Learning quantities of interest from parametric PDEs: An efficient neural-weighted Minimal Residual approach
Computers & Mathematics with Applications(2024)
摘要
The efficient approximation of parametric PDEs is of tremendous importance in science and engineering. In this paper, we show how one can train Galerkin discretizations to efficiently learn quantities of interest of solutions to a parametric PDE. The central component in our approach is an efficient neural-network-weighted Minimal-Residual formulation, which, after training, provides Galerkin-based approximations in standard discrete spaces that have accurate quantities of interest, regardless of the coarseness of the discrete space.
更多查看译文
关键词
Parametric PDEs,Artificial neural networks,Weighted inner-products,Goal-oriented finite elements,Residual minimization
AI 理解论文
溯源树
样例
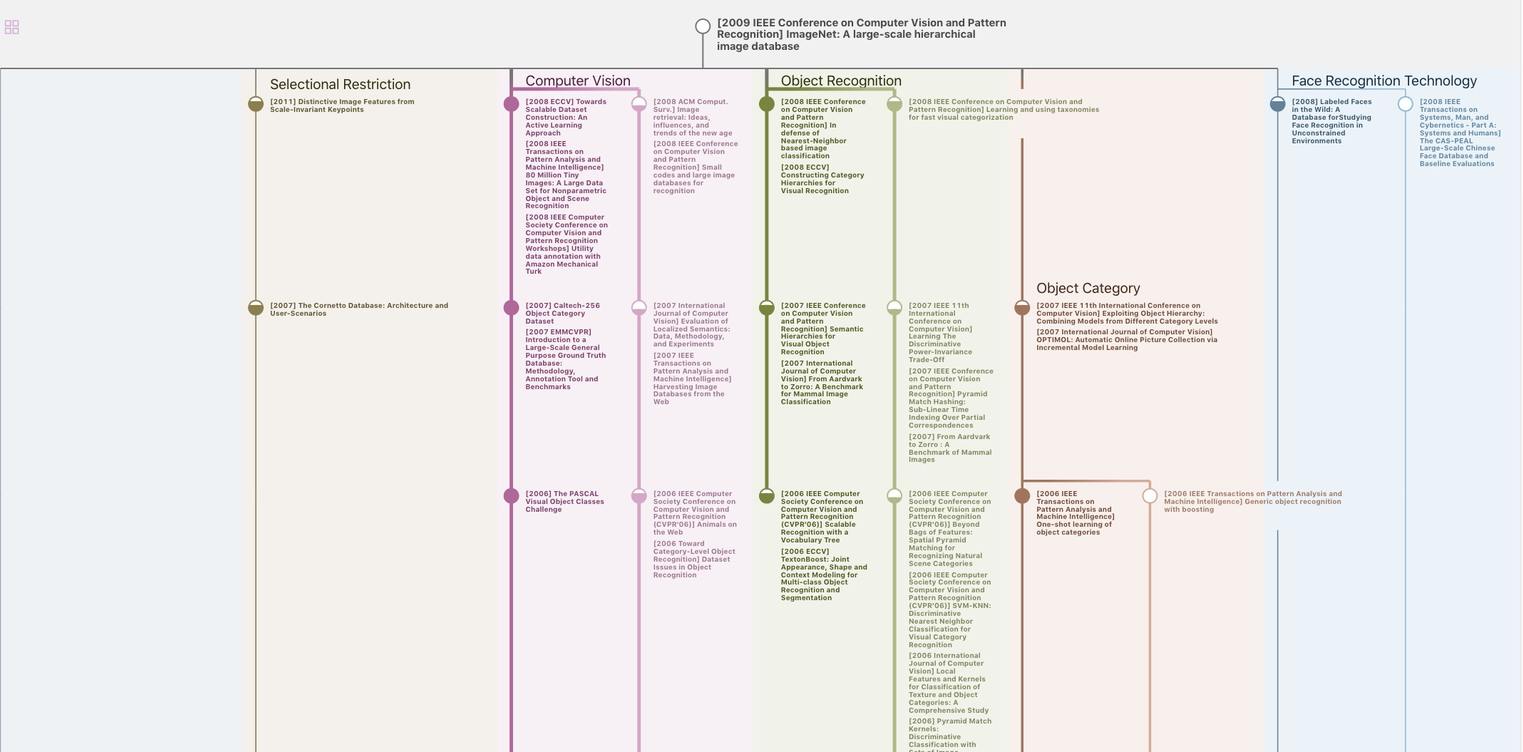
生成溯源树,研究论文发展脉络
Chat Paper
正在生成论文摘要