SimCSum: Joint Learning of Simplification and Cross-lingual Summarization for Cross-lingual Science Journalism
CoRR(2023)
摘要
Cross-lingual science journalism generates popular science stories of scientific articles different from the source language for a non-expert audience. Hence, a cross-lingual popular summary must contain the salient content of the input document, and the content should be coherent, comprehensible, and in a local language for the targeted audience. We improve these aspects of cross-lingual summary generation by joint training of two high-level NLP tasks, simplification and cross-lingual summarization. The former task reduces linguistic complexity, and the latter focuses on cross-lingual abstractive summarization. We propose a novel multi-task architecture - SimCSum consisting of one shared encoder and two parallel decoders jointly learning simplification and cross-lingual summarization. We empirically investigate the performance of SimCSum by comparing it with several strong baselines over several evaluation metrics and by human evaluation. Overall, SimCSum demonstrates statistically significant improvements over the state-of-the-art on two non-synthetic cross-lingual scientific datasets. Furthermore, we conduct an in-depth investigation into the linguistic properties of generated summaries and an error analysis.
更多查看译文
关键词
summarization,journalism,simplification,simcsum,joint learning,cross-lingual,cross-lingual
AI 理解论文
溯源树
样例
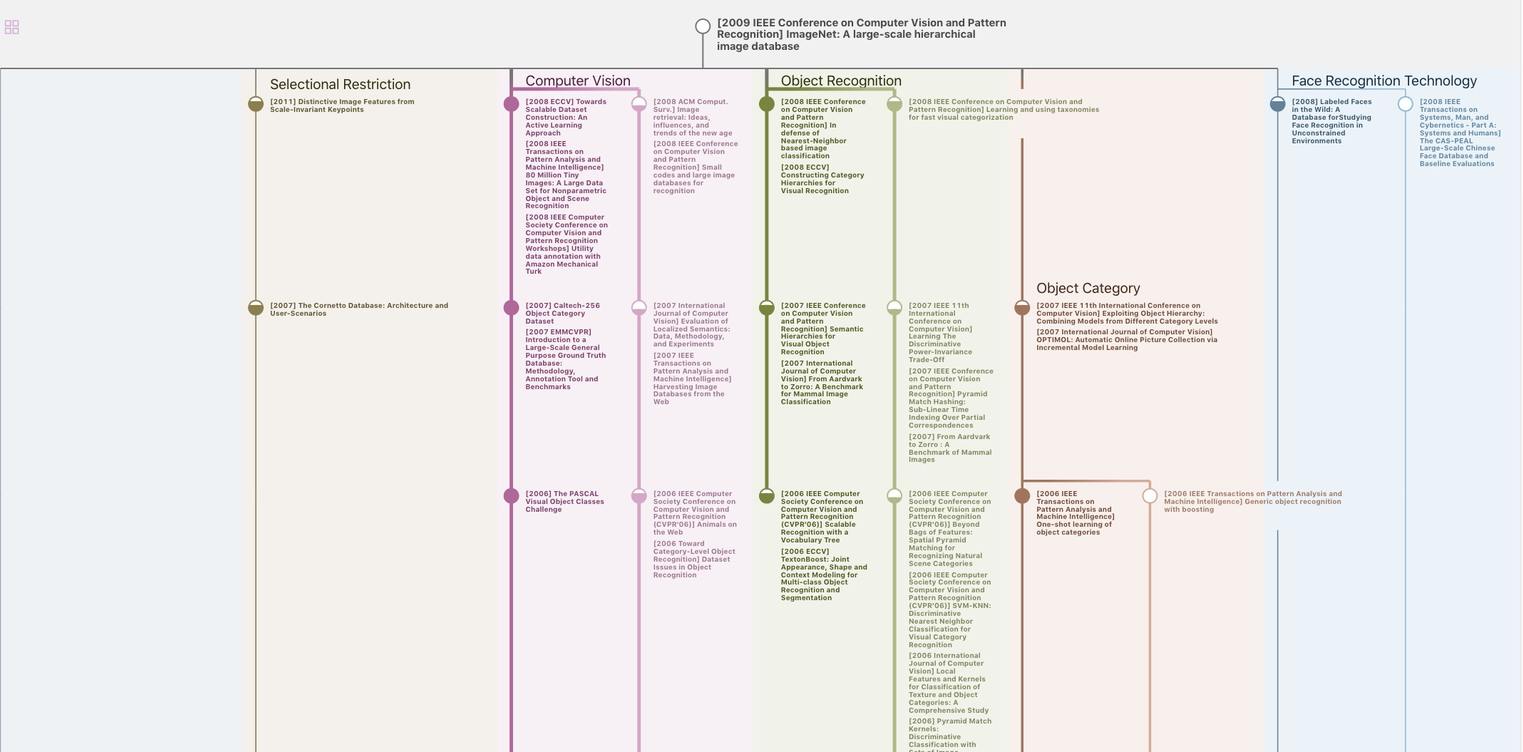
生成溯源树,研究论文发展脉络
Chat Paper
正在生成论文摘要