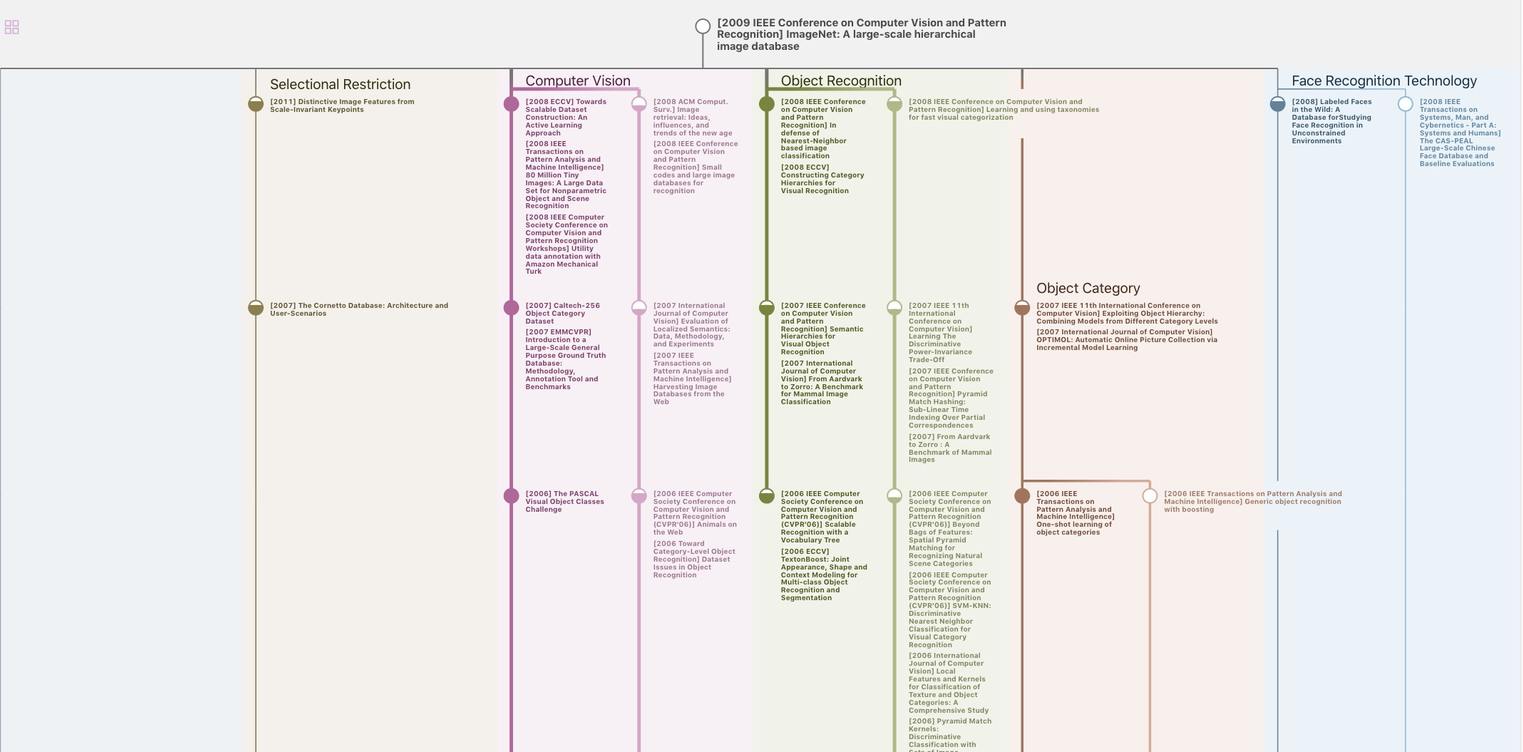
生成溯源树,研究论文发展脉络
Chat Paper
正在生成论文摘要