Model-corrected learned primal-dual models for fast limited-view photoacoustic tomography
CoRR(2023)
摘要
Learned iterative reconstructions hold great promise to accelerate tomographic imaging with empirical robustness to model perturbations. Nevertheless, an adoption for photoacoustic tomography is hindered by the need to repeatedly evaluate the computational expensive forward model. Computational feasibility can be obtained by the use of fast approximate models, but a need to compensate model errors arises. In this work we advance the methodological and theoretical basis for model corrections in learned image reconstructions by embedding the model correction in a learned primal-dual framework. Here, the model correction is jointly learned in data space coupled with a learned updating operator in image space within an unrolled end-to-end learned iterative reconstruction approach. The proposed formulation allows an extension to a primal-dual deep equilibrium model providing fixed-point convergence as well as reduced memory requirements for training. We provide theoretical and empirical insights into the proposed models with numerical validation in a realistic 2D limited-view setting. The model-corrected learned primal-dual methods show excellent reconstruction quality with fast inference times and thus providing a methodological basis for real-time capable and scalable iterative reconstructions in photoacoustic tomography.
更多查看译文
关键词
tomography,models,model-corrected,primal-dual,limited-view
AI 理解论文
溯源树
样例
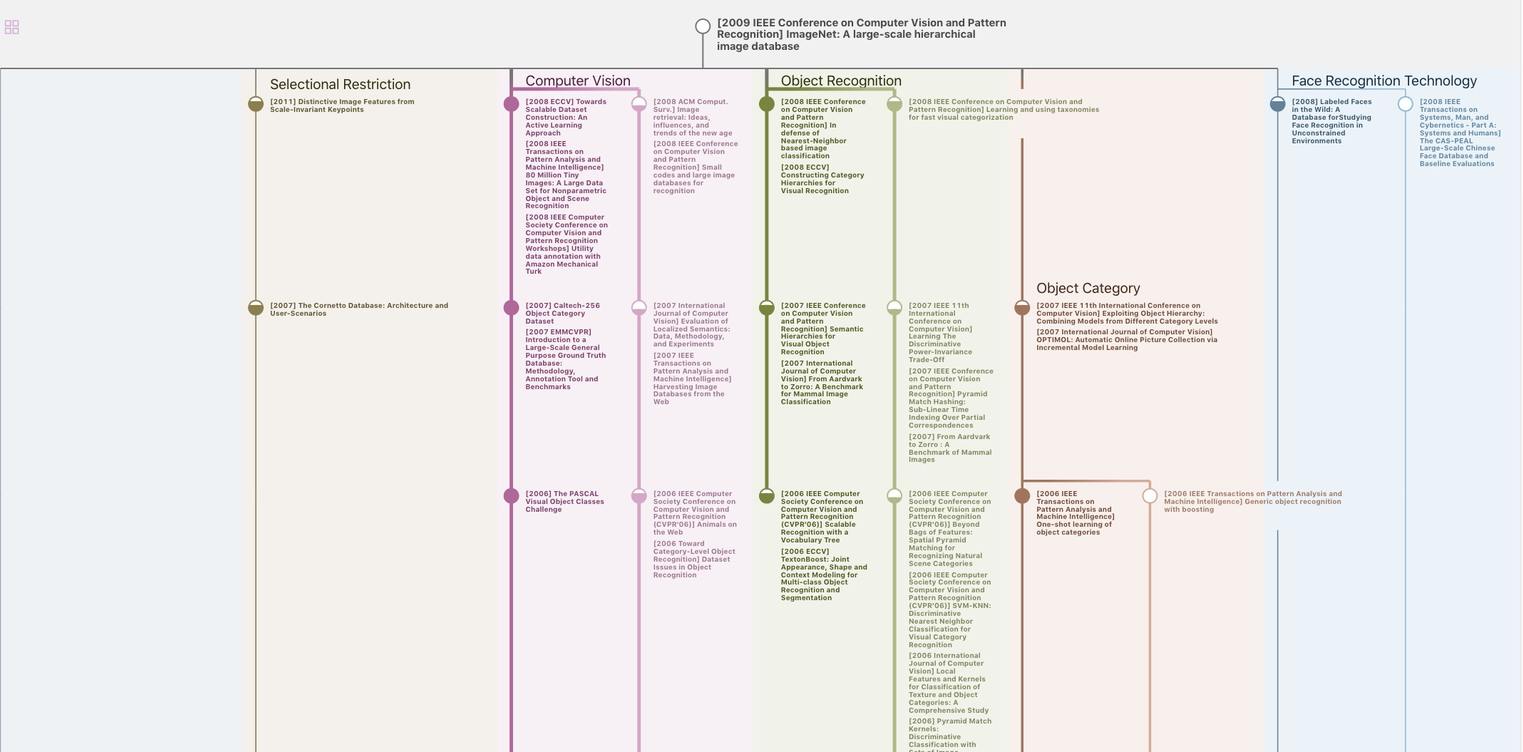
生成溯源树,研究论文发展脉络
Chat Paper
正在生成论文摘要