Abstract 3150: Computational cohort discovery and coordinator-led validation to navigate potential research candidates to clinical trials
Cancer Research(2023)
摘要
Abstract The purpose of this study was to improve the identification and solicitation of human subject candidates to augment patient enrollment to clinical trials. In the past, our enrollment efforts involved manual mechanisms that began at the time that cancer patients were presented for care, most commonly at our chemotherapy clinics. We reasoned that computational review of pathology records may be far more efficient, allowing us to screen larger numbers of patients, and may allow us to intercept patients at the time of diagnosis, rather than time of care. We thus implemented a new workflow: (1) computational query of pathology database to filter candidates based on key eligibility criteria; (2) validation of the query using a clinical research coordinator, who can then (3) navigate the patient to the trial. As a proof of concept, starting in August of 2022, we selected two gastrointestinal oncology trials, the COBRA and PACES trial, based on trial eligibility that could be easily extracted using pathologic criteria, and because these trials had low enrollment. We designed computational queries to extract cohorts of potential patients whose surgery dates and pathology staging identified potential clinical trial candidates. A coordinator performed chart-review to validate these candidates. The trial coordinator then contacted the patient’s surgical oncologist, facilitated referral to a medical oncologist, and tracked the number of patients approached and consented. Whereas in a 12-month period preceding this new workflow, we had 0 and 1 patients approached for the PACES and COBRA trial, in the 3-month period following the new intervention, we had 8 and 2 patients approached. These data suggest computational cohort building and subsequent navigation have the potential to improve enrollment onto clinical trials and motivate extension of this work to additional trials. Table(s) Trial # of patients approached pre-intervention Computational Criteria Meeting computational criteria Coordinator validation criteria # of patients validated by coordinator # of patients connected with medical oncologist # of patients approached post-intervention PACES 0 Colorectal cancer, stage T0-T3,N0-1 Date Aug 2021 – July 2022 23 Facility location, No evidence of disease on colonoscopy performed 120 -456 days post-surgery, No chemotherapy, No other malignancy 18 8 8 COBRA 1 Colorectal cancer pT3N0resection date August 2022 – October 2022 18 ≥ 12 lymph nodes assessedPatient locationIf the subject appropriate for active surveillanceSurgery within 14-60 days of randomization 4 0 2 Citation Format: Yonah Ziemba, Kaitlin Parnahay, Paul Ward, Nalan Yurtsever, Sharon Fox, Cheryl Schleicher, Priya Singh-Shiwsankar, Vincent Vinciguerra, Joseph Herman, James M. Crawford, Daniel A. King. Computational cohort discovery and coordinator-led validation to navigate potential research candidates to clinical trials [abstract]. In: Proceedings of the American Association for Cancer Research Annual Meeting 2023; Part 1 (Regular and Invited Abstracts); 2023 Apr 14-19; Orlando, FL. Philadelphia (PA): AACR; Cancer Res 2023;83(7_Suppl):Abstract nr 3150.
更多查看译文
关键词
computational cohort discovery,clinical trials,potential research candidates,coordinator-led
AI 理解论文
溯源树
样例
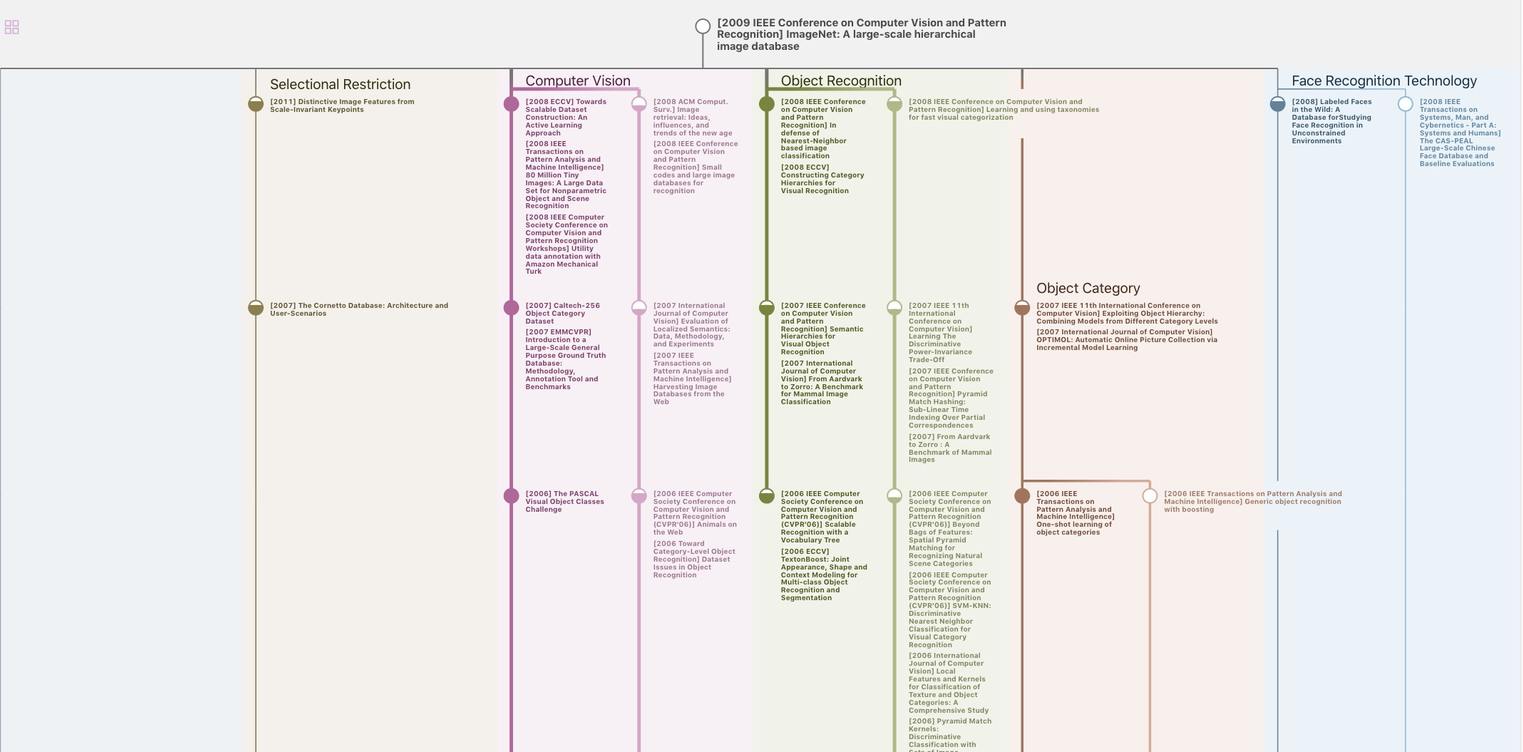
生成溯源树,研究论文发展脉络
Chat Paper
正在生成论文摘要