Online Distillation with Continual Learning for Cyclic Domain Shifts
CoRR(2023)
摘要
In recent years, online distillation has emerged as a powerful technique for adapting real-time deep neural networks on the fly using a slow, but accurate teacher model. However, a major challenge in online distillation is catastrophic forgetting when the domain shifts, which occurs when the student model is updated with data from the new domain and forgets previously learned knowledge. In this paper, we propose a solution to this issue by leveraging the power of continual learning methods to reduce the impact of domain shifts. Specifically, we integrate several state-of-the-art continual learning methods in the context of online distillation and demonstrate their effectiveness in reducing catastrophic forgetting. Furthermore, we provide a detailed analysis of our proposed solution in the case of cyclic domain shifts. Our experimental results demonstrate the efficacy of our approach in improving the robustness and accuracy of online distillation, with potential applications in domains such as video surveillance or autonomous driving. Overall, our work represents an important step forward in the field of online distillation and continual learning, with the potential to significantly impact real-world applications.
更多查看译文
关键词
online distillation,continual learning
AI 理解论文
溯源树
样例
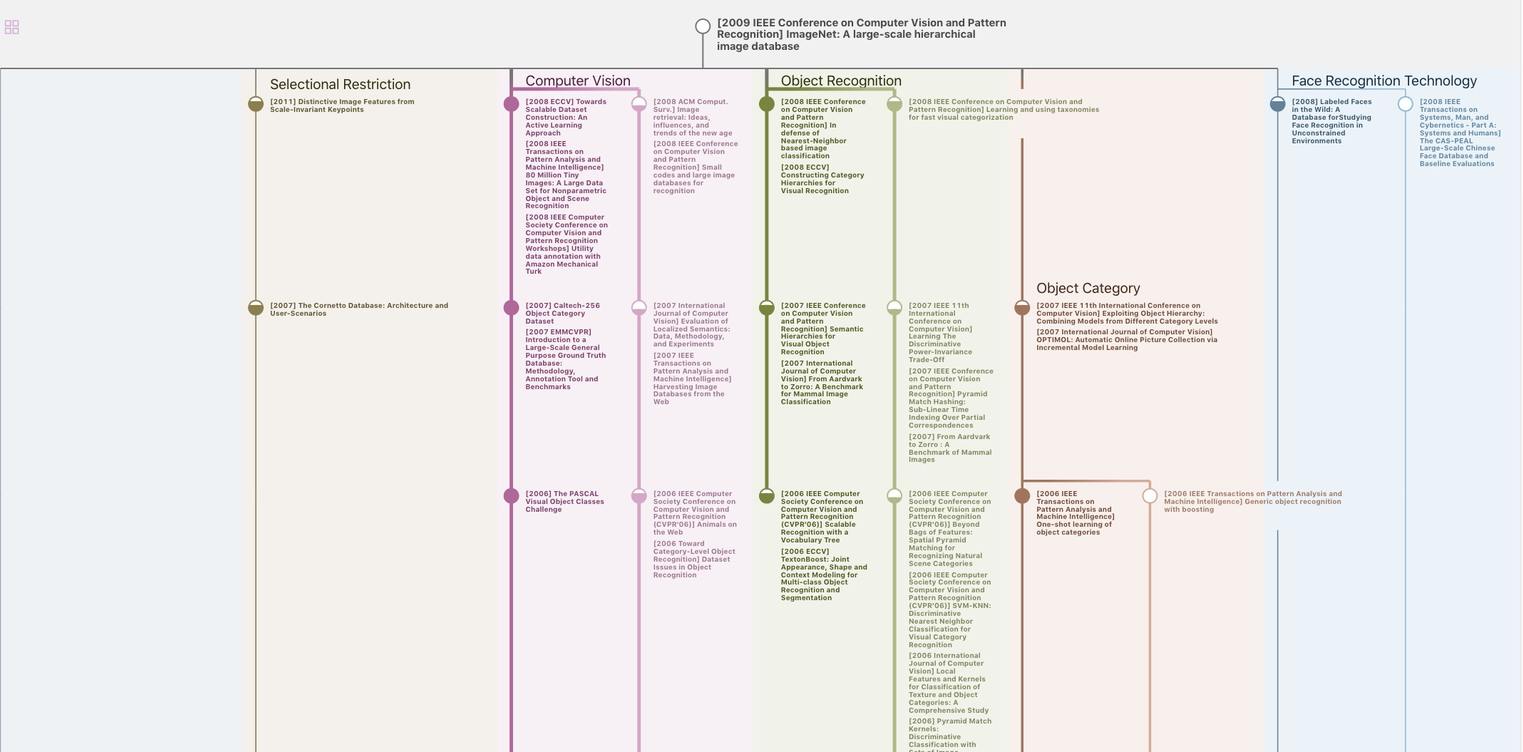
生成溯源树,研究论文发展脉络
Chat Paper
正在生成论文摘要