Iterative transfer learning for automatic collective motion tuning on multiple robot platforms
FRONTIERS IN NEUROROBOTICS(2023)
摘要
This paper proposes an iterative transfer learning approach to achieve swarming collective motion in groups of mobile robots. By applying transfer learning, a deep learner capable of recognizing swarming collective motion can use its knowledge to tune stable collective motion behaviors across multiple robot platforms. The transfer learner requires only a small set of initial training data from each robot platform, and this data can be collected from random movements. The transfer learner then progressively updates its own knowledge base with an iterative approach. This transfer learning eliminates the cost of extensive training data collection and the risk of trial-and-error learning on robot hardware. We test this approach on two robot platforms: simulated Pioneer 3DX robots and real Sphero BOLT robots. The transfer learning approach enables both platforms to automatically tune stable collective behaviors. Using the knowledge-base library the tuning procedure is fast and accurate. We demonstrate that these tuned behaviors can be used for typical multi-robot tasks such as coverage, even though they are not specifically designed for coverage tasks.
更多查看译文
关键词
automatic collective motion,transfer learning,iterative transfer,robot
AI 理解论文
溯源树
样例
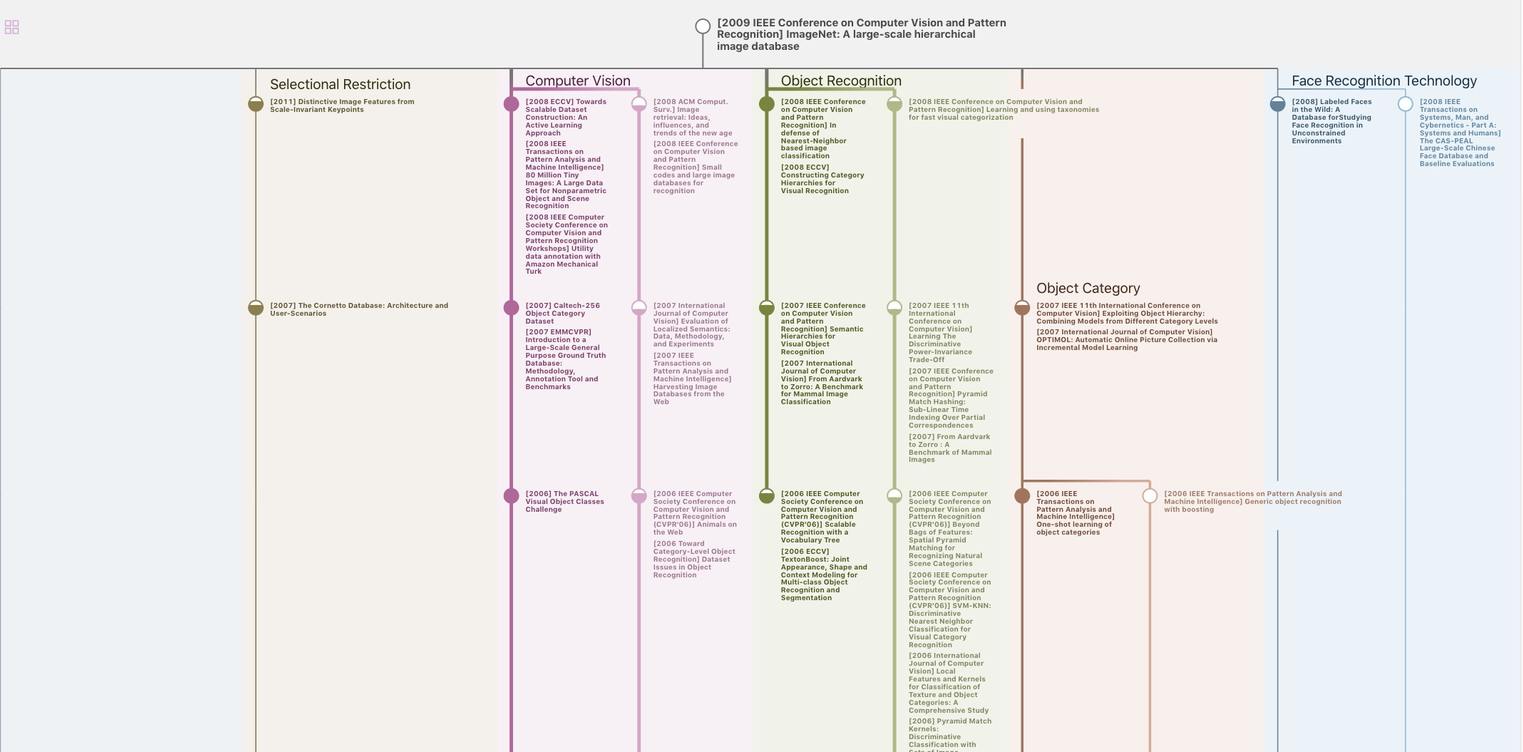
生成溯源树,研究论文发展脉络
Chat Paper
正在生成论文摘要