Knowledge Accumulation in Continually Learned Representations and the Issue of Feature Forgetting
CoRR(2023)
摘要
Continual learning research has shown that neural networks suffer from
catastrophic forgetting "at the output level", but it is debated whether this
is also the case at the level of learned representations. Multiple recent
studies ascribe representations a certain level of innate robustness against
forgetting - that they only forget minimally and no critical information. We
revisit and expand upon the experiments that revealed this difference in
forgetting and illustrate the coexistence of two phenomena that affect the
quality of continually learned representations: knowledge accumulation and
feature forgetting. Carefully taking both aspects into account, we show that,
even though it is true that feature forgetting can be small in absolute terms,
newly learned information tends to be forgotten just as catastrophically at the
level of the representation as it is at the output level. Next we show that
this feature forgetting is problematic as it substantially slows down knowledge
accumulation. Finally, we study how feature forgetting and knowledge
accumulation are affected by different types of continual learning methods.
更多查看译文
关键词
continually learned representations,knowledge
AI 理解论文
溯源树
样例
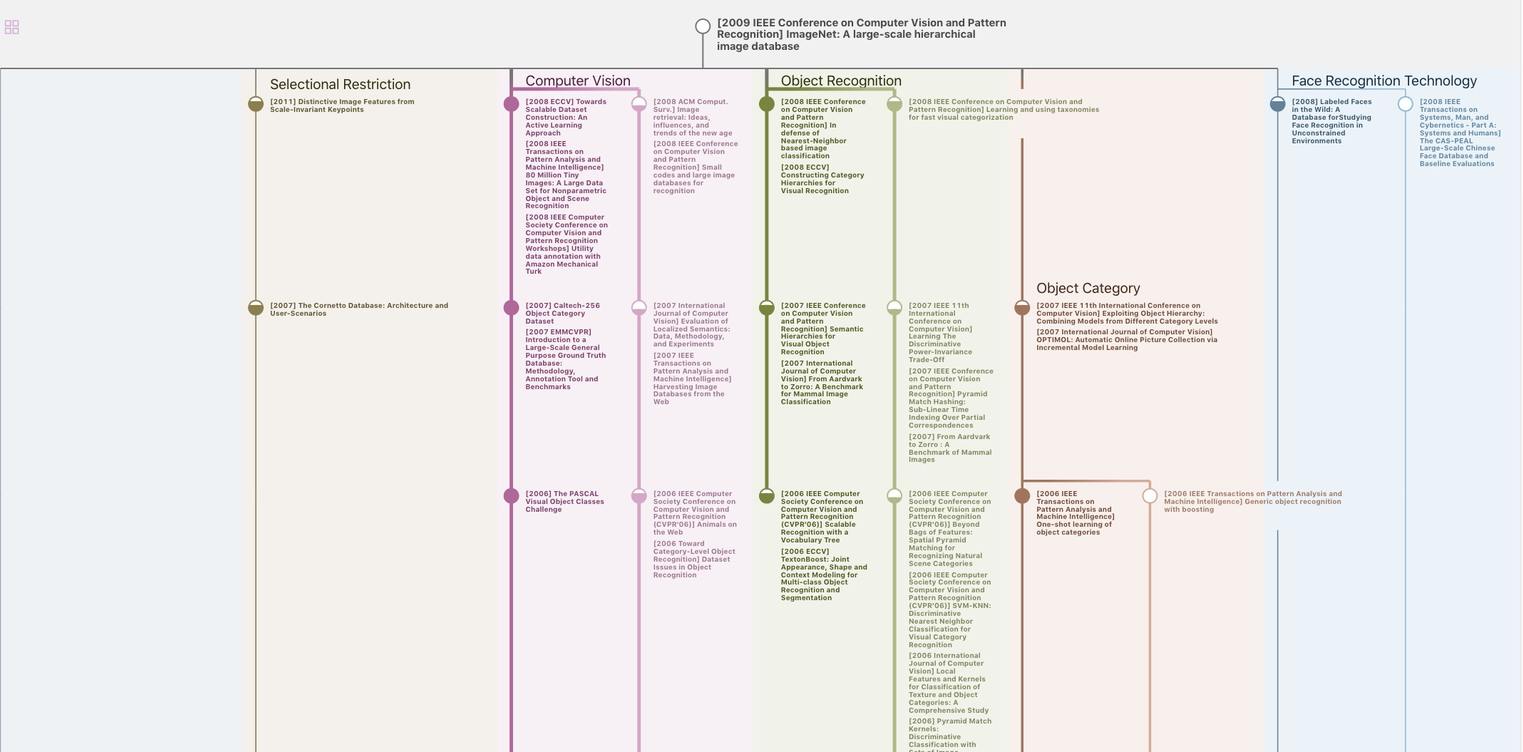
生成溯源树,研究论文发展脉络
Chat Paper
正在生成论文摘要