Gaussian Anamorphosis for Ensemble Kalman Filter Analysis of SAR-Derived Wet Surface Ratio Observations
IEEE TRANSACTIONS ON GEOSCIENCE AND REMOTE SENSING(2024)
摘要
Flood simulation and forecast capability have been greatly improved thanks to advances in data assimilation (DA) strategies incorporating various types of observations; many are derived from Earth Observations from space. This article focuses on the assimilation of 2-D flood observations derived from synthetic aperture radar (SAR) images acquired during a flood event with a dual state-parameter ensemble Kalman filter (EnKF). Resulting binary wet/dry maps are here expressed in terms of wet surface ratios (WSRs) over a number of subdomains of the floodplain. This ratio is assimilated jointly with in situ water-level observations to improve the flow dynamics within the floodplain. However, the non-Gaussianity of the observation errors associated with these SAR-derived measurements breaks a major hypothesis for the application of the EnKF, thus jeopardizing the optimality of the filter analysis. The novelty of this article lies in the treatment of the non-Gaussianity of the SAR-derived WSR observations with a Gaussian anamorphosis (GA) process. This DA strategy was validated and applied over the Garonne Marmandaise catchment (southwest of France) represented with a TELEMAC-2D hydrodynamic model, first in a twin experiment and then for a major flood event that occurred in January and February 2021. It was shown that assimilating SAR-derived WSR observations in complement to the in situ water-level observations significantly improves the representation of the flood dynamics. The GA process brings further improvement to the DA analysis while also demonstrating to be a nonessential element. This study heralds a reliable solution for flood forecasting over poorly gauged catchments thanks to available remote sensing datasets.
更多查看译文
关键词
Anamorphosis,data assimilation (DA),ensemble Kalman filter (EnKF),flooding,Gaussianity,hydrodynamics,remote sensing (RS),Sentinel-1 (S1),synthetic aperture radar (SAR)
AI 理解论文
溯源树
样例
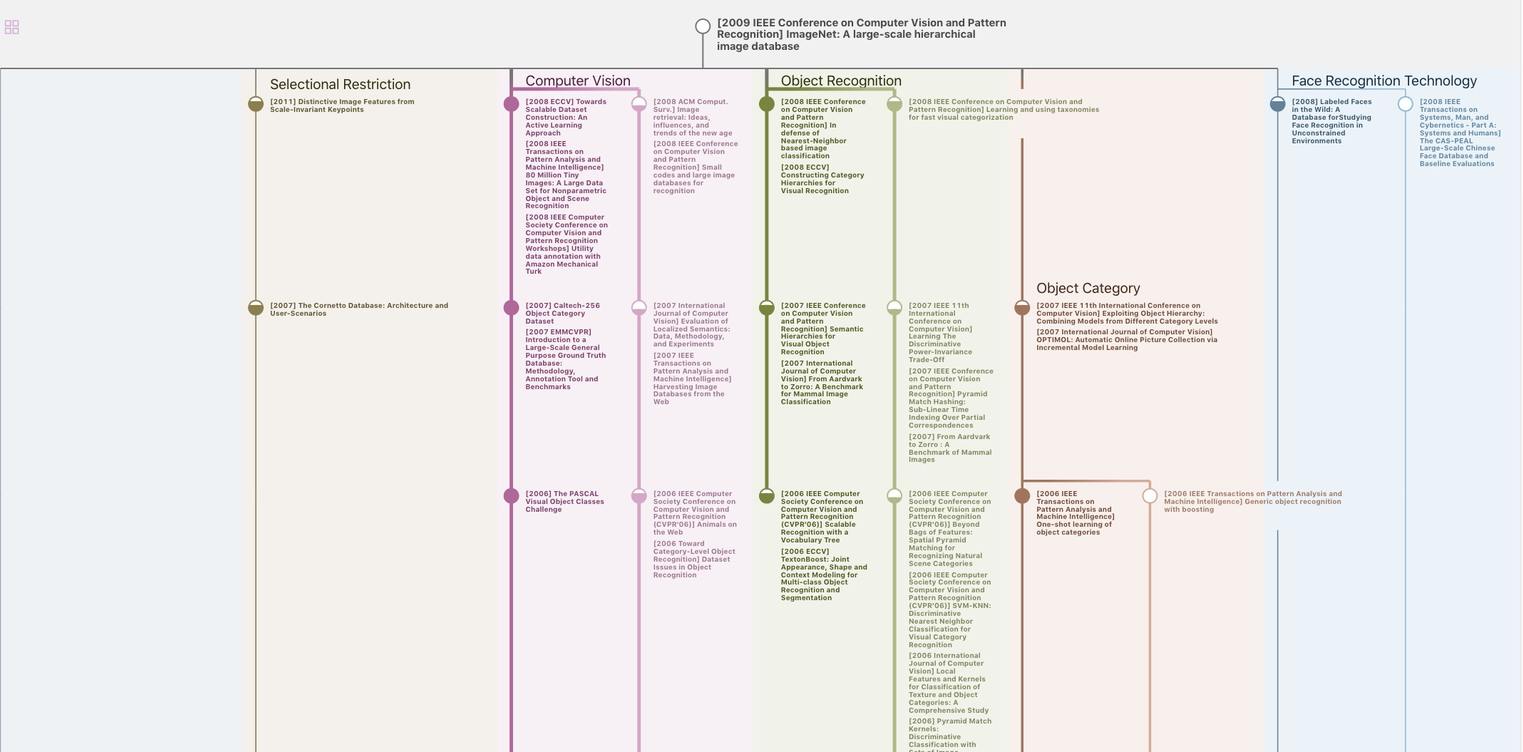
生成溯源树,研究论文发展脉络
Chat Paper
正在生成论文摘要