Pushing the limits of low-cost ultralow-field MRI by dual-acquisition deep learning 3D superresolution
Magnetic resonance in medicine(2023)
摘要
PurposeRecent development of ultralow-field (ULF) MRI presents opportunities for low-power, shielding-free, and portable clinical applications at a fraction of the cost. However, its performance remains limited by poor image quality. Here, a computational approach is formulated to advance ULF MR brain imaging through deep learning of large-scale publicly available 3T brain data. MethodsA dual-acquisition 3D superresolution model is developed for ULF brain MRI at 0.055 T. It consists of deep cross-scale feature extraction, attentional fusion of two acquisitions, and reconstruction. Models for T-1-weighted and T-2-weighted imaging were trained with 3D ULF image data sets synthesized from the high-resolution 3T brain data from the Human Connectome Project. They were applied to 0.055T brain MRI with two repetitions and isotropic 3-mm acquisition resolution in healthy volunteers, young and old, as well as patients. ResultsThe proposed approach significantly enhanced image spatial resolution and suppressed noise/artifacts. It yielded high 3D image quality at 0.055 T for the two most common neuroimaging protocols with isotropic 1.5-mm synthetic resolution and total scan time under 20 min. Fine anatomical details were restored with intrasubject reproducibility, intercontrast consistency, and confirmed by 3T MRI. ConclusionThe proposed dual-acquisition 3D supe-resolution approach advances ULF MRI for quality brain imaging through deep learning of high-field brain data. Such strategy can empower ULF MRI for low-cost brain imaging, especially in point-of-care scenarios or/and in low-income and mid-income countries.
更多查看译文
关键词
3D superresolution,brain,deep learning,MRI,ultralow-field MRI
AI 理解论文
溯源树
样例
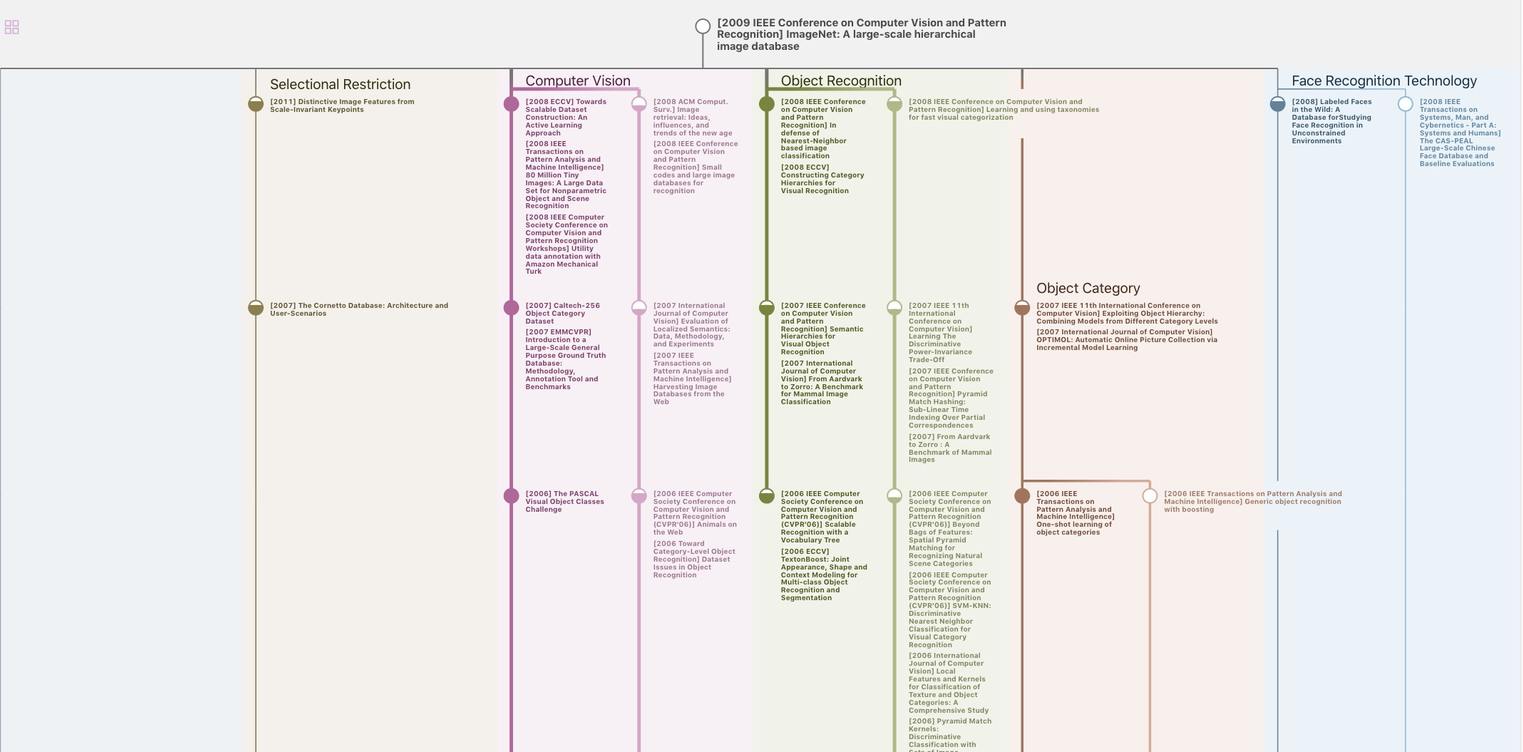
生成溯源树,研究论文发展脉络
Chat Paper
正在生成论文摘要