Fast Convergence of Random Reshuffling under Over-Parameterization and the Polyak-Łojasiewicz Condition.
CoRR(2023)
摘要
Modern machine learning models are often over-parameterized and as a result they can interpolate the training data. Under such a scenario, we study the convergence properties of a sampling-without-replacement variant of stochastic gradient descent (SGD) known as random reshuffling (RR). Unlike SGD that samples data with replacement at every iteration, RR chooses a random permutation of data at the beginning of each epoch and each iteration chooses the next sample from the permutation. For under-parameterized models, it has been shown RR can converge faster than SGD under certain assumptions. However, previous works do not show that RR outperforms SGD in over-parameterized settings except in some highly-restrictive scenarios. For the class of Polyak-Łojasiewicz (PL) functions, we show that RR can outperform SGD in over-parameterized settings when either one of the following holds: (i) the number of samples ( n ) is less than the product of the condition number ( κ ) and the parameter ( α ) of a weak growth condition (WGC), or (ii) n is less than the parameter ( ρ ) of a strong growth condition (SGC).
更多查看译文
关键词
random reshuffling,fast convergence,over-parameterization
AI 理解论文
溯源树
样例
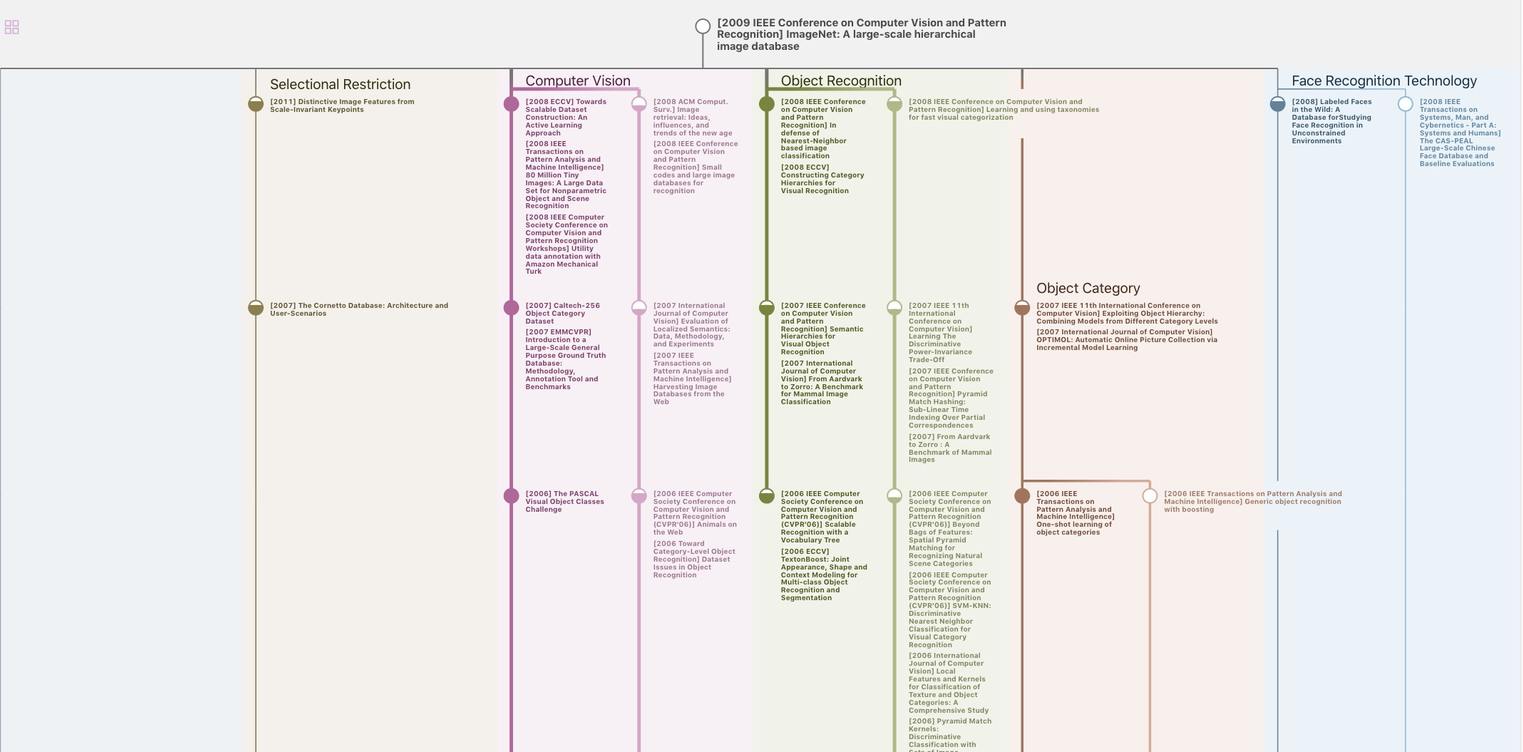
生成溯源树,研究论文发展脉络
Chat Paper
正在生成论文摘要